The Race Toward FTQC: Ocelot, Majorana, Willow, Heron, Zuchongzhi
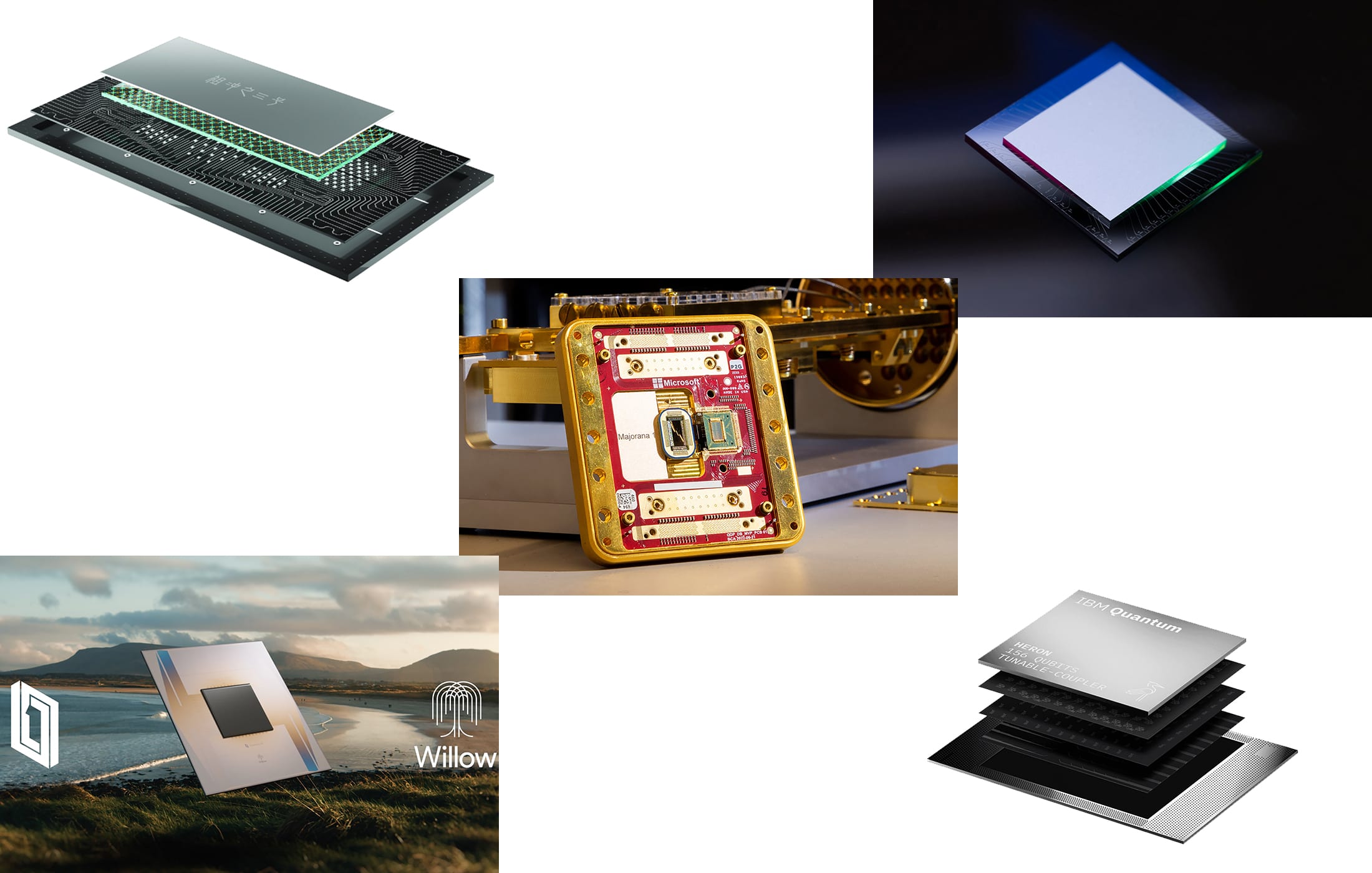
Table of Contents
Introduction
Quantum computing is entering a new phase marked by five major announcements from five quantum powerhouses—Zuchongzhi, Amazon Web Services (AWS), Microsoft, Google, and IBM—all in the last 4 months. Are these just hype-fueled announcements, or do they mark real progress toward useful, large-scale, fault-tolerant quantum computing—and perhaps signal an accelerated timeline for “Q-Day”? Personally, I’m bullish about these announcements. Each of these reveals a different and interesting strategy for tackling the field’s biggest challenge: quantum error correction. The combined innovation pushes the field forward in a big way. But let’s dig into some details:
- Yesterday, China’s quantum computing powerhouse, the Zuchongzhi research teams, unveiled Zuchongzhi 3.0, a new superconducting quantum processor with 105 qubits.
- Few days ago, AWS unveiled “Ocelot,” a prototype quantum chip built around bosonic cat qubits with error correction in its very design.
- Only a few days earlier Microsoft introduced “Majorana 1,” (to massive media hype, I must say) claiming the first quantum processor using topological qubits that promise inherent stability against noise.
- Last December, Google announced their “Willow” chip which pushes superconducting transmon qubits to unprecedented fidelity, demonstrating for the first time that adding qubits can reduce error rates exponentially (a key threshold for fault tolerance).
- And in November last year IBM announced upgraded “Heron R2” quantum chip which similarly advances superconducting qubit architecture with more qubits, tunable couplers, and mitigation of noise sources, enabling circuits with thousands of operations to run reliably.
These developments are significant because they directly address the roadblock of quantum errors that has limited the progress of quantum computers. And they do it in different ways. By improving qubit stability and error correction, they are collectively pushing the industry closer to practical, large-scale, fault-tolerant quantum computing. In the race toward a useful quantum computer, progress is measured not just in qubit count, but in overcoming noise and scaling issues – and that is exactly what these announcements target.
Equally important, these breakthroughs illustrate diverse and complementary approaches. AWS’s bosonic qubits aim to reduce the overhead of error correction by encoding information in oscillator states. Microsoft’s topological qubits seek to harness exotic states of matter to intrinsically protect quantum information at the hardware level. Google and IBM, while both sticking with superconducting circuits, are dramatically improving coherence and using clever engineering (surface codes in Google’s case, and tunable couplers and software optimizations in IBM’s) to inch toward fault-tolerant operation. Zuchongzhi is at the same time time demonstrating the improvements that can be achieved by better noise reduction in the circuit design and better qubit packaging.
Each development is critical in its own way. Together, they represent a concerted push toward the long-sought goal of a practical quantum computer – one that can maintain quantum coherence long enough, and at a large enough scale, to solve real problems beyond the reach of classical machines.
Breakdown of Each Announcement
AWS Ocelot: Bosonic Cat Qubits and Built-In Error Correction
AWS’s announcement of the Ocelot quantum processor marks a paradigm shift in hardware design by making quantum error correction a primary feature of the chip, rather than an afterthought. Ocelot is a prototype consisting of two stacked silicon dies (each ~1 cm²) with superconducting circuits fabricated on their surfaces. It integrates 14 core components: five bosonic “cat” data qubits, five nonlinear buffer circuits to stabilize those cat states, and four ancillary qubits to detect errors. The term cat qubit refers to a qubit encoded in a quantum superposition of two coherent states of a harmonic oscillator (analogous to Schrödinger’s famous alive/dead cat thought experiment). Each cat qubit is realized in a high-quality microwave resonator (oscillator) made from superconducting tantalum, engineered to have extremely long-lived states. The key advantage is that these qubits exhibit a strong noise bias: bit-flip errors (i.e. flips between the two oscillator coherent states) are exponentially suppressed by increasing the photon number in the oscillator. In fact, AWS reports bit-flip error times approaching one second – over 1,000× longer than a normal superconducting qubit’s lifetime. This leaves the primary remaining error mode as phase-flips (relative phase errors between the cat basis states), which occur on the order of tens of microseconds. By dramatically reducing one type of error at the physical level, Ocelot can focus resources on correcting the other.
To catch and correct phase-flip errors, Ocelot uses a simple repetition code across the five cat qubits. The cat qubits are arranged in a linear array and entangled via specially tuned CNOT gates with the four transmon ancilla qubits, which act as syndrome detectors for phase errors. In essence, a phase-flip on any one cat qubit is detected through parity-check measurements (enabled by those ancillas), and the information is redundantly encoded so that a single phase error can be identified and corrected (much like a classical repetition code would correct a bit flip). Meanwhile, each cat qubit’s attached buffer circuit and the noise-biased design of the CNOT gates ensure that the process of error detection doesn’t introduce too many bit-flip errors in return. This concatenation of a bosonic code (for reducing bit-flips) with a simple classical code (for correcting phase-flips) is what AWS calls a hardware-efficient error correction architecture. Notably, the entire logical qubit (distance-5 repetition code) in Ocelot uses only 5 data qubits + 4 ancillas = 9 physical qubits in total, compared to 49 physical qubits that a standard distance-5 surface code would require. AWS’s Nature paper reports that moving from a shorter code (distance 3) to the full distance-5 code significantly lowered the logical error rate (especially for phase flips) without being undermined by additional bit-flip errors. In fact, the total logical error per cycle was roughly 1.65% for the 5-qubit code, essentially the same as the ~1.72% for the 3-qubit code. This demonstrates that Ocelot maintained a large bias in favor of phase errors – the added redundancy suppressed phase flips faster than any new bit-flip opportunities could hurt it. In practical terms, Ocelot achieved a fully error-corrected logical memory that spans five physical qubits, with a net error rate far lower than any individual qubit.
While Ocelot is only a single logical qubit prototype, its specifications are impressive. The cat qubits’ Tbit-flip (bit-flip lifetime) is ~1 s and Tphase-flip ~20 µs. By comparison, a typical transmon qubit might have T1 and T2 in the 0.02–0.1 ms (20–100 µs) range. Thus, Ocelot’s qubits are orders of magnitude more robust against bit-flips. The trade-off is that phase errors remain frequent, but those are exactly what the repetition code handles.
One potential scaling challenge for this approach will be implementing logical gates between multiple cat-qubit logical units – so far Ocelot demonstrates a memory qubit (it stores a quantum state with improved fidelity), but not a logic gate between two logical qubits. Extending the scheme to a fully programmable computer will require linking many such encoded qubits and orchestrating complex syndrome measurements, all while preserving the delicate noise bias. This will demand further integration (more resonators, couplers, and readout circuitry) and likely new techniques to manage higher photon-number states across many modes. Additionally, while repetition codes are simple, more powerful error-correcting codes (with higher distance) might be needed for logic operations, which could increase overhead. AWS, however, is optimistic – they note that if this bosonic approach scales, a full fault-tolerant quantum computer might need only one-tenth the number of physical qubits that conventional architectures would require. Ocelot’s success is a proof-of-concept that bosonic qubits can be integrated into a chip and outperform equivalent transmon-based logical qubits, potentially accelerating the timeline to a useful quantum computer by several years.
For more information see: AWS Pounces on Quantum Stage with Ocelot Chip for Ultra-Reliable Qubits.
Microsoft’s Majorana 1: Topological Qubits and the Quest for Stable Qubits
Microsoft’s Majorana 1 chip represents a long-awaited breakthrough in topological quantum computing. It is the first prototype quantum processor based on Majorana zero modes (MZMs) – exotic quasiparticles that emerge at the ends of specially engineered nanowires and that behave as quasi-particles which are their own antiparticles. In theory, pairs of these MZMs can encode quantum information in a non-local way that is inherently protected from many forms of noise. The Majorana 1 chip is a palm-sized device housing eight topological qubits on a single chip, fabricated with a new materials stack of indium arsenide (InAs) semiconductor and aluminum superconductor. These materials form what Microsoft calls “topoconductors,” creating a topological superconducting state when cooled and placed in a magnetic field. In this state, each tiny nanowire (on the order of 100 µm in length) can host a pair of Majorana zero modes at its ends. Four MZMs (for example, the ends of two nanowires) together encode one qubit’s state in a distributed manner – essentially storing quantum information in the parity of electrons shared across the wire ends, rather than at any single location. This topological encoding is expected to be highly resistant to local disturbances: an error would require a global change that alters the topology (e.g. breaking the pair or moving a Majorana from one end to the other), which is energetically or statistically very unlikely. As a result, a qubit encoded in MZMs should remain coherent far longer than a conventional qubit, without active error correction – at least for certain types of errors (notably, bit-flips in the topologically protected basis).
In announcing Majorana 1, Microsoft revealed that after nearly two decades of research (!), they finally achieved the creation and detection of Majorana zero modes in a device that allows qubit operations. The chip’s eight qubits are arranged in a way that is designed to be scalable to millions of qubits on one chip. Each qubit is extremely small (about 1/100th of a millimeter, or ~10 µm, in size) and fast to manipulate via electronic controls. One of the headline achievements in the accompanying Nature publication was a demonstration of a single-shot interferometric parity measurement of the Majorana modes. In simpler terms, they can read out the joint state of a pair of MZMs (which reveals the qubit’s value) in one go, without needing to average over many trials. This is crucial for using these as qubits. The Nature paper’s peer-reviewed findings confirm that Microsoft created the conditions for Majorana modes and measured their quantum information reliably. However, it’s worth noting an important caveat: while Microsoft has announced the creation of a topological qubit, the Nature reviewers included a comment that the results “do not represent evidence for the presence of Majorana zero modes” themselves, but rather demonstrate a device architecture that could host them. In other words, the scientific community is cautious – they want more definitive proof that the observed behavior is truly due to MZMs. For more on this controversy and other past controversies with Microsoft Majorana team, see my article: Microsoft’s Majorana-Based Quantum Chip – Beyond the Hype.
From a theoretical perspective, a stable topological qubit is like the holy grail of quantum hardware. Its implications are profound. Firstly, stability by design could drastically reduce the overhead needed for error correction – you might not need to constantly perform syndrome measurements or have dozens of physical qubits guarding one logical bit, if the physical qubit is already extremely immune to noise. Microsoft envisions scaling Majorana qubits such that a single chip can host on the order of 1,000,000 qubits. They argue that only with such massive scaling (enabled by the small size and digital controllability of topological qubits) will quantum computers reach the complexity for transformative applications. A million topological qubits, if each is much more reliable than today’s qubits, could theoretically perform the trillions of operations needed for useful algorithms like breaking down complex molecules or factoring large numbers.
It’s sobering, however, that currently Majorana 1 has just eight qubits, and even those have not yet been shown performing arbitrary quantum logic gates – the announcement focused on initialization and measurement (parity control) of the qubits. The next steps will likely involve demonstrating qubit operations like braiding (exchanging Majoranas to perform logic gates) and two-qubit interactions, and showing that these operations obey the expected topological properties (e.g. certain gates being inherently fault-tolerant). If any of these pieces falter – for instance, if environmental factors like quasiparticle poisoning disturb the MZMs too often – additional error correction would still be needed on top of the topological protection. Microsoft did acknowledge that not all gates are topologically protected; for example, the so-called T-gate (a non-Clifford operation) would still be “noisy” and require supplemental techniques. In summary, Majorana 1 is a daring bet on a fundamentally different approach to quantum computing. After years of setbacks and skepticism, Microsoft’s latest results have started to convince the community that topological qubits might finally be real. If the claim stands, it’s a watershed moment: a new state of matter (topological superconductor) harnessed to create qubits that are naturally resilient. That could eventually translate to quantum processors with vastly higher effective performance, as error correction overhead is slashed. In the near term, Majorana 1 will be used internally for further research – it’s not yet solving any useful problems – but it lays a theoretical foundation that could leapfrog other technologies if it scales as hoped.
For more information see: Microsoft’s Majorana-Based Quantum Chip – Beyond the Hype.
Google Willow: A 105-Qubit Transmon Processor Achieving Error-Correction Thresholds
Google’s Willow quantum chip is the latest in the line of superconducting processors from the Google Quantum AI team, and it comes with two major achievements:
- it significantly boosts coherence and fidelity such that adding more qubits actually decreases the overall error rate (crossing the coveted error-correction threshold), and
- it demonstrated an ultra-high-complexity computation in minutes that would take a classical supercomputer an astronomically long time.
Willow contains 105 superconducting qubits of the transmon variety, arranged in a 2D lattice suitable for the surface code error-correcting scheme. The qubits are connected by couplers in a layout similar to Google’s previous 54-qubit Sycamore processor, but with notable architectural improvements. One key upgrade is that Willow retains the frequency tunability of qubits/couplers (as Sycamore had) for flexible interactions, while dramatically improving coherence times: the average qubit energy-relaxation time T1 on Willow is about 68 µs, compared to ~20 µs on Sycamore. This ~3× improvement in coherence is partly due to better materials and fabrication (Google cites a new qubit design and mitigation of noise sources) and partly due to improved calibration techniques (leveraging machine learning and more efficient control electronics). In tandem, two-qubit gate fidelities were roughly doubled compared to the Sycamore generation. If Sycamore’s CZ gates had error rates on the order of 0.6%, Willow’s are around ~0.3% or better (single-qubit gates are even higher fidelity). These numbers put Willow in the regime of the best superconducting qubits reported in any lab to date.
Crucially, this hardware boost allowed Google to demonstrate scalable quantum error correction for the first time. Using the surface code (a topological quantum error-correcting code on a 2D grid of qubits), the team encoded a logical qubit into increasing sizes of code: a 3×3 patch of qubits (distance-3 code), a 5×5 patch (distance 5), and a 7×7 patch (distance 7). With each increase in code distance, they observed an exponential suppression of error rates – specifically, each step up reduced the logical error rate by about a factor of 2 (a 7×7 code’s logical error was ~4× lower than that of a 3×3 code). By the largest code (49 physical qubits encoding 1 logical qubit), the logical qubit’s lifetime exceeded that of the best individual physical qubit on the chip. This means the logical qubit is actually higher quality than any bare physical qubit – a landmark known as “beyond break-even” quantum error correction. In the language of error correction, Google had crossed the fault-tolerance threshold: their operations are in a regime “below threshold” where adding more qubits to the code yields net fewer errors. This is the first time a superconducting quantum system has definitively shown such behavior in real time (previous attempts either saw no improvement with code size or only marginal improvement). For more in-depth information about the Google’s error correction achievement, see the Nature paper accompanying the announcement: Quantum error correction below the surface code threshold. Achieving this required not only excellent qubits, but also real-time decoding and feedback. Google implemented fast error syndrome extraction cycles and decoding algorithms (with help from classical compute and custom ML algorithms) that can identify and correct errors on the fly, faster than they accumulate. In the Nature article published alongside Willow, they report that with the 7×7 code, the logical error probability per cycle was cut in half compared to the 5×5 code, firmly establishing that they are operating in the scalable regime. In summary, Google Willow is the first platform where quantum error correction “works” in practice – reaching the point where bigger truly means better in terms of qubit arrays.
Another headline from Google’s announcement was a demonstration of raw computational power. Willow executed a random circuit sampling benchmark of unprecedented size, completing it in about 4.5 minutes. Google claims that the Frontier supercomputer (currently the world’s fastest, at ~1.35 exaflops) would take on the order of 1025 years to perform the equivalent task. This massive separation (quantum vs classical) far exceeds the 2019 “quantum supremacy” demonstration, where the task was estimated to take 10,000 years on an IBM supercomputer. In fact, after optimizations, that 2019 task was brought down to a matter of days on classical machines, but Google notes that for this new experiment, even accounting for future classical improvements, the quantum speedup is growing “at a double exponential rate” as circuit size increases. The benchmark involved entangling all 105 qubits in a complex pattern and performing many layers of random two-qubit gates, a test that is both computationally hard for classical simulation and pushes the quantum chip to its limits. The ability to run such a large circuit (5000+ two-qubit gate operations in total) was enabled by Willow’s lower error rates and the error correction capability – indeed, IBM had run a similarly large circuit (2880 two-qubit gates) in 2023 on their 127-qubit Eagle, but required heavy error mitigation to get a valid result. Google’s milestone indicates that quantum supremacy has been re-affirmed on a larger scale, and now with a machine that is closer to being error-corrected. It is a proof that increased qubit count plus error reduction can yield computational results vastly beyond classical reach, reinforcing confidence that scaling up will unlock useful quantum advantage.
From an architectural standpoint, Willow doesn’t introduce radical new qubit types – it’s still a transmon chip – but it showcases incremental advances coalescing into a big leap. The coherence improvements (68 µs T1, ~50–70 µs T2) came from material upgrades like better substrate and surface treatment and possibly using indium bump-bonds (though unconfirmed) to reduce loss. The tunable couplers and qubits allow flexibility in isolating qubits when idle and reducing crosstalk (a technique IBM also employs in its chips), which contributes to lower error rates. Additionally, Google employed advanced control software: automated calibrations, machine learning for fine-tuning pulses, and a reinforcement learning agent to optimize error correction performance. The integration of all these pieces is what allowed Willow to hit the below-threshold regime. One challenge ahead for Google is how to continue scaling qubit count while keeping errors down. Their roadmap, like others, will require modularity – possibly linking multiple 100+ qubit chips or developing larger wafers – since simply doubling qubits on one die could re-introduce noise and fabrication difficulties. But the surface-code approach has now been validated: if each module can have, say, 1000 qubits at a few 0.1% error rate, one can start assembling logical qubits of very high quality by using enough physical qubits. Google’s achievement with Willow gives a clear quantitative target: a logical qubit with error ~10-3 (0.1%) per operation was achieved with 49 qubits; pushing that error down further to, say, 10-6 will require perhaps a few hundred physical qubits per logical qubit. Willow is the stepping stone demonstrating that the scaling curve holds as expected.
In short, Google’s Willow announcement is a strong validation of the transmon/surface-code path to fault tolerance: it showed that with improved hardware and clever coding, one can now suppress errors exponentially with system size. This moves the field closer to practical quantum error correction, and along with it, closer to running useful algorithms reliably on a quantum machine.
For more information see my summary of the announcement: Google Announces Willow Quantum Chip.
IBM Heron R2: Tunable-Coupler Architecture and Enhanced Quantum Volume
IBM’s announcement of the Heron R2 processor is an evolution of their superconducting quantum hardware focused on scaling up qubit count while maintaining high performance. Heron R2 contains 156 qubits arranged in IBM’s signature heavy-hexagonal lattice topology. This is a qubit connectivity graph where each qubit connects to at most 3 neighbors in a hexagonal pattern with missing connections, which IBM uses to reduce crosstalk and enable efficient error-correcting codes. The Heron family is notable for introducing tunable couplers between every pair of connected qubits, a feature first seen in Heron R1 (a 133-qubit chip debuted in late 2023). In R2, IBM increased the qubit count to 156 by extending the lattice and incorporating lessons from their 433-qubit Osprey system’s signal delivery improvements. The tunable coupler design allows two qubits’ interaction to be turned on or off (and adjusted in strength) dynamically, which greatly suppresses unwanted coupling and frequency collisions when multiple operations are happening in parallel. This effectively eliminates a lot of “cross-talk” errors that plague fixed-coupling architectures. According to IBM, Heron demonstrated a 3–5× improvement in device performance metrics compared to their previous generation (the 127-qubit Eagle) while “virtually eliminating” cross-talk. Specific numbers from Heron r1 (133 qubits) showed a quantum volume (QV) of 512 – a measure combining number of qubits and gate fidelity – which was a new high for IBM at that time. Heron R2 likely pushes that even further.
The Heron R2 chip also introduced a new “two-level system” (TLS) mitigation technique in hardware. TLS defects in the materials (like microscopic two-level fluctuators at surfaces or interfaces) are a known cause of qubit decoherence and sporadic errors. IBM built circuitry or calibration procedures into Heron R2 to detect and mitigate the impact of TLS noise on the qubits. The result is improved stability of qubit frequencies and, by extension, better gate fidelity and coherence times. While IBM hasn’t publicly quoted average T1/T2 for Heron R2, their emphasis on TLS mitigation suggests each qubit’s coherence is more consistently near the upper limits (potentially several hundred microseconds). They also improved the readout and reset processes (IBM has been developing fast, high-fidelity qubit readout and qubit reuse via reset to speed up circuits). In terms of integration with software, Heron R2 is delivered via IBM’s Quantum Cloud and is fully compatible with Qiskit runtime improvements. In fact, IBM highlighted that by combining the Heron R2 hardware with software advances like dynamic circuits and parametric compilation, they achieved a sustained performance of 150,000 circuit layer operations per second (CLOPS) on this system. This is a dramatic increase in circuit execution speed – by comparison, in 2022 their systems ran ~1k CLOPS, and by early 2024 around 37k CLOPS. Faster CLOPS means researchers can execute deeper and more complex algorithms within the qubits’ coherence time or gather more statistics in less wall-clock time.
The most concrete evidence of Heron R2’s advancement was IBM’s announcement that it can reliably run quantum circuits with up to 5,000 two-qubit gates. This is nearly double the 2,880 two-qubit gates used in IBM’s 2023 “quantum utility” experiment on the Eagle chip. In that experiment (published in Nature), IBM showed that a complex many-qubit circuit could be executed with enough fidelity – using error mitigation – to get a meaningful result beyond the reach of brute-force classical simulation. Now, with Heron R2, circuits almost twice as long can be run accurately without custom hardware tweaks, using the standard Qiskit toolchain. In other words, Heron R2 pushed IBM’s quantum processors firmly into the “utility scale” regime, where they can explore algorithms that are not toy models. Importantly, this 5,000-gate capability was achieved by both hardware improvements (lower error rates per gate) and software error mitigation. IBM mentions a “tensor error mitigation (TEM) algorithm” in Qiskit that was applied. TEM is a method to reduce errors in circuit outputs via classical post-processing and knowledge of the noise, which IBM integrated into its runtime. So Heron R2, paired with such techniques, can execute long circuits 50× faster than was possible a year before, and with enough accuracy that the outputs are trustworthy.
In terms of raw metrics: IBM’s median two-qubit gate error on Heron R2 is not explicitly stated in the announcement, but given the performance, it is likely on the order of ~0.5% or better, with some qubits achieving 99.5–99.7% fidelity. Single-qubit gates are usually above 99.9%. The heavy-hex topology is slightly less connectivity than a full grid, but it has an advantage for the surface code (which IBM uses in some experiments) because it naturally forms a planar grid of data and measure qubits when laid out appropriately. IBM has been testing small distance surface codes on previous chips and will presumably do so on Heron as well. However, IBM’s near-term strategy has also emphasized error mitigation and “quantum utility” over full error correction, meaning they try to find ways to get useful results from the hardware at hand by combining it with classical processing. Heron R2 is a continuation of that philosophy: improve the hardware just enough to push the envelope of what can be done in the NISQ era, while laying groundwork for truly fault-tolerant hardware in the future. The Heron architecture (with tunable couplers) is in fact the template for IBM’s upcoming larger systems and modular systems. IBM plans to connect multiple Heron chips via flexible interconnects and a special coupler (codenamed Flamingo) to scale to larger effective processors. They already demonstrated a prototype of this modular approach, showing that two Heron chips could be linked with an entangling gate across a ~meter distance with only minor loss. So Heron R2 is not just a stand-alone 156-qubit device, but also a module in IBM’s System Two quantum computer architecture, which envisions combining modules to reach thousands of qubits. In summary, IBM’s Heron R2 announcement is about refinement and integration: more qubits, better noise control (tunable couplers + TLS mitigation), and faster software all coming together. The result is a quantum processor that significantly extends IBM’s ability to run complex algorithms (approaching the 100×100 qubit-depth challenge they posed). While it may not boast a fundamentally new qubit type or a dramatic physics breakthrough, it is a critical incremental step. It shows that IBM can scale up without sacrificing performance, which is essential on the march toward a fault-tolerant machine.
Zuchongzhi 3.0: China’s Breakthrough in Superconducting Quantum Hardware
Chinese researchers have officially unveiled Zuchongzhi 3.0, a 105-qubit superconducting quantum processor that sets a new benchmark in computational speed and scale. In its debut demonstration, the chip executed an 83-qubit random circuit sampling task (32 layers deep) in only a few minutes, producing results that would take a state-of-the-art classical supercomputer on the order of 6.4 billion years to simulate. This represents an estimated 1015-fold speedup over classical computation and roughly a one-million-fold (6 orders of magnitude) improvement over Google’s previous Sycamore experiment. The feat, published as a cover article in Physical Review Letters, reinforces China’s advancement in the race for quantum computational advantage, marking the strongest quantum advantage achieved to date on a superconducting platform.
Zuchongzhi 3.0 features significant upgrades from its 66-qubit predecessor. The new processor integrates 105 transmon qubits in a two-dimensional grid with 182 couplers (interconnections) to enable more complex entanglement patterns. It boasts a longer average coherence time of about 72 µs and high-fidelity operations (approximately 99.9% for single-qubit gates and 99.6% for two-qubit gates) – achievements made possible by engineering improvements such as noise reduction in the circuit design and better qubit packaging. These technical innovations allow Zuchongzhi 3.0 to run deeper quantum circuits than earlier chips and even support initial quantum error-correction experiments. The team has demonstrated surface-code memory elements (distance-7 code) on this chip and is working to push to higher error-correction thresholds, highlighting the new capabilities enabled by the processor’s improved stability and scale.
Expanded Technical Comparison
Each of these five quantum computing approaches brings something unique to the table. In this section, I’ll try and compare their technical metrics and how they contribute to the overarching goal of fault-tolerant quantum computation.
Qubit Type and Architecture
The five quantum processors employ three distinct qubit technologies.
AWS Ocelot
AWS Ocelot uses superconducting cat qubits, where each qubit is encoded in two coherent states of a microwave resonator. This design intrinsically suppresses certain errors by biasing them (bit-flip errors are strongly suppressed). Ocelot’s chip integrates 14 core components: 5 cat-qubit resonators serving as data qubits, 5 buffer circuits to stabilize these oscillator qubits, and 4 superconducting ancilla qubits to detect errors on the data qubits. Notably, it’s a hardware-efficient logical qubit architecture: only 9 physical qubits yield one protected logical qubit thanks to the cat qubit’s built-in error bias. The chip is manufactured with standard microelectronics processes (using tantalum on silicon resonators) for scalability. In essence, AWS has taken a transmon-based circuit and augmented it with bosonic oscillator qubits to realize a bias-preserving quantum memory.
Microsoft Majorana-1
In contrast, Microsoft Majorana-1 uses an entirely different approach: topological qubits based on Majorana zero modes (MZMs). These qubits are realized in a special “topological superconductor” formed in indium arsenide/aluminum nanowires at cryogenic temperatures. Each qubit (a so-called tetron) consists of a pair of nanowires hosting four MZMs in total (two MZMs per wire, at the ends). Quantum information is stored non-locally in the parity of electron occupation across two MZMs, which makes it inherently protected from local noise. The Majorana qubits are manipulated through braiding operations or equivalent measurement-based schemes, rather than the gate pulses used for transmons. Majorana-1 is an 8-qubit prototype (meaning it can host 8 topological qubits) implemented as a 2D array of these nanowire-based devices. It’s the first processor to demonstrate this Topological Core architecture, which Microsoft claims can be scaled to millions of qubits on a chip if the approach proves out. The challenge of reading out a topological qubit’s state (since the information is “hidden” in a parity) is solved by a novel measurement mechanism: coupling the ends of the nanowire to a small quantum dot and probing with microwaves. The reflection of the microwave signal changes depending on whether the qubit’s parity is even or odd, allowing single-shot readout of the Majorana qubit’s state.
The remaining three chips – Google Willow, IBM Heron R2, and Zuchongzhi 3.0 – all use superconducting transmon qubits, but with different circuit architectures.
Google Willow
Google’s Willow is a 105-qubit superconducting processor that builds on Google’s prior Sycamore design. The qubits are laid out in a 2D planar grid with tunable couplers or fixed capacitive couplings forming a near-nearest-neighbor topology. Willow’s lattice is effectively a dense rectangular grid (15×7 array) similar to a heavy-square lattice, with an average coordination of ~3.5 couplings per qubit. (Each qubit interacts with 3 or 4 neighbors, facilitating two-qubit gates in parallel.) Google optimized Willow’s design for both high connectivity and low cross-talk – for example, by using an iSWAP-like two-qubit gate that can be applied on many pairs simultaneously without excessive interference. The Willow chip is fabricated in a multi-layer process with superconducting aluminum circuits on silicon, and includes integrated microwave resonators for readout of each qubit.
IBM Heron R2
IBM Heron R2 is also a superconducting transmon processor, but IBM employs its signature heavy-hexagon lattice architecture. Heron R2 contains 156 transmon qubits arranged such that each qubit has at most 3 neighbors (a heavy-hex lattice). This geometry deliberately “prunes” the connectivity relative to a square grid in order to reduce cross-talk and correlated errors. Crucially, Heron uses tunable coupler elements between qubits. These couplers (based on additional Josephson junction circuits) can be activated to mediate two-qubit interactions or deactivated to effectively isolate qubits when no gate is intended. This tunable coupling architecture dramatically suppresses unwanted interactions and cross-talk during idle periods. The first revision Heron R1 had 133 qubits; the revised Heron R2 expanded to 156 qubits in the same architecture. Each qubit is coupled to a dedicated resonator for state readout, and the chip features advanced signal delivery (high-density flex wiring and packaging) to control so many qubits in parallel. IBM’s design emphasizes modularity: 156-qubit Heron chips are the building blocks for larger systems, and multiple Heron chips can be connected via microwave links in IBM’s Quantum System Two for scaling beyond a single die.
USTC Zuchongzhi 3.0
Zuchongzhi 3.0, developed by USTC in China, likewise consists of superconducting transmon qubits in a planar array. It has 105 qubits laid out in a 2D rectangular lattice (15 rows × 7 columns). Unlike IBM’s heavy-hex, Zuchongzhi uses a relatively high connectivity: each qubit is coupled to up to 4 nearest neighbors (except on edges), similar to Google’s approach. In fact, the device is noted to be quite similar to Google’s Willow in terms of qubit count and connectivity. One distinctive aspect of Zuchongzhi 3.0’s architecture is its flip-chip integration: it is built from two bonded sapphire chips. One chip contains the 105 transmon qubits and 182 coupling circuits (the in-plane couplers between qubits), and a second chip mounts on top containing all the control wiring and readout resonators. This 3D integration separates the dense control interconnects from the qubit plane, reducing interference and allowing a more compact qubit layout. The transmons are implemented using superconducting tantalum/aluminum fabrication (USTC introduced tantalum material to improve quality factors). The use of flip-chip and novel materials in Zuchongzhi 3.0 shows a strong engineering focus on scaling up superconducting qubit count without sacrificing coherence.
Summary of Qubit Types and Architectures
Ocelot and Majorana-1 pursue radical qubit designs (bosonic and topological, respectively) to embed error resilience at the hardware level, whereas Willow, Heron R2, and Zuchongzhi 3.0 refine the well-established transmon approach with clever layout and coupling innovations. The transmon-based chips pack the largest qubit counts (105–156 qubits) and have demonstrated complex circuit benchmarks, while the cat-qubit and Majorana devices, though smaller in qubit number, represent proof-of-concept leaps toward fault-tolerant architectures built on novel physics.
Coherence Times (T1 and T2)
Coherence time is a critical metric for qubit performance, as it determines how long a qubit can retain quantum information. There are two relevant timescales: T1, the energy relaxation time (how long the qubit stays in an excited state before decaying to ground), and T2, the dephasing time (how long superposition phase coherence is maintained). In an ideal two-level qubit, the excited state population decays as $$e^{-t/T1}$$, and off-diagonal elements of the qubit’s density matrix decay as $$e^{-t/T2}$$. Longer T1 and T2 are better, allowing more operations to be performed before errors occur. The five chips show significant differences in coherence, largely stemming from their differing qubit implementations:
AWS Ocelot
The cat qubit architecture achieves an extreme asymmetry in coherence times. By encoding the qubit in a pair of oscillator states, Ocelot’s qubits exhibit a bit-flip error time (T1) exceeding 10 seconds in experimental demonstrations – several orders of magnitude longer than ordinary transmons. In other words, spontaneous transitions between the logical |0⟩ and |1⟩ states (which correspond to two distinct coherent states of the resonator) are extraordinarily rare. This 10+ s T1 for bit-flips is 4–5 orders larger than previous cat qubits and vastly larger than any transmon T1. However, this comes at a cost: the phase-flip coherence (T2) of the cat qubit is much shorter, because the environment can more easily cause dephasing between the two cat basis states. The reported phase-flip time is on the order of $$~5×10^{-7}$$ s (sub-microsecond) in current cat qubit experiments. In essence, Ocelot’s qubits have a highly biased noise: bit-flip processes are suppressed by ~107 relative to phase-flip. The buffer circuits in Ocelot are designed to prolong the phase coherence somewhat, but T2 is still much shorter than T1. This is acceptable since the system will use active error correction to handle phase errors. The key point is that Ocelot’s qubits rarely lose energy (T1 ~ 10 s), but they lose phase coherence relatively quickly, meaning their superpositions need periodic stabilization.
Microsoft Majorana-1
Majorana qubits are expected to be intrinsically long-lived because the qubit states are stored non-locally. In the initial Majorana-1 device, the team reported that external disturbances (e.g. quasiparticle poisoning events that flip the parity) are rare: roughly one parity flip per millisecond on average. We can treat this as an effective T1 on the order of 1 ms for the topological qubit, meaning the probability of a qubit spontaneously changing state is about $$10^{-3}$$ per millisecond. This is already an order of magnitude longer lifetime than typical superconducting qubits. It implies, for example, that during a 1 µs operation, the chance of an environment-induced error is on the order of $$10^{-6}$$, which is very low. As for T2, a topologically encoded qubit should be largely immune to many dephasing mechanisms since local phase perturbations do not change the global parity state. The practical T2 might be limited by residual coupling between the Majorana modes or fluctuations in the device tuning (e.g. magnetic field noise), but quantitative values have not been fully disclosed. The Majorana qubits have demonstrated the ability to maintain quantum superposition without decay over experimental timescales shorter than the 1 ms parity lifetime, indicating T2 on the order of at least hundreds of microseconds in the current device. In essence, Majorana-1 shows millisecond-scale coherence – a significant leap – thanks to topological protection. (The first measurements had ~1% readout error, which is more a readout infidelity than a coherence limit, and the team sees paths to reduce that error further.)
Google Willow
Willow represents a big improvement in coherence over Google’s earlier Sycamore chip. Google reports mean coherence times of T1 ≈ 98 µs and T2 (CPMG) ≈ 89 µs for the qubits on Willow. This is about a 5× increase over Sycamore’s ~20 µs coherence. Such improvement was achieved by materials and design changes (for example, using improved fabrication to reduce two-level-system defects and better shielding to reduce noise). A T1 of 98 µs means an excited qubit loses energy with a time constant of nearly 0.1 ms, and T2 of 89 µs indicates phase coherence is maintained nearly as long. These figures are among the highest reported for large-scale superconducting chips. Crucially, Willow’s coherence is uniform across its 105 qubits – the averages imply most qubits are in that ballpark, which is important for multi-qubit operations. With ~100 µs coherence and gate times on the order of tens of nanoseconds, Willow’s qubits can undergo on the order of $$10^3$$ operations before decohering (in the absence of error correction). This long coherence was key to enabling Willow to run relatively deep circuits and even error-correction experiments successfully. It’s worth noting that Google employed dynamical decoupling and CPMG sequences (hence quoting T2,CPMG) to extend effective T2 to ~89 µs. The true T2 (Ramsey dephasing without echoes) might be lower, but through echo techniques they mitigate inhomogeneous dephasing.
IBM Heron R2
IBM’s Heron family also achieved substantial coherence times, though IBM often emphasizes other metrics (like gate fidelity) over raw T1/T2 in public disclosures. The heavy-hex design and introduction of a TLS mitigation layer in R2 specifically targeted improving coherence across the whole 156-qubit chip. By reducing two-level-system defects and material losses, IBM likely has many qubits with T1 on the order of 100 µs or more. In earlier IBM devices (e.g. 27-qubit Falcon chips), T1 ~ 50–100 µs and T2 ~ 50 µs were typical. Heron R2, being a new revision, likely pushed T1 further. Indeed, one source notes Heron’s two-qubit gate fidelity improvements came partly from better coherence and stability across the chip due to TLS environment control. Without official IBM numbers in this text, we extrapolate: Heron R2’s coherence should be comparable to Willow’s range. IBM’s focus on uniformity means no outlier qubits with very low T1 – they design for a stable floor of performance. It’s reasonable to assume T1,T2 on Heron are in the few × $$10^1$$ µs range (tens of microseconds). IBM has reported individual qubits with T1 > 300 µs in the past, but for Heron’s large array, a safer estimate is T1 ~100 µs, T2 ~100 µs average. This is supported by IBM’s introduction of new filtering and isolation techniques that “improve coherence and stability across the whole chip.” In summary, Heron R2’s transmons have high but perhaps slightly lower coherence than Willow’s best (IBM prioritizes reducing noise and cross-talk in other ways as well). The heavy-hex layout itself helps coherence by minimizing frequency crowding and interference. Thus, IBM’s coherence times are in the same ballpark as Google’s – on the order of $$10^{-4}$$ s – ensuring that hundreds to a thousand operations can be executed per qubit before decoherence if error mitigation is applied.
USTC Zuchongzhi 3.0
The USTC team made coherence enhancement a major goal in Zuchongzhi 3.0, and they achieved a marked improvement over their prior 66-qubit chip. They report an average T1 ≈ 72 µs and T2 (CPMG) ≈ 58 µs across the 105 qubits. These values, while slightly below Google Willow’s, are still very high for a large device (for comparison, Zuchongzhi 2.0 had significantly lower coherence, though exact numbers weren’t given in this snippet). The team credits several engineering strategies for this improvement: adjusting qubit capacitor geometries to reduce surface dielectric loss, improved cryogenic attenuation to cut environmental noise (boosting T2), and using tantalum/aluminum fabrication to get better material quality. They also implemented an indium bump bonding process (flip-chip) which reduced interface contaminants and improved T1 by mitigating Purcell effect and other loss channels. The results speak to a careful balancing act: after adding more qubits and couplers, they still increased average coherence (T1 ~72 µs) relative to the previous generation. However, as the comparison published shows, Zuchongzhi 3.0’s T1/T2, while excellent, are a bit lower than Willow’s (98/89 µs) – likely due to the slightly denser integration or materials differences. Still, with ~60–70 µs coherence, Zuchongzhi’s qubits can handle many operations within their coherence window. The team found that this coherence boost directly translated to lower gate errors (single-qubit and two-qubit error rates dropped accordingly).
Summary of Coherence Times
Majorana-1 and Ocelot offer novel forms of extended coherence: Majorana qubits with ~1 ms parity stability and cat qubits with an astounding ~10 s T1 (but short T2). The transmon-based chips (Willow, Heron R2, Zuchongzhi 3.0) all achieve T1,T2 on the order of $$10^{-5}$$ to $$10^{-4}$$ seconds (tens of microseconds to nearly 0.1 ms), which is state-of-the-art for superconducting qubits at their scale. These coherence times are long enough that, if combined with quantum error correction, qubit errors can be significantly suppressed. From a mathematical perspective, if a gate operation takes $$t_g$$ and a qubit has $$T2$$, the decoherence error per gate is roughly $$1 – \exp(-t_g/T2) ≈ t_g/T2$$ for $$t_g \ll T2$$. For example, Willow’s two-qubit gate time of ~42 ns on a qubit with $$T2≈89 µs$$ yields an intrinsic dephasing error ~$$42\text{ns}/89\text{µs} ≈ 5×10^{-4}$$, consistent with its measured two-qubit error on the order of $$10^{-3}$$ (since control errors and T1 contribute additional small error). Each platform has pushed coherence to a regime sufficient for complex multi-qubit experiments: the superconducting platforms do so with improved materials and design, while the cat and topological platforms do so via fundamentally different qubit encodings that eliminate or postpone certain decay channels.
Error Rates and Error Correction Techniques
Because quantum computations are so sensitive to errors, all these platforms employ strategies to minimize and correct errors at either the physical or logical level. We distinguish physical error rates (errors per gate or per time on individual qubits) from logical error rates (errors in encoded qubits that use many physical qubits with an error-correcting code). A key concept is the fault-tolerance threshold: if physical error rates can be pushed below some threshold (around 1% for many codes like the surface code), then increasing the code size will exponentially suppress the logical error rate. Each of the five chips approaches this challenge differently.
AWS Ocelot
Ocelot attacks the error problem by biasing the qubit noise so that one type of error is extremely rare. In Ocelot’s cat qubits, bit-flip errors (X errors) are essentially eliminated at the hardware level (bit-flip time ~10 s as noted). This means the physical error rate for bit-flips is astronomically low (on the order of $$10^{-8}$$ per second or less). The dominant remaining errors are phase flips (Z errors), which occur with much higher probability (phase coherence time ~0.5 µs). However, phase errors can be detected and corrected by a simpler code since bit-flips don’t occur to compound the problem. Ocelot essentially builds quantum error correction into the qubit architecture from the start. The 5 cat qubits plus 4 ancillas on the chip implement a small quantum error-correcting code that continuously stabilizes the logical qubit. Although AWS hasn’t publicly detailed the exact code, it is likely a repetition code or parity-check code across the cat qubits that detects phase flips. The result is a hardware-efficient logical qubit: AWS achieved one logical qubit from only 9 physical qubits, versus the thousands of physical qubits that a surface code would require to get similar logical stability.
In percentage terms, AWS claims Ocelot’s approach reduces the qubit overhead for error correction by up to 90%. This is a tremendous resource saving. The trade-off is that Ocelot’s current logical qubit is just a single qubit – error correction is used to keep that qubit stable, but the chip doesn’t perform multi-qubit logic yet. Still, demonstrating a logical qubit with 9 physical qubits is an error-correction breakthrough. The logical error rate achieved hasn’t been explicitly stated, but presumably the logical qubit has a dramatically longer lifetime than any single transmon. AWS also implemented the first noise-bias-preserving logic gates on the cat qubits. These are gates designed not to mix X and Z errors (so that a phase error in a cat qubit doesn’t accidentally cause a bit-flip). By “tuning out” certain error channels in the gate operations, they keep the error bias intact even during computations.
In summary, Ocelot’s strategy is prevent most errors, then correct the rest. By combining the cat qubit (which inherently corrects bit-flips) with a small outer code for phase errors, Ocelot’s logical qubit can operate in a regime where logical error per circuit is extremely low – potentially low enough for practical algorithms with far fewer qubits than other approaches would need.
Microsoft Majorana-1
Microsoft’s approach is to make qubits that are almost error-free at the physical level by using topological protection. In theory, a topological qubit in a perfect Majorana device would have zero bit-flip or phase-flip errors (aside from very infrequent non-local errors). In practice, Majorana-1 has shown that many of the usual error mechanisms are absent – for example, the qubit is unaffected by local noise that would disturb a conventional qubit. The only operation that is not topologically protected is the so-called T-gate (a $$\pi/4$$ phase gate), which requires introducing a non-topological resource (magic state injection). This means that while Clifford gates can be done essentially without error by braiding or measurement sequences, the T-gate will have some error that must be corrected via higher-level encoding. The Majorana qubit thus pushes most error correction overhead to a very high level (only needed for handling those T-gates). The measured performance so far: initial readout of the qubit had an error of ~1% per measurement, which can be improved by refining the quantum dot sensor, and qubit parity flips are ~$$10^{-3}$$ per ms as mentioned. If we interpret that in an error-per-operation sense, consider a Majorana qubit undergoing a sequence of operations each ~1 µs long: in 1 µs, the chance of a spontaneous error is ~$$10^{-6}$$ (since 1 µs is 1/1000 of the 1 ms T1). That is an incredibly low physical error probability, far below typical threshold (which is around $$10^{-2}$$).
So Majorana qubits operate firmly in the below-threshold regime at the single-qubit level. Microsoft’s plan is to leverage this by building logical qubits that require far fewer physical qubits. They estimate that a million physical Majorana qubits could yield on the order of a million logical qubits – essentially one physical one per logical, because each is already (almost) a perfect qubit. In practice, some small overhead will be needed for the T-gates: likely they will implement a lightweight error correction or distillation just for those operations. But the overhead is constant, not huge, because all other gates are protected. This is a qualitatively different scenario from, say, superconducting approaches where every operation on every qubit must be error-corrected by redundancy. The phrase used is that Majorana qubits could be “almost error-free” in operation. The Nature paper by Microsoft demonstrated the existence and control of these topological qubits (a big step), and the follow-up roadmap (on arXiv) lays out how to go from the current 8-qubit device to a scalable fault-tolerant machine.
In summary, Microsoft’s error correction philosophy is to build qubits that need far less correction. By achieving physical error rates well below threshold (parity flips ~$$10^{-6}$$ per operation), they sidestep the need for large quantum codes for most operations. They will still need to correct the rare errors (e.g. using repetition codes to catch any parity flips that do occur, and using magic state factories for T-gates), but the resource overhead is vastly smaller. This is why they claim their chip could solve industrial-scale problems “in years, not decades” – because if the physics holds, they won’t have to wait for a million physical qubits just to get 100 logical qubits; they can use those physical qubits directly as useful qubits.
Google Willow
Google’s approach stays within the conventional transmon qubit paradigm but pushes the physical error rates low enough to meet the threshold and then uses standard quantum error correction (QEC) codes. On Willow, the physical gate error rates are impressively low: single-qubit error ≈0.035% and two-qubit error ≈0.14% on average. These error rates (roughly $$3.5×10^{-4}$$ and $$1.4×10^{-3}$$ per gate respectively) are below or on par with the surface code threshold (~1% for a standard surface code, potentially a few tenths of a percent for more realistic noise models). This means Willow’s qubits are good enough that adding redundancy will reduce errors, not amplify them. Google explicitly demonstrated this: they implemented quantum error correction on Willow and on a smaller 72-qubit device, achieving landmark results. In a recent experiment, they realized a distance-5 surface code using 72 qubits and a distance-7 surface code using 105 qubits (the full Willow). The logical error rate of the distance-7 code was significantly lower than that of the distance-5, confirming the threshold condition has been met. In fact, Google reported that their distance-5 logical qubit reached “break-even” – meaning the logical qubit’s error rate was about equal to the best physical qubit’s error rate, and the distance-7 logical qubit lasted twice as long as the best physical qubit on the chip. This is a historic milestone: it’s the first time a logical qubit outperformed the physical components in a solid-state device. Mathematically, in the surface code the logical error $$p_{\text{logical}}$$ should scale approximately as $$p_{\text{logical}} \approx A \left(\frac{p_{\text{phys}}}{p_{\text{thresh}}}\right)^{(d+1)/2}$$ for large code distance $$d$$. Google observed exactly this exponential suppression: as they went from distance 3 to 5 to 7, the logical error dropped in line with an error-per-gate of ~$$0.1%$$ being below threshold. They also ran repetition codes up to distance 29 (using many qubits for a simple linear code) and saw logical error decreasing until very rare correlated error bursts (like cosmic ray hits) set an eventual floor at about one error per hour for the largest code. Those correlated events (which cause simultaneous errors in many qubits) are non-Markovian and weren’t corrected by the code; Google mitigated some by improving chip fabrication (gap engineering to reduce radiation-induced error spikes by 10,000× was mentioned in a commentary). For common error sources, though, Willow’s QEC worked as expected.
The upshot is that Google has demonstrated fault-tolerant operation in a prototype form: they can store quantum info longer with a logical qubit than any single qubit can hold it. The logical error per cycle in their distance-7 code was about $$2.8×10^{-3}$$, roughly half the physical error of the best qubit. As they improve physical qubit fidelity further and scale to larger codes (distance 11, etc.), the logical error will shrink exponentially. Google uses the surface code (a 2D topological code) for these experiments, which requires a 2D array of qubits with nearest-neighbor gates – exactly what Willow provides. They also built a custom high-speed decoder to keep up with the ~MHz-scale cycle time of the code. In summary, Willow’s error correction technique is the traditional one: encode logical qubits in many physical qubits (a distance-7 surface code used 49 physical qubits) and perform syndrome measurements to correct errors in real-time.
What’s important is Willow crossed the threshold: physical two-qubit error 0.14% < 1% means logical errors can be suppressed exponentially. So unlike previous devices that were “too noisy to correct,” Willow’s qubits are good enough to benefit from QEC. This opens the path to scaling – though a lot more qubits will be needed to do something like run a fault-tolerant algorithm, Google has proven the concept on actual hardware.
IBM Heron R2
IBM has also been steadily reducing physical error rates, though at the time of Heron R2’s debut, IBM had not publicly shown a logical qubit beating physical qubits. IBM’s two-qubit gate error on Heron is around 0.3% (99.7% fidelity) on average, and single-qubit errors are on the order of 0.03% (99.97% fidelity). These are comparable to Google’s numbers, albeit slightly higher two-qubit error. This places IBM right around the threshold regime as well. IBM’s strategy for error correction revolves around the surface code in the future, but in the near term they have focused on error mitigation and “quantum utility” using uncorrected qubits. Because Heron R2 can execute circuits with thousands of two-qubit gates reliably (see Benchmarking section), IBM can attempt algorithms with shallow depths or use techniques like zero-noise extrapolation to mitigate errors rather than fully correcting them. That said, IBM’s roadmap explicitly includes scaling up to error-corrected quantum computing. They have been developing the software and classical infrastructure for QEC (e.g., fast decoders, as well as investigating hexagonal lattice variants of the surface code that map well to heavy-hex qubit layout). The heavy-hex lattice is compatible with a rotated surface code, albeit with some boundary adjustments due to degree-3 connectivity. IBM has argued that heavy-hex actually reduces the overhead for the surface code by cutting the number of connections that need to be managed, thereby potentially improving threshold behavior by reducing correlated errors.
The tunable couplers in Heron help suppress cross-talk errors, which means errors are more local and stochastic – an assumption underlying most QEC codes. For example, when qubits are idle, the couplers are off, greatly reducing unintended two-qubit error (which can otherwise create correlated errors that are harder for QEC to handle).
Additionally, IBM introduced “two-level system (TLS) mitigation” in R2, which addresses a specific noise source (spurious defects interacting with qubits). By stabilizing the TLS environment, they reduce fluctuations that could cause multiple qubits to err at once.
These advances are crucial to approach the fault-tolerance threshold with a large chip. While IBM hasn’t announced a qubit-level logical encoding demo like Google did, they have done smaller QEC experiments in the past (e.g., on 5-qubit devices they showed repetition code and small Bacon-Shor code error detection). We can expect IBM to attempt a logical qubit on a bigger system soon. In IBM’s vision, error correction will be integrated with a modular architecture – they talk about concatenated codes or connecting patches of surface code across multiple chips in the future. IBM also often cites the figure that about 1,000 physical qubits per logical qubit might be needed for real applications with surface codes. Heron R2’s error rates (~0.3%) are still a bit above optimum threshold, so IBM is likely aiming to get errors down to ~0.1% or less in next generations (with improved materials, as indicated by their ongoing research). In the meantime, IBM leans on error mitigation: for instance, they use readout error mitigation and probabilistic error cancellation in software to improve effective circuit fidelity without full QEC. This allowed them to do things like successfully execute circuits with 5,000 two-qubit gates and still get meaningful results. Those results are not fully error-corrected, but error-mitigated. The difference is that mitigation doesn’t give exponential suppression of error with size, but it can extend what’s classically simulable or improve accuracy for specific tasks. So one could say IBM is straddling the line – pushing physical qubits as far as possible and using any available error reduction technique, until their hardware is just good enough to justify the jump into full QEC. Given Heron R2’s fidelities, IBM is very close to that line.
In short, IBM’s error rate per gate is approaching the threshold, and their focus is on systematic error reduction (cross-talk, leakage, correlated events) to meet all the criteria for fault-tolerance. As soon as they cross that threshold decisively, they will employ the standard QEC codes (likely a surface code on heavy-hex) to yield logical qubits. Their designs and roadmap have considered this, ensuring the architecture can support fast syndrome measurement cycles and scale logically. IBM also often mentions fault-tolerant quantum operations by 2026 as a goal, implying Heron’s successors (e.g., the 433-qubit Osprey and 1121-qubit Condor and beyond) will incorporate QEC.
USTC Zuchongzhi 3.0
The USTC team has not reported building a logical qubit yet; instead, they focused on demonstrating quantum computational advantage (an ultrahard task for classical computers). Nevertheless, the error characteristics of Zuchongzhi 3.0 are in the same league as Google/IBM, which means it is in principle capable of running QEC codes. The average two-qubit gate error on Zuchongzhi 3.0 is ~0.38% (slightly higher than IBM’s 0.3% or Google’s 0.14%), and single-qubit error ~0.10%. These are just below the 1% threshold. By improving those fidelities a bit further (which could come from the continued materials optimization they described), they could attempt surface code experiments too. In their arXiv paper, the USTC researchers note that increasing qubit coherence and reducing gate errors directly “pushes the limits of current quantum hardware capabilities” and lays the groundwork for exploring error correction in larger circuits. Notably, even without full error correction, they ran a loose error mitigation during their random circuit sampling: they reset qubits frequently (via measure-and-reinitialize) to avoid error accumulation, and they performed statistical verification of outputs to ensure errors were not dominating the results.
For error correction proper, USTC might leverage similar codes as Google (since their lattice is compatible with surface code as well). They have expertise in quantum error correction from the photonic side (Pan’s group demonstrated some bosonic codes with photons). It wouldn’t be surprising if a next milestone from USTC is an error-corrected logical qubit too.
One interesting note: Zuchongzhi 3.0’s architecture with flip-chip could be beneficial for QEC because the second chip can incorporate circuitry for fast feedforward or crosstalk isolation needed in error correction cycles. Also, their use of active reset of qubits (via gates) between rounds is essentially part of an error correction cycle (resetting ancilla qubits).
In summary, while Zuchongzhi 3.0 hasn’t demonstrated a logical qubit, its physical error rates (~0.1–0.4%) are low enough to be at the brink of the error correction threshold. The team’s priority so far was achieving a quantum advantage experiment, but the same hardware improvements (longer T1, T2, better gates) directly translate to enabling error-corrected computations in the near future. Given that Willow and Zuchongzhi are so similar, we can expect that if Google can do distance-7 QEC, USTC’s device could replicate a similar feat with some refinement. The researchers explicitly state that their advances in coherence and fidelity “open avenues for investigating how increases in qubit count and circuit complexity can enhance the efficiency in solving real-world problems” – which hints at error correction as one such avenue for real-world algorithms.
Summary of Error Rates and Error Correction Techniques
Two complementary paradigms have emerged among these five: (1) Intrinsic error reduction: AWS and Microsoft drastically reduce physical error rates by qubit design (cat qubits, topological qubits), aiming to minimize the burden on error correction codes. (2) Active error correction: Google, IBM, and USTC improve their superconducting qubits to the threshold regime and then apply quantum error correcting codes (like the surface code) to suppress errors further. Both approaches are racing toward the same goal of fault-tolerant quantum computation. A simple threshold condition inequality $$p_{\text{phys}} < p_{\text{thresh}}$$ underpins both – AWS/Microsoft satisfy it by making $$p_{\text{phys}}$$ extremely small for certain errors, and Google/IBM/USTC satisfy it by engineering $$p_{\text{phys}}$$ below the known $$p_{\text{thresh}} \sim 1%$$ for surface codes. Ultimately, all five efforts aim to realize logical qubits with error rates low enough for deep, reliable quantum circuits. Already, we see Ocelot achieving a working logical qubit with an 85% resource reduction, and Willow demonstrating a logical memory that outperforms any single physical qubit. These are strong validations that error correction – whether hardware- or software-intensive – is on the verge of making quantum computing scalable.
Benchmarking and Performance Metrics
To compare quantum processors, a variety of benchmarks and metrics are used, from device-independent metrics like Quantum Volume to task-specific benchmarks like random circuit sampling. We will consider a few key performance measures: quantum volume (QV), Circuit Layer Operations Per Second (CLOPS), algorithmic benchmarking (quantum advantage experiments), and other published performance numbers like fidelity scaling in large circuits.
Quantum Volume
QV is a holistic metric introduced by IBM that accounts for number of qubits, gate fidelity, and connectivity by finding the largest random circuit of equal width and depth that the computer can implement with a success probability > 2/3. A higher QV (which is typically reported as $$2^d$$ for some integer $$d$$) means the machine can handle larger entangled circuits reliably.
IBM Heron R2
Among our five chips, IBM Heron R2 currently has the highest reported QV. IBM announced that Heron achieved Quantum Volume = 512 (since a heavy-hex 127-qubit Eagle had reached QV 128 earlier, Heron’s better fidelity pushed it to 512). QV 512 corresponds to successfully running a random 9-qubit circuit of depth 9 (since $$2^9=512$$). This indicates Heron can entangle at least 9 qubits deeply with good fidelity.
Google Willow and USTC Zuchongzhi 3.0
Neither Google nor USTC have formally reported QV for Willow or Zuchongzhi, but given their specs, one can infer. Willow with 105 qubits and 99.86% two-qubit fidelity could likely achieve a similar or higher QV (perhaps 512 or 1024) if measured, because it has even more qubits to trade for circuit depth. Google typically focuses on specific milestones rather than QV, but the capabilities demonstrated (like a full 105-qubit random circuit at depth > 30 in the advantage experiment) far exceed what QV 512 implies. In fact, QV becomes less informative at the cutting edge where targeted benchmarks are more illustrative (for instance, Google might say their quantum volume is effectively unbounded for random sampling tasks given they beat classical by a huge margin).
AWS Ocelot
AWS Ocelot’s QV is not directly applicable, since it currently realizes one logical qubit – QV is defined for circuits, so a single logical qubit can’t generate an entangled multi-qubit circuit. The concept of QV will apply to Ocelot only when it’s scaled to multiple logical qubits that can run a non-trivial circuit. However, one could consider the logical qubit’s lifetime or error rate as analogous performance measures (discussed above).
Microsoft Majorana-1
Microsoft Majorana-1’s QV is also not yet meaningful – with 8 physical qubits that are in early experimental stages, they haven’t run random circuits. The purpose of Majorana-1 isn’t to maximize QV at this stage but to validate the new qubit type. So in terms of QV: IBM leads with 512, and Google/USTC likely have comparable or higher effective circuit capabilities though they don’t frame it in QV terms. It’s worth noting that another company, IonQ, has claimed very high QVs (e.g., 2,097,152), but that is for trapped-ion systems with lower gate speed; our focus is on these five chips. Among these five, Heron R2’s QV 512 is a concrete benchmark of its balanced qubit count and fidelity.
CLOPS (Circuit Layer Operations Per Second)
This IBM-defined metric measures how many layers of a parameterized circuit the system can execute per second (including compilation and feedback overheads). It gauges the throughput or speed of executing quantum circuits.
IBM Heron R2
IBM Heron R2 (on IBM Quantum System Two) has achieved a CLOPS of 150,000+ layers per second. This is an impressive number, about 50× higher than what IBM had a couple of years prior. It reflects improvements in both the physical chip (fast gates, parallel operation) and the software stack (qubit reuse, better scheduling, and a new runtime). For context, a CLOPS of 150k means one can run 150k layers of a 100-qubit circuit in one second (if each layer is a set of single- and two-qubit operations across the 100 qubits). IBM achieved this by reducing the idle times between operations and by introducing parametric compilation (so repeated circuit executions don’t need full recompilation).
Google Willow
None of the other vendors have officially published a CLOPS for their devices, but qualitatively: Google’s processor has very fast gates (see Gate Speed section), so physically it could have high CLOPS, but Google’s software stack is not as openly benchmarked as IBM’s. IBM has put emphasis on making the entire system fast and user-friendly (since it’s accessible via cloud to users) – hence metrics like CLOPS. Google’s team, primarily focused on internal experiments, might not optimize for running as many circuits per second for external users. If we consider raw capability, Google’s 25 ns single-qubit and 42 ns two-qubit gates are faster than IBM’s ~35 ns and ~200 ns gates, so physically Google could do more layers per second on hardware. However, CLOPS includes compiler and control electronics latency. IBM’s achievement of 150k CLOPS was due to highly optimized classical control and streaming of circuits (they mention new runtime and data movement optimizations). It’s likely that Google’s system (as used in their lab) is not optimized for high-throughput in the same way; it is optimized for specific large experiments.
USTC Zuchongzhi 3.0
Meanwhile, USTC’s Zuchongzhi 3.0 – being a research prototype – likely has relatively low throughput (they took “a few hundred seconds” to collect 1 million samples of a 83-qubit random circuit, which implies on the order of $$10^3$$ – $$10^4$$ circuit layers per second, not nearly 150k). But again, that system wasn’t optimized for user throughput, it was optimized to push qubit count and fidelity.
AWS Ocelot and Microsoft Majorana-1
AWS Ocelot and Microsoft Majorana-1 also are not at the stage of needing CLOPS benchmarking; Ocelot runs one logical qubit (so CLOPS is moot), and Majorana-1’s focus is on qubit stability, not running many circuits. In summary, IBM leads in quantum computing throughput with 150k CLOPS on Heron R2, a metric that underscores the integration of a fast chip with an efficient software stack. This means IBM’s system can execute, say, variational algorithm circuits extremely quickly, which is useful for research and commercial cloud offerings. Google and USTC haven’t emphasized this metric, but their chips excel in other benchmarking areas as discussed next.
Quantum Advantage / Computational Task Benchmarks
Perhaps the most dramatic benchmark is solving a problem believed to be intractable for classical supercomputers – often termed “quantum supremacy” or quantum advantage demonstration. Both Google and USTC have excelled here with their latest chips:
Google Willow
Willow performed a random circuit sampling (RCS) task with 105 qubits and around 24 cycles (layers) of random two-qubit gates, generating a huge number of bitstring samples. They reported that Willow completed in about 5 minutes a computation that would take Frontier (the world’s fastest supercomputer) an estimated $$10^{25}$$ years to simulate. This is 10 septillion years – billions of billions of years, far longer than the age of the universe. In practical terms, this firmly establishes a quantum computational advantage – no existing classical method can replicate that specific random circuit sampling experiment. This experiment is essentially an extension of Google’s 2019 supremacy test (which was 53 qubits, 20 cycles). With Willow’s higher fidelity, they could go to 105 qubits and deeper circuits while still getting statistically meaningful results (verified by cross-entropy benchmarking). The results are staggering: they pushed the boundary of quantum sampling by six orders of magnitude in classical difficulty beyond the previous state-of-the-art (USTC’s earlier 56-qubit, 20-cycle experiment). This is not an “application” in the useful sense, but it is a crucial benchmark of raw computational power. It shows Willow can entangle over 100 qubits and perform >1,000 two-qubit gates in a complex circuit with enough fidelity that the output has structure that can be measured. The fidelity of the whole circuit was low but detectable (a tiny fraction of a percent, which is expected at that scale), yet it beat brute-force simulation by a huge margin.
USTC Zuchongzhi 3.0
Zuchongzhi 3.0 likewise demonstrated quantum advantage. They ran an RCS experiment with circuits of 83 qubits for 32 cycles, collecting one million samples in just a few minutes. They estimate Frontier supercomputer would take $$6.4×10^{9}$$ years (6.4 billion years) to do the same. While 6.4 billion years is less mind-boggling than Google’s $$10^{25}$$, it still qualifies as a quantum advantage demonstration by a huge margin. In fact, USTC’s earlier chip (Zuchongzhi 2.1) had already claimed an advantage (with 66 qubits, 20 cycles). Zuchongzhi 3.0’s advantage is more robust due to higher fidelity: by increasing circuit depth to 32 and qubit count to 83, they made the classical task exponentially harder. The quantum experiment is roughly on par in scale to Google’s 2019 experiment, and about 2–3 orders of magnitude beyond what classical algorithms had caught up to after 2019. It’s noteworthy that Willow’s experiment went even further: 105 qubits, similar cycles, thus pushing beyond Zuchongzhi 3.0 by a big factor (hence Google’s $$10^25$$ vs USTC’s $$10^9$$ year claim). The Quantum Computing Report compared Willow and Zuchongzhi 3.0 and found Willow had a slight edge in key qubit quality metrics, which allowed a larger, harder RCS experiment. Indeed, as listed earlier, Willow’s average two-qubit fidelity (99.86%) is higher than Zuchongzhi’s (99.62%), giving Willow an edge in executing deeper circuits with sufficient fidelity. Both chips outpaced IBM’s publicly reported experiments in this domain – IBM has not attempted an RCS at that scale publicly (IBM did a 127-qubit, depth-12 “utility” circuit which was classically simulable with effort, and IBM argues for focusing on useful tasks rather than contrived supremacy tasks ).
IBM Heron R2
Instead of chasing RCS supremacy, IBM demonstrated what they call a “100×100 challenge” milestone – running a circuit with 100 qubits × 100 depth (10,000 two-qubit gate operations) with meaningful results. At the Quantum Developer Conference 2024, IBM announced they can run circuits with 5,000 two-qubit gates (and indeed up to 10,000 in some cases) on Heron R2 with high fidelity outputs. They compare this to the earlier “quantum utility” experiment on Eagle which used 2,880 two-qubit gates. Achieving 5,000 entangling operations without the result being complete garbage is a big deal – it means errors did not compound too quickly. IBM did not frame it as a supremacy demonstration, but rather as enabling useful-sized circuits beyond what classical can simulate exactly. 100 qubits × 50 depth (5k gates) is indeed beyond exact brute-force simulation (Hilbert space dimension $$2^{100}$$ is enormous), though classical approximations or sampling could handle certain types of circuits of that size if the circuit structure is special. IBM’s emphasis was that clients can run such large circuits on the system reliably now, indicating a focus on near-term practical algorithms (which often involve many gates but require some fidelity to get a result). So Heron R2’s benchmark is “5,000 two-qubit gate circuit executed with 50× faster run-time than before” – a different flavor of progress than Google’s 1e25-year supremacy claim, but still important. It shows IBM’s machine has entered a regime of complexity (100 qubits, depth ~50–100) that is at or just beyond what classical simulation can do for arbitrary circuits. IBM’s QV and CLOPS already indicated this capability, and the 5k-gate demonstration confirms it concretely.
AWS Ocelot and Microsoft Majorana-1
These two are not yet judged by large circuit benchmarks, as they are at the component stage. Ocelot’s achievement is creating a stable logical qubit, which could be benchmarked by memory time or logical error per sequence (which presumably is very good – possibly no logical error seen over many operations since bit-flips are absent). One might measure a “logical T1” or “logical error per Clifford” for Ocelot as a performance metric, but those numbers aren’t public. Majorana-1’s benchmark is likewise the demonstration of braiding/measurement operations with <1% error and ms stability, as discussed. So for now, AWS and Microsoft can’t claim high quantum volume or solving intractable problems; instead, their “benchmark” achievements are in the error suppression domain (90% reduction in overhead, world’s first topological qubit, etc.).
Other Benchmarks
We should mention gate depth and fidelity in algorithms. Google demonstrated that using surface code error correction, a distance-7 logical qubit can preserve quantum information for >2× the duration of a physical qubit – essentially a quantum memory benchmark. Also, they showed a logically encoded gate (a logical CNOT or CZ) can be performed with error ~0.14%, which is an interesting metric: the fidelity of an error-corrected logical gate. That 0.143% logical CZ error (as reported in Oezratty’s summary of Google’s preprint) is “not bad” as he says – it’s on par with physical gate errors, indicating the overhead is starting to pay off. Another metric is readout fidelity – the accuracy of measuring qubits. From the earlier table: Willow’s single-shot readout fidelity is ~99.33%, Zuchongzhi’s 99.18%. IBM hasn’t published a single number, but in their Eagle generation it was around 95–99% typically; Heron likely improved to similarly >99%. The higher the readout fidelity, the fewer errors in final measurements or in mid-circuit syndrome measurements for QEC. Microsoft’s Majorana readout was 99% (1% error) in initial tests, which is already competitive and expected to get better. AWS Ocelot likely uses multiple ancilla measurements; their effective logical readout fidelity hasn’t been stated but presumably high as well (they can do repeated QEC until confident of result).
In terms of practical algorithm benchmarks, none of these chips have definitively solved a useful problem better than a classical computer yet – we are still in the preparatory phase. However, IBM and others often run small instances of algorithmic problems to test performance:
IBM, for example, demonstrated a 127-qubit circuit simulating a differential equation (the “utility” experiment) that yielded a meaningful result which matched theory. It wasn’t something classical computers couldn’t do, but it was a stepping stone showing that 100-qubit circuits can produce valid physical results (like observing a physics phenomenon) with error mitigation.
Google has used earlier chips to simulate simple chemistry problems (e.g., computing the energy of a light molecule via variational algorithms) and to observe many-body physics phenomena (like creating a time crystal in a periodically driven spin system). Those were proof-of-concept simulations. With Willow’s increased power, they could attempt larger simulation tasks (perhaps simulating a modest chemical reaction dynamics or a larger spin model) and see quantum signatures that are hard to get classically.
USTC’s group, aside from random circuits, also did Gaussian boson sampling with photonics and some simple quantum chemistry on superconducting qubits. They might try to use Zuchongzhi 3.0 for tasks like quantum phase estimation on small problem instances or optimization tasks, though no specific results announced yet.
One common benchmarking tool is Quantum Volume which we covered, and another is entanglement capability (like how many qubits can be entangled in a GHZ state). Usually, these chips can entangle all their qubits (e.g., generate a 100-qubit GHZ state, though fidelity would drop with more qubits entangled). Not sure if that specific was published, but likely.
Circuit fidelity at scale
Google’s cross-entropy fidelity for the full 105-qubit circuit was extremely low (~1e-3 or lower in absolute probability), but that’s expected – still it was enough to demonstrate a signal above noise. IBM’s 100×100 circuit likely had some aggregate fidelity that allowed extracting the result (maybe improved by error mitigation). These high-level metrics are complex but essentially both IBM and Google showed they can run circuits at the edge of what’s classically possible and still get a verifiable answer.
Summary of Benchmarking and Performance Metrics
To summarize the benchmarking landscape:
IBM Heron R2 excels in quantum volume (512) and throughput (CLOPS 150k), and has demonstrated running up to 5,000 gate circuits (100× the previous generation) reliably.
Google Willow leads in quantum computational advantage – solving a random circuit problem vastly out of reach for classical (~$$10^25$$ vs ~$$10^9$$ years for its competitors). It also demonstrated fault-tolerant logical qubit operation (distance-7 code) – a benchmark in error correction performance.
USTC Zuchongzhi 3.0 achieved a major quantum advantage experiment as well ($$10^9$$ year classical cost), underlining it as one of the most powerful processors, second only to Willow in that specific measure so far. Its qubit quality metrics are just slightly behind Willow’s, but very close.
AWS Ocelot and Microsoft Majorana-1 are not measured by large circuit benchmarks yet, but their success is measured by how well they hit error correction milestones. AWS’s key performance claim is 90% reduction in error-correction resource overhead (i.e., needing one-tenth the qubits for a logical qubit compared to conventional schemes). That can be viewed as a “figure of merit” for fault-tolerance efficiency. Microsoft’s performance claim is having built a qubit that can potentially scale to a million with each still maintaining coherence – effectively claiming a breakthrough in scalability potential. They haven’t given numeric benchmarks besides the existence of Majorana zero modes and stable operation for an hour-scale experiment. But in a qualitative sense, if one extrapolates, a million Majorana qubits at ~0.1% error rate would have an astronomical quantum volume and could, in theory, break modern cryptography or simulate complex chemistry without needing complex codes – though that’s aspirational at this point.
In conclusion, benchmarking results illustrate that IBM and Google are at the forefront of general quantum computing performance – IBM highlighting broad and fast circuit capability (QV, CLOPS), Google highlighting feats in error correction and quantum supremacy. USTC’s Zuchongzhi 3.0 is very close behind, essentially matching Google in qubit count and approaching in fidelity, thus also achieving a form of supremacy. AWS and Microsoft show their strengths in more specialized metrics related to error correction (logical qubit overhead, stability), which will translate to performance when they scale up. Each metric gives a different slice of performance: QV encapsulates balanced performance, CLOPS emphasizes speed, and quantum advantage experiments test the extreme limit of computational power. Together, they paint these chips as the most advanced quantum processors in the world, each excelling on different axes.
Gate Fidelity and Speed
The fidelity (accuracy) of quantum gates and their speed (duration) directly affect a quantum computer’s ability to run algorithms reliably. High-fidelity gates minimize error per operation, and fast gates allow more operations within the coherence time. We’ll compare 1-qubit and 2-qubit gate performance for the five chips, noting any special gate designs or innovations:
AWS Ocelot
While specific numerical gate fidelities for Ocelot haven’t been published, we can discuss the nature of its gates. Ocelot implements the first bias-preserving gates on cat qubits. This means the gate operations are designed not to introduce bit-flip errors that weren’t already present. In a conventional system, a control-X gate, for example, could convert some phase noise on the control qubit into a bit flip on the target. In Ocelot, such gates are engineered (likely through complex pulse sequences on the multi-component cat qubit system) to maintain the error bias. Achieving high fidelity while doing this is non-trivial, but AWS reports success in “tuning out” certain error modes. We know from the Nature paper that manipulating a cat qubit’s phase can be done without breaking its protection – they demonstrated coherent control (phase rotations) while still having 10 s bit-flip times. This implies the single-qubit gate fidelity on cat qubits can be very high; essentially limited by phase error during the gate. If a single-qubit rotation takes, say, 100 ns, and T2 for phase is ~0.5 µs, then a rough estimate of fidelity is $$1 – 100\text{ns}/500\text{ns} = 0.80$$ (80%) if done naively. But likely the buffers and echo techniques improve the effective T2 during gates, yielding much higher fidelity. Possibly they use error-transparent gate techniques that ensure any phase error is trackable.
The AWS press emphasizes reduced errors by up to 90%, hinting that gate infidelities are an order of magnitude lower than they would be without the cat approach. Because Ocelot uses a small logical qubit, one could also quote its logical gate fidelity – presumably significantly higher than a physical transmon’s gate fidelity, since 9 physical qubits back it. If each physical gate is, say, 99%, and error correction corrects most single faults, a logical gate might be ~99.9% effective. We don’t have exact numbers, but it’s clear Ocelot’s selling point is fewer errors per gate by design.
On speed: cat qubit gates might be slightly slower than standard gates because they involve manipulating an oscillator state (which might require longer pulses or multiple steps). However, AWS indicates this is still implemented on a microchip with standard techniques, so gate times likely remain in the nanoseconds to sub-microsecond range. Perhaps single-qubit gates on cat qubits are tens of nanoseconds (comparable to transmons), and two-qubit entangling gates (like a controlled-phase between cat qubits) might involve intermediate ancilla operations, taking maybe a few hundred nanoseconds. As Ocelot is a prototype, optimizing speed wasn’t the primary goal; achieving bias-preserving fidelity was. We can expect as they refine it, gate times will be brought down to typical superconducting time scales.
Microsoft Majorana-1
In Majorana qubits, gates are typically done either by physically braiding MZMs (which in practice means changing the Hamiltonian connections in a sequence) or by measuring certain multi-qubit operators to enact gate teleportation. These processes are generally slower than a single microwave pulse, but can be done with high fidelity due to topological protection. Microsoft’s approach involves measurement-based gates – using projective measurements of parity to implement logic (this is related to magic state injection and measurement-based computation). The Majorana qubit readout itself takes on the order of a few microseconds (as it involves microwave reflectometry) – initial measurements had 1% error, which is quite good for a first try. A coherent topologically protected braiding operation can be slow: one might need to adiabatically vary gate voltages over microseconds to swap Majoranas. However, since no fragile phase needs to be maintained locally, this slowness doesn’t incur much additional error (the qubit’s T2 is effectively very long for protected operations). So one can trade speed for fidelity here. If it takes e.g. 1 µs to enact a braiding operation, that’s fine because the qubit can sit for 1 ms without flipping. In effect, Majorana qubits have a very favorable ratio of coherence time to gate time – possibly $$T_{\text{gate}} \sim 1\text{µs}$$, $$T_{\text{coherence}} \sim 1\text{ms}$$, giving a 1:1000 ratio, much better than transmons (~1:100). Thus, even if gates are slower, error per gate can be extremely low.
Microsoft hasn’t quoted gate fidelities yet (as doing a full braiding gate on Majorana-1 is likely the next step). But theoretically, swapping two Majoranas (which yields a topologically protected $$e^{i\pi/4}$$ phase gate on the qubit) should be virtually error-free if done adiabatically and isolated from environment. The only errors would come from quasiparticles appearing (which is that ~1/ms parity flip rate) or from control imperfections that break adiabaticity. Given their results, one can speculate single-qubit Clifford gates (like an $$X$$ or $$Z$$ on the topological qubit, which might be done via a sequence of parity measurements or braids) could be fidelity >99.9%. The T-gate (non-Clifford) is not protected and would involve injecting a state with some error – they might realize T-gates via magic state injection with some overhead. The fidelity of a T-gate then depends on the fidelity of preparing a magic state (which could be, say, 99% and then distilled to higher). But that’s more a future concern when doing full algorithms.
In terms of speed: measurement-based gates on Majorana-1 will be limited by measurement time (a few microseconds per parity measurement). So a sequence implementing a CNOT between two topological qubits might take maybe tens of microseconds. That is slower than a ~200 ns transmon CNOT, but if it’s 100x more reliable, it’s a worthy trade-off. And since each topological qubit acts as a logical qubit, you’d need far fewer of them – so you can afford slower gates if it saves thousands of physical operations.
In summary, Majorana-1’s single-qubit gate fidelity is expected to be extremely high (with the exception of T gates needing auxiliary methods), and two-qubit gates will likely be implemented via sequences of reliable measurements. Speed per gate is slower (microseconds), but given the much longer coherence, the “gate operations per coherence time” might actually be higher than in superconducting systems (e.g., if T2 ~1 ms and gate ~5 µs, you could do ~200 topologically protected operations within coherence, whereas in a transmon T2 ~100 µs and gate ~20 ns, you could do ~5000 operations – so actually transmons still allow more operations in absolute count, but those ops carry error that needs correction).
Google Willow
Google’s Willow chip has very fast gate speeds and excellent fidelities, representing perhaps the current pinnacle of superconducting gate performance. The average single-qubit gate fidelity on Willow is 99.965% (error ~$$3.5×10^{-4}$$). Single-qubit gates (like $$X/2$$ rotations) on transmons are typically done with ~20–25 ns microwave pulses. Indeed, Willow’s single-qubit gate time is reported ~25 ns. With T1 ~98 µs, the error from decoherence in 25 ns is negligible (~0.025%). The remaining error comes from control imperfections (amplitude and phase errors in the pulse, crosstalk, etc.), which they have tuned down to the 1e-4 level – an impressive calibration feat. For two-qubit gates, Google uses a variant of the iSWAP gate (sometimes referred to as a $$\sqrt{i\text{SWAP}}$$ or Sycamore gate). On Willow, the two-qubit gate fidelity averages 99.86% (error ~$$1.4×10^{-3}$$). This is remarkably high for such a large system – indicating uniform high-quality couplers and calibration. The two-qubit gate time is about 42 ns. This is extremely fast for a two-qubit entangling gate – by comparison, IBM’s cross-resonance gates are ~200 ns, and even their newer CZ gates (on certain tunable coupler designs) are ~100 ns or more. Google achieves 42 ns by using a direct microwave drive on a resonant coupling between qubits (essentially turning on an exchange interaction briefly). The short duration helps limit decoherence error, but requires precision and synchronization. Running all two-qubit gates simultaneously in a layer is possible due to their careful isolation (Willow can apply all couplers in parallel with only slight fidelity hit). In a simultaneous operation scenario, they measured an average two-qubit Pauli error of 0.38%, which is higher than the isolated error (0.14%), but still manageable. This parallel fidelity drop is due to spectral crowding and microwave cross-talk when many qubits are gated at once. Google likely optimizes the gate frequency allocation to minimize such interference (hence their claims of improvements over previous gen). With these gate speeds, Willow can perform on the order of 1000 two-qubit gate operations within each qubit’s coherence time (~100 µs / 0.042 µs ≈ 2380 gates max if sequential on one pair; in practice, parallel ops and idle times reduce the number, but still a large number). The high fidelities mean even large circuits can retain some fidelity (for RCS, as noted, they did ~1000 two-qubit gates across the chip and still had measurable correlation with ideal output).
Another aspect is readout speed and fidelity – Willow uses multiplexed dispersive readout with JPA amplification, achieving ~99.3% readout fidelity in maybe ~few microseconds integration time. That is on par with IBM’s readout (IBM reported ~95–99% fidelity in 0.5–1 µs on prior devices). So Willow’s I/O is also quite efficient, which helped in quickly collapsing the state for resets during QEC cycles.
In terms of innovations: Willow’s main innovation in gating is the all-microwave fast iSWAP and improvements in simultaneous gate calibration – allowing many gates at once with minimal interference, which is critical for high quantum volume and fast algorithm execution. The chip also likely uses some form of two-pulse echoed gate to cancel certain errors (like echo CR pulses in IBM, Google might do echoing to cancel single-qubit Stark shifts during two-qubit gates). Overall, Willow leads in raw gate speed among these chips and has fidelities at the cutting edge of what’s been achieved in any platform.
IBM Heron R2
IBM’s focus has been increasing fidelity, even if gate speeds are moderate. On Heron R2, IBM still uses the cross-resonance (CR) two-qubit gate (a microwave-activated CZ gate via a tunable coupler). However, with the introduction of tunable couplers, they can effectively turn off interactions when not gating, which reduced unwanted frequency shifts and cross-talk, allowing more precise calibration of CR pulses. The reported two-qubit gate fidelity is 99.7% on average (error ~$$3×10^{-3}$$). Some pairs may be as high as 99.8–99.9% (IBM often highlights best-case performance in smaller devices: e.g., 99.85% CZ on 2-qubit experiments). The single-qubit fidelity ~99.97% (error ~$$3×10^{-4}$$), similar to Google’s. The gate times on IBM Heron: single-qubit gates (X/2) ~35–50 ns typically. Two-qubit CR gate times are around ~200 ns on average now. IBM hasn’t explicitly published Heron’s gate durations, but from prior devices and slight improvements one can infer ~150–250 ns range for a controlled-NOT (CR pulse). IBM’s heavy-hex layout reduces the number of neighbors, simplifying frequency collision avoidance; combined with tunable couplers, they likely could shorten CR pulses a bit (because they can increase coupling during the pulse more aggressively when cross-talk is less). IBM also uses echo sequences in the CR gate (echoing on target qubit) to cancel Z errors and has analytic calibration techniques to minimize coherent errors. The result is that although 200 ns is much longer than Google’s 42 ns, IBM’s error is only about 2× higher – indicating the longer gate is very well calibrated. The advantage of a 200 ns gate is possibly less spectral broadening (less Fourier width) which might ease cross-talk. But it means fewer operations fit in the coherence time (100 µs / 200 ns = 500 operations theoretically, vs ~2380 for Google’s case). IBM mitigates this by cycling qubits between idle and active periods – heavy-hex and tunable couplers ensure that when a qubit is not participating in a gate, it’s as idle and decoherence-limited as possible (no additional drive noise). So not all qubits are accumulating gate error simultaneously. IBM’s single-qubit gates are also extremely good (99.97%). These are about 35 ns (they often use derivative-shaped DRAG pulses to minimize leakage, which they calibrate meticulously). IBM achieved T1’s long enough (some in 100 µs+) that 35 ns gates are negligible error from decoherence (~0.035/1000 = 3.5e-5). So the 3e-4 error is mostly from pulse imperfections/crosstalk, which IBM continuously works to reduce. Because IBM’s readout is also high-fidelity and faster now, they can do mid-circuit measurements for resets or QEC with decent reliability (not as high as Google’s reference—IBM’s readout might be slightly less fidelity per qubit, but they do repeated readout to purge entropy as needed).
One innovation in Heron R2 is the “TLS mitigation” which improved coherence and thereby indirectly improved gate fidelity stability across the chip. Another is that IBM introduced system-level calibration of many qubits – using techniques like Floquet calibration and scalable parameter tuning to handle the 156-qubit system. This contributed to the uniformity of 99.7% fidelity across 156 qubits, which is impressive (much like Willow’s uniformity).
IBM is also exploring alternative gates (there’s research into direct CZ via tunable coupler bias). It’s possible some two-qubit gates on Heron R2 are actually implemented as a direct CZ by biasing the tunable coupler for ~100 ns to induce an effective ZZ interaction. If so, that could account for high fidelity and might shorten the gate time relative to CR. IBM’s documentation indicates native gates include CZ on certain processors. If Heron supports a direct CZ, that could be faster (~100 ns) and high fidelity. The BlueQubit data doesn’t differentiate, but says “CZ” 99.7%. This suggests IBM might indeed be using CZ (which is symmetric, unlike CR which is one-directional but can be compiled into a CNOT). A 100–150 ns CZ gate at 99.7% fidelity is comparable to CR.
IBM’s heavy-hex connectivity is slightly less ideal for some algorithms (since degree-3 vs degree-4 means slightly more swap overhead for certain graph mappings), but it’s a deliberate trade for fidelity.
Overall, IBM’s gate fidelity is a little lower than Google’s but in the same ballpark, and their gate speeds are slower (roughly 5× slower two-qubit gates). IBM compensates with superior orchestration (parallelize what they can, fast classical feedback, etc.).
USTC Zuchongzhi 3.0
The Chinese chip’s gate performance is very close to Google’s, as shown in the direct comparison. Single-qubit fidelity ~99.90% (error 1e-3) and two-qubit fidelity ~99.62% (error 3.8e-3). These are slightly below Willow’s 99.965% and 99.86%, but not by much. The gate times were given as 28 ns for 1-qubit gates, 45 ns for two-qubit gates – essentially the same order as Google’s (25 ns, 42 ns).
The Chinese team uses a similar scheme of microwave-activated iSWAP-like gates. They mention specifically an “iSWAP-like gate” with improved calibration that achieves those 0.38% average error when all gates simultaneous. So Zuchongzhi’s gate style is presumably very akin to Google’s: fast, resonant exchanges. One difference: their connectivity is a full grid (degree-4) but they have slightly more decoherence (T2 58 µs vs 89 µs), which might be why their error is a tad higher. Also, they may not have exactly the same error mitigation on chip (Google has years of tuning experience with certain flux pulse shaping, etc.). Still, 99.6% fidelity for two-qubit gates is world-class. With 45 ns gate times, they too can perform ~1000 gates within T1.
The readout fidelity on Zuchongzhi is about 99.18%, slightly lower than Willow’s 99.33%, which could influence multi-round algorithms. But for non-QEC tasks like RCS, that’s not a limiting factor. The USTC team also did something clever: they adjusted their gate scheduling to specific patterns (ABCD-CDAB sequence) that minimized crosstalk, and inserted dynamical decoupling for idle qubits which improved overall fidelity of deep circuits. All these demonstrate an advanced understanding of gate-level control.
So Zuchongzhi 3.0’s gates are essentially on par with the best of Google/IBM – only marginally lower fidelity which they will likely continue to improve. The fact that two independent groups (Google and USTC) achieved sub-1% two-qubit errors with ~40 ns gates is an encouraging sign that the approach is reproducible and not unique to one lab.
Relevant Innovations
AWS’s bias-preserving gate design is an innovation enabling error correction with fewer qubits.
Microsoft’s Majorana readout and prospective braiding operations are innovations in achieving gates that don’t disturb the system (and essentially push classical control complexity into the measurement layer).
Google’s innovation was achieving exponentially decaying fidelity with system size that matches independent error models – meaning they’ve validated that their gate errors are mostly local and uncorrelated, following a simple exponential fidelity decay as circuits grow. This suggests no unknown error sources creeping in at scale, which is a big achievement in calibration uniformity. Scott Aaronson noted that Google’s and IBM’s observed exponential fidelity falloff is exactly what you’d expect from independent gate errors. That’s actually an affirmation that their gate fidelity numbers hold up in larger circuits and errors don’t compound worse than expected.
IBM’s tunable couplers and heavy-hex lattice are an architectural innovation that dramatically reduced certain errors (like spectator qubit shifts and cross-talk). This made their gates more robust when many are executed on a chip. For instance, IBM showed that heavy-hex+couplers eliminated frequency collisions that plagued previous processors and allowed executing gates in parallel with minimal added error.
USTC’s flip-chip integration can be seen as an innovation enabling them to run more qubits with fast gates without signal interference. They mention e.g. the flip-chip allowed them to increase coupling strength (for faster gates) while using a bandpass filter to avoid Purcell effect, thus keeping T1 high.
Summary of Gate Fidelity and Speed
Google’s Willow and USTC’s Zuchongzhi 3.0 have the fastest gates (~tens of ns) with errors around 0.1–0.3%, enabling extremely rapid operations. IBM’s Heron R2 has slightly slower two-qubit gates (~100–200 ns) but with very low error ~0.3%, and single-qubit gates essentially at the physical limits of $$10^{-4}$$ error. AWS Ocelot’s gates are bespoke but presumably already in the <1% error regime given the effective logical qubit formed – importantly, they preserve the qubit’s special error bias. Microsoft’s Majorana-1 promises near error-free Clifford operations albeit at microsecond timescales, with only special gates needing assistance. All chips aim for a high gate fidelity × speed product – roughly, one can multiply $$(gate fidelity)^{(number of gates per coherence)}$$ to get an idea of how complex a circuit they can handle. Google and IBM both push towards being able to perform thousands of gates within coherence with only a few percent total error probability. This is exactly what allowed IBM’s 100×100 circuit success and Google’s deep circuit supremacy. We also see that scaling up qubit count has not compromised individual gate quality drastically – Willow at 105 qubits still has ~0.1% errors, Heron at 156 qubits ~0.3%. This indicates the control systems and calibration protocols have scaled well, which is a critical sign for future larger chips.
Scalability and Integration
A crucial aspect of quantum technology is how readily each approach can scale to larger numbers of qubits and how to integrate those qubits into a functional system. We examine the prospects and strategies for scaling for each of the five:
AWS Ocelot
Ocelot is designed with scalability in mind by leveraging standard microfabrication and a modular approach to error correction. The current Ocelot chip is a prototype logical qubit; scaling it means replicating many such logical qubit units and connecting them. AWS has emphasized that Ocelot’s architecture can be manufactured using processes borrowed from classical microelectronics, such as thin-film deposition of tantalum for high-Q resonators on chip. This implies that making chips with dozens or potentially hundreds of cat qubits (plus ancillas) is feasible with existing fab infrastructure.
The big win for Ocelot is resource reduction: they estimate needing only ~100,000 physical qubits to build a useful fault-tolerant quantum computer, instead of ~1,000,000 as often estimated for other approaches. This ~10× improvement means the scaling target is an order of magnitude less demanding. If each Ocelot chip can host, say, 10 logical qubits (just as an example, if it were a slightly larger chip with 10 groups of 14 components), then 10,000 such chips would be needed to reach 100k physical qubits. That’s still a huge number, but AWS is a cloud infrastructure company comfortable with deploying massive numbers of processors in data centers. They could aim to tile many small quantum modules (each with some logical qubits) and network them (perhaps via optical or microwave interconnects).
In the near term, the next step would be to demonstrate two logical qubits on one chip entangled, which would require scaling from 14 qubit components to maybe 28+ (for two logical blocks plus connecting ancillas). There’s no fundamental barrier cited in doing that – the chip area might double, control wiring will increase, but since each cat qubit is an oscillator, multiple can possibly share some infrastructure like readout amplifiers.
Also, AWS notes that chips built in the Ocelot architecture “could cost as little as one-fifth of current approaches” due to fewer qubits needed. Lower cost suggests simpler scaling in production yield – if only 9 qubits yield a logical qubit, then even if one or two qubits have imperfections, error correction might tolerate it.
We also know Ocelot is implemented in a planar geometry using transmons and resonators, which is inherently scalable to a few hundred elements on a chip (IBM and Google put ~100 qubits on a chip of a few cm²; AWS could do similar). The integration challenge will be wiring up many resonators and transmons for control without cross-talk; AWS’s partnership with Caltech likely has been tackling wiring cross-capacitances and such in the cat qubit lattice.
Another aspect is 3D integration: classical processors use billions of components because of 3D layering; quantum chips are mostly planar right now. AWS could potentially stack chips or use multiple layers for connectivity (like flip-chip, as USTC did). Because their cat qubits are oscillators requiring capacitive coupling, integrating many of them might require careful 3D wiring to avoid frequency collisions. However, AWS likely can leverage the same 3D wiring strategies IBM and USTC have (like feedlines on one chip, qubits on another).
In summary, Ocelot’s approach appears scalable in manufacturing (no exotic materials, uses proven superconducting fab) and scalable in architecture (each logical qubit uses a fixed, small number of physical qubits). The big to-do is scaling the number of qubits: from 9 physical to thousands, which they’ll achieve by replication and tiling. AWS’s end vision is a modular quantum computer where error-corrected qubits are the basic units – Ocelot accelerates reaching that by needing fewer physical qubits per unit. While there will be engineering challenges (like packaging many microwave resonators, and handling increased heat load in a dilution refrigerator for more control lines), these are akin to those faced in the superconducting transmon approach, so solutions will carry over (e.g., cryo multiplexers, etc.). AWS explicitly believes Ocelot architecture can “accelerate our timeline to a practical quantum computer by ~5 years,” indicating confidence that scaling won’t require as long as other methods might.
Microsoft Majorana-1
Microsoft’s approach banks on extreme scalability, if the physics works out. They claim the Majorana-based Topological Core can integrate up to a million qubits on a single chip. The reason this is plausible is that the qubit elements (nanowires, gates, quantum dots) are all semiconductor structures that can be defined by lithography, much like classical transistor circuits. In effect, building a million topological qubits is similar in complexity to building a modern computer chip with millions of transistors – albeit with added constraints of cryogenics and material homogeneity.
Microsoft’s recent breakthrough was the creation of a “topological superconductor” material (called a topoconductor) that reliably hosts MZMs. This material innovation (InAs/Al heterostructures with gating) can potentially be scaled by the wafer, using techniques from the semiconductor industry. Indeed, Microsoft has been developing fabrication processes to make arrays of nanowire devices on 300 mm wafers. The Majorana 1 chip has 8 qubits; the next generation might have, say, 16 or 32. They foresee a 4-generation roadmap: (1) single qubit device (demonstrated now), (2) two-qubit device with braiding operations (likely next), (3) a small network (maybe ~>10 qubits) to show a logical qubit with T-gates, (4) a scaling to a full plane of qubits (which could be hundreds or more).
The nature of topological qubits means that error rates don’t blow up when scaling: each qubit is largely independent except when deliberately coupled via measurement circuits. So adding more qubits primarily adds more wiring and control gates. One challenge is that the Majorana qubits need a global magnetic field to be in the topological regime – that means the entire chip must be under a uniform magnetic field (~0.5 T perhaps). This complicates integration with superconducting components (some parts might need to be ferromagnetic shielded or use materials that work under field). They’ve likely chosen materials that are resilient (e.g., aluminum thin film becomes superconducting but with suppressed critical field maybe offset by geometry).
For scaling, Microsoft will also have to integrate CMOS control electronics at cryogenic temperatures to handle millions of qubit gate electrodes – they have research in that direction (using cryo-CMOS to multiplex many control lines).
Another integration aspect is connecting distant qubits: in a million-qubit chip, not every qubit can be directly connected (that would be a wiring nightmare). Instead, they’ll probably use an architecture akin to a 2D grid or modular blocks of qubits connected by a routing network (which could be done via measurement-based entanglement swapping between adjacent blocks).
The roadmap to fault-tolerant quantum computation paper on arXiv describes an array approach (likely surface-code-like but with topological qubits as nodes). Indeed, they mention 4 generations culminating in an array of topological qubits with error correction on top to handle the remaining T-gate errors. The estimated million qubits might refer to physical Majorana “building blocks” that would equate to, say, ~1000 logical qubits if they still need some error correction. Nonetheless, Majorana qubits have arguably the best scaling outlook if they fully work: because each qubit is high-fidelity, you can tile many of them without introducing huge overhead, and they’re built with conventional nanofabrication.
On the engineering side, Microsoft will need to ensure uniformity – each of the million nanowires must behave consistently, which is a tall order (variations in the semiconductor, disorder, etc. could cause some qubits to fail). However, classical chip makers routinely manage billions of transistors with certain yield; Microsoft will leverage that expertise and also incorporate redundancy (maybe include spare structures that can be turned into qubits if some fail, analogous to redundancy in memory chips).
Integration also involves the cryostat scale: a million qubits likely won’t fit in a single small dilution fridge if each has separate connections. But if cryo-CMOS multiplexing is used, they could drastically cut the number of wires. Alternatively, they might distribute qubits across multiple cryomodules connected via quantum interconnects (similar to how IBM envisions linking 1121-qubit modules). But Microsoft seems to aim for a monolithic chip with many qubits – perhaps using advanced packaging to incorporate control chips in the same fridge. They showcased a 55-qubit planar cable in Majorana 1 for controlling gates; scaling that to thousands might require multi-layer wiring.
In summary, Majorana qubits have a clear path to scaling in principle: rely on semiconductor industry techniques to mass-produce qubit devices, use digital electronics-like control (voltage pulses, measurements) rather than purely analog microwave pulses, and exploit the fact that fewer qubits are needed for the same logic due to inherent stability. Microsoft’s Station Q team essentially aims to transition quantum hardware from “physics experiment” to “engineered processor” by using topologically robust components. If they succeed, scalability could leapfrog others (they might skip to hundreds or thousands of qubits in one go once the design is proven, because it’s more about repeating units on a chip, not fighting decoherence scaling). The risk is that if any part of the qubit fabrication doesn’t scale (e.g., if yield of topological qubits is low per chip), it could complicate the plan. But given recent Nature results confirming the existence of the phase and MZMs, optimism is warranted. In short, Majorana-1’s architecture is conceptually one of the most scalable, aiming for integrated million-qubit chips, though it’s at an earlier stage of demonstration.
Google Willow
Google’s scaling strategy has been incremental so far – they went from 9 qubits (Bristlecone early experiments) to 54 qubits (Sycamore) to 72 qubits (unused fully, they built one called “Bristlecone” but it had lower fidelity), then to 70 qubits (used in 2021 quantum supremacy 2.0) and now 105 qubits (Willow). Each step involved increasing qubit count while adjusting design to maintain fidelity. Google likely will continue doubling qubit count or so in future chips, provided fidelity remains high. However, superconducting qubits face some physical scaling limits: control wiring and cross-talk grow as qubit count grows. Willow at 105 qubits already has a dense arrangement of control lines.
Google uses a 2D grid connectivity, which is more connection-heavy than IBM’s sparse heavy-hex. This means each qubit has 4 neighbors max – great for algorithms, but also more couplers (Willow had 104 couplers for 105 qubits presumably, maybe more). The flip-chip 3D packaging approach is one way to handle scaling: USTC used it for 66 and 105 qubits; IBM uses through-silicon vias and multi-layer routing in their packaging. Google has not publicly detailed their packaging, but it’s likely they are also moving to flip-chip. (An indicator: The Chinese report noted their device is similar to Google’s and has the same qubit count and lattice, suggesting Google possibly also did flip-chip for Willow to integrate more qubits, or they found another way to jam 105 qubits in one layer by smarter wiring). For further scaling, modular quantum computing is an approach Google will likely adopt. They have published research on quantum interconnects (for instance, using photons to connect distant superconducting qubits). It’s expected that at some point, instead of one chip with, say, 1000 qubits (which might be challenging in one cryostat due to wiring heat load and size), they might use 10 chips of 100 qubits each connected via microwave or optical links. Indeed, other efforts (like the US IARPA Quantum Logical Qubit program) are exploring multi-chip modules for superconducting qubits. Google could connect chips via coax lines using tunable couplers bridging chips (like HQAN research on that) or via conversion of microwave to optical signals (they have projects in that space).
In terms of integration, Google built their own quantum data center with multiple cryostats and automated control – to scale experiments, they will probably parallelize across multiple cryogenic systems for error correction research (e.g., distribute logical qubits across hardware for reliability). However, to solve one larger problem, connecting qubits within one system is needed. Google’s timeline suggests they intend to achieve a useful error-corrected calculation by end of decade. They have not publicized a precise qubit roadmap (unlike IBM), but one can infer: they’ll need on the order of a few thousand physical qubits for a logical circuit (if each logical qubit uses ~50 physical qubits with distance ~11 code to get very low error, and then you need maybe 100 logical qubits for some interesting algorithm). So scaling from 105 to a few thousand physical qubits is a factor of 20–50. Achieving that on one chip might be done by a tiling approach: possibly using a larger die size (if current chip is maybe 2 cm x 2 cm, they might go to 4 cm x 4 cm and double density with flip-chip, etc.). But extremely large die can suffer yield issues. Another approach: modular architecture – e.g., four chips of 256 qubits each connected via short couplers (like on a multi-chip module). Research from USTC even has an approach to connect two chips with galvanic coupling through a common bus resonator. Google could similarly do an “integrated multichip module” where chips sit on an interposer that provides resonant coupling between them. There’s no evidence they’ve done that yet, but it’s a logical next step if they want to surpass ~200 qubits. So far, Google prioritized improving qubit quality over raw number, which was wise to reach below-threshold errors. Now that threshold is reached, scaling up qubit count becomes the priority to get more logical qubits. They have the benefit that each added qubit is high-quality. But also, as count grows, issues like inhomogeneity (slight variations requiring unique frequency tuning for each qubit to avoid collisions) become harder – but they managed 105, which likely required carefully choosing each qubit frequency to avoid spectral crowding. At 1000 qubits, frequency crowding is a serious challenge (only so much spectrum in 4-8 GHz band). They may incorporate more sophisticated frequency allocation (maybe using more frequency spacing and sacrificing some couplings, or adding more tunability such as using tunable couplers more extensively to relieve frequency collisions). The heavy-hex approach was IBM’s solution to that; Google might adopt partial tunability or adjust lattice. It’s notable that USTC’s device being similar to Google’s and having succeeded suggests that fundamental scaling to ~100 qubits is okay. But the next leap might need architectural changes.
In terms of control electronics integration: at 100 qubits, Google already uses room-temperature electronics with many coax lines. For 1000 qubits, cryo-electronics (on-chip multiplexers, or cryo FPGA controlling multiple channels) might be needed to avoid a huge heat load and complexity. Google is likely researching that (as are IBM and others).
Summing up, Google can scale to a few hundred qubits on a chip with current tech (Willow’s proof), but going beyond, they will need either bigger chips or multi-chip modules and more advanced packaging. Their team is large and has the resources, so likely they have prototypes in the works for 200-250 qubit chips with similar fidelity. The integration of error correction also changes scaling demands: once physical errors are low enough, scaling up qubits primarily increases the number of logical qubits or code distance, which directly improves capability. Google’s demonstration of connecting 105 qubits in a single error-corrected code (distance-7) is a step towards using many qubits together for one logical qubit. They will scale that distance to maybe 11, 13, etc., which might use a couple hundred physical qubits for one logical qubit. That’s an internal scaling (using more qubits per code). They showed that larger codes do better, so they’ll likely try a distance-11 surface code next (needs 241 physical qubits for one logical, if using a full 2d patch). Willow had 105, so maybe the next chip might target ~225 qubits to realize a distance-11 logical qubit. Achieving that with good yield and uniformity is the next test. Given their track record, they likely will manage it (with maybe one more generation chip or combining two Willow chips).
In conclusion, Google’s superconducting platform is moderately scalable – up to a few hundred qubits on chip with engineering effort, but beyond that, multi-chip scaling or new integration techniques will be needed. They are actively researching modularization and quantum interconnects, so long-term scaling is feasible (they can leverage photonics for networking chips, ironically merging expertise from their photonic quantum supremacy as well).
IBM Heron R2
IBM has a very explicit scaling roadmap. Heron R2 (156 qubits) is part of their modular quantum architecture plan. IBM already built a 433-qubit chip (Osprey) and plans a 1121-qubit chip (Condor) by 2024/2025, although those larger chips might not have as high fidelity as Heron (they might be more experimental initially).
IBM’s key strategy for scaling is modularity: they introduced Quantum System Two, which can house multiple cryogenic modules connected by controlled couplings or even photonic links. In IBM’s view, scaling to millions of qubits will require linking many smaller clusters of qubits – they project by 2030s to have circuit sizes of order 1 million in their vision. In the near term, IBM’s approach is to scale by tiling Heron chips. They mention that Heron r2 “is the base of Flamingo” which “will associate multiple of these processors with a microwave link connecting them.” This implies the next generation (Flamingo) will be a multi-chip module (perhaps 3 Heron chips in one system). Indeed, IBM showcased a 3-chip arrangement in a recent demo: a 3x Heron system, likely entailing a 3×156 ≈ 468-qubit combined system (if fully connected). The heavy-hex lattice is particularly conducive to a tiling approach: one can imagine each Heron chip is a tile, and between chips, there are couplers linking boundary qubits. Because each qubit has at most 3 neighbors, a boundary qubit can dedicate one neighbor link to a qubit on another chip without increasing its degree beyond 3.
IBM has also been developing quantum communication techniques – e.g., they have a partnership with University of Chicago on cavity-mediated long-range gates. Possibly Flamingo uses cavity buses to connect chips in the same fridge, or coax lines. By 2025+, IBM plans the 1121-qubit Condor which might still be a single chip (maybe using a huge wafer or new wiring tech), but more likely that will be broken into smaller sublattices if needed. IBM further discusses linking multiple 1121-qubit units via optical fiber for distributed quantum computing by 2030 (quantum-centric supercomputing vision). So IBM’s scaling path is a combination of larger chips and linking chips.
They have already confronted integration challenges: their packaging uses multilayer interposers to bring ~1000 connections into the chip, and they’ve done thermal management for 400+ coax lines in a fridge (for Osprey). They have also pioneered cryo signal multiplexing to reduce line count. The heavy-hex structure allows using the same frequency for multiple qubits more easily, reducing the frequency crowding problem because fewer qubits are directly coupled (less all-to-all spectral constraints). So they can scale qubits without running out of distinct frequencies as quickly – that’s a subtle but key integration advantage for scaling.
IBM also invests in fabrication improvements – e.g., using 3D integration (through-silicon vias to get signals to qubits from the back side of the chip), which eliminates the need for wire bonds that limit geometry. They have shown the ability to place many qubits and couplers with good yield (127 on Eagle, 433 on Osprey albeit Osprey’s fidelity data is not fully known yet). Osprey scaling from Eagle was done by expanding to a larger chip with more multiplexed readout lines, and Condor presumably by further expansion and 3D wiring. But after Condor, they move to modular – because going monolithic beyond ~1000 might be impractical. That’s why Heron’s modular approach is crucial: it sets up a blueprint for connecting multiple chips. Indeed, they reported demonstrating entangling gates across two chips (with new coupler tech). Achieving high-fidelity inter-chip gates will be a key milestone (likely requiring synchronization and possibly new calibration for those cross-chip links). IBM’s advantage is they foresee combining this with their strong classical compute integration (fast feedback, etc.), so a modular IBM system might act like one big quantum computer to the user. For instance, you could have 4 Heron chips linked, each performing part of a surface code for a larger logical qubit, etc.
In terms of space, IBM System Two’s cryostat is designed big enough to house multiple chips and the interconnects. They use a 1 m wide hexagonal dilution refrigerator that can mount multiple chip modules inside. So physically, they are ready to host thousands of qubits by clustering. So IBM’s scalability outlook is: 1000+ qubit single modules by mid-2020s (Condor), then connecting multiple modules to reach 10k-100k scale by late-2020s to 2030. IBM’s focus on error correction also means they’ll likely not scale to millions of physical qubits without significant error reduction enabling easier error correction – but their plan is to use those thousands of physical qubits to demonstrate logical qubits and a logical quantum advantage. If error rates keep dropping, they won’t need exorbitant numbers of physical qubits to solve some problems. But ultimately, large-scale useful quantum computing still implies thousands of logical qubits, which in surface code could be millions of physical qubits. IBM’s bet is that modular architecture plus continuing fidelity improvements will allow assembling those millions from manageably sized pieces. The heavy-hex connectivity is especially nice for tiling: IBM can fabricate e.g. a wafer with multiple 156-qubit tiles and then cut and assemble them with couplers bridging them – quite akin to how multi-core classical processors link multiple dies. They already basically did a 3-Heron assembly. The next might be a 6-Heron assembly to get ~936 qubits.
On integration: IBM’s long experience in packaging (through-silicon vias, bump bonding, cryo-cables) is a strength – they consistently demonstrate complex integration steps a year or two ahead of others (like first to break 100 qubit barrier, first to use large-scale 3D integration in quantum). Therefore, IBM’s approach is highly scalable engineering-wise, albeit needing a cluster approach beyond ~1000 qubits.
USTC Zuchongzhi 3.0
The Chinese team’s approach to scaling is similar to Google/IBM (since it’s the same technology), with a strong focus on fabrication and 3D integration improvements. Zuchongzhi 3.0’s main scaling innovation was the flip-chip 2-chip stack to integrate more qubits and couplers without performance loss. This technique allowed them to put 105 qubits (with 182 couplers) in a fairly dense lattice while still controlling them effectively. They will likely continue using flip-chip for any larger devices. The head of the group, Pan Jianwei, has indicated in press that they plan to continue increasing qubit numbers and perhaps also implement error correction soon. China has a national quantum plan, so presumably scaling to 200-300 qubits is on their roadmap. We might expect a Zuchongzhi 4.0 with ~200+ qubits if they solve some technical issues (Zuchongzhi 2.0 had 66 qubits, 3.0 jumped to 105, maybe 4.0 could aim 200-300). They might also experiment with different topologies; so far they used a rectangular grid (15×7 with some qubits unused to avoid bad ones presumably). Possibly they could try a larger die with 20×10 ~200 qubits if yields allow. Using flip-chip, they could also integrate even more couplers or control circuitry. They mention co-design of multiplexed readout etc., which indicates they will incorporate techniques to manage wiring as they scale. Another aspect is that USTC could also attempt multichip linking. Pan’s group historically does photonic quantum communication, so they have expertise in linking systems. They might attempt an optical fiber link between two dilution fridges to entangle two chips – though that’s more long-term and not needed until each chip saturates what a fridge can hold (which at 100 qubits it hasn’t yet). For near term, focusing on monolithic scaling plus error correction on chip is likely. They also indicated interest in demonstrating more complex algorithms (like solving linear equations or simulating quantum dynamics) on their next-gen hardware, which might require more qubits or at least using the existing ones in a clever way with mid-circuit measurements (which needs QEC or feedback infrastructure). The integration challenge for USTC is that they are catching up on the control electronics and software – historically, their focus was on achieving one-off experiments (like sampling tasks). To scale to a general purpose machine, they need better frameworks (compilers, calibration automation, etc.). They are working on that (the quantum computing report suggests they have a Global Quantum Initiative portal etc. for more data). In terms of hardware, they have shown they can replicate Google’s achievements – meaning they have built the competency to scale fabrications. They collaborate with the Institute of Microelectronics for chip fab; having done 66 and 105 qubit chips suggests they have a solid process. If they solve uniformity and materials further (maybe adopting some of IBM’s techniques like tantalum metal, which they did), they can keep raising qubit count. It’s unclear if they have a formal plan to hit X qubits by year Y, but competitive pressures will likely push them: if IBM goes 433 and 1121, and Google maybe goes 200+, USTC will aim to not fall behind. So possibly a 200+ qubit chip from USTC could come out in a year or two. They will also likely implement quantum error correction with those qubits – which might limit effective scaling for a while (using more qubits for logical qubits rather than raw count for raw tasks). The Chinese quantum community also has separate groups working on multi-node quantum networks (e.g., entangling distant superconducting qubits via microwave-to-optical converters). So down the line, they could connect multiple dilution fridges too. The approach is similar to IBM’s vision of a quantum network of processors. But to get to thousands or millions of qubits, everyone including USTC will need to confront wiring and heat: this means cryo-CMOS multiplexers, optical I/O for high bandwidth at low heat, etc. We haven’t heard of Chinese efforts on cryo-CMOS, but given their emphasis on vertical integration, they may be developing their own cryogenic control chips. Another aspect is funding: China is investing heavily in quantum, which bodes well for them being able to attempt costly large-scale integration projects.
In summary, Zuchongzhi’s architecture is as scalable as Google’s since it’s nearly the same – planar transmons improved by flip-chip and materials. They will scale by gradually increasing chip size and complexity, while keeping errors in check via those fabrication improvements. They already note that improvements in coherence and reducing correlated errors are needed as they scale – and they have implemented measures for those. The flip-chip technique is one of their key contributions to scaling superconducting qubits and likely will be used in any next generation devices (e.g., adding a third chip layer for even more complex signal routing if necessary). They also innovated in reset schemes to handle thermal noise with multiple rounds of measurement – which suggests they are thinking about scale issues like residual excited populations (a known issue that grows with number of qubits, as more qubits = more chance one is thermally excited at start). They actively suppressed that with a triple measurement and feedback scheme before running circuits. This kind of system-level improvement shows they are preparing for making the machine operate reliably as it scales.
Summary of Scalability and Integration
Cat qubits (AWS): Modular small logical qubit units, massively replicated. They reduce overhead per logical qubit drastically, making scaling to useful quantum computing more reachable (100k physical for something useful vs millions). Scalable manufacturing via microelectronics processes is a plus.
Topological qubits (Microsoft): Monolithic integration of many qubits using semiconductor tech. If realized, it could jump to extremely large qubit counts (millions) on a chip without needing complex error correction for most errors. The challenge is more in materials and yield, which they are tackling with specialized material science.
Superconducting transmons (Google/IBM/USTC): Incrementally larger chips plus modular interconnects. They rely on improved packaging (3D integration) and eventually networking multiple chips. IBM is at the forefront of modularity (linking chips), Google and USTC focused on single-chip fidelity first but will likely adopt modular strategies soon. This approach is proven up to ~100s of qubits now and expected to reach ~1000 qubits per module within a couple years (IBM’s roadmap). For going beyond, all plan to use quantum inter-chip links (IBM explicitly, Google likely with their photonic know-how, USTC possibly with their quantum communication background).
Integration with classical: All approaches will require more sophisticated cryo integration as qubit count grows. IBM and Google are working on cryo-electronics (IBM with Zurich’s team on amplifiers, Google with Horton et al. on cryo processors). Microsoft’s approach reduces analog microwave lines (since their qubits are controlled by DC gate voltages largely), which could make classical integration simpler at scale – a subtle scalability advantage that often isn’t highlighted: topological qubits might be more wire-efficient, because you don’t need an AWG channel generating GHz pulses for each qubit; instead you might set a stable voltage or slowly ramp some global parameter for braiding, and use a few microwave readout channels. If so, controlling a million Majorana qubits could be easier than a million transmons from a wiring perspective.
Error rate vs scale: There’s also the notion of fault-tolerant threshold providing a scalability gateway – once below threshold, you can scale logical qubits arbitrarily by adding more physical qubits with manageable overhead. Google and IBM crossing threshold means they can scale logical qubit count by adding physical qubits linearly (with overhead of surface code). AWS/Microsoft avoid high overhead, so once they have enough qubits to perform meaningful tasks, scaling further mainly increases capacity or solves bigger problems directly. So from a computational scalability view, all five approaches either have reached or aim to soon reach the point where adding more qubits translates to exponential computational gain (through QEC). For example, IBM at 1000 physical qubits (if all quality high) could make maybe ~10 logical qubits; at 1,000,000 physical (1000x), they could in theory get ~10, maybe 100 logical qubits depending on code and overhead – enough for some interesting algorithms. AWS/Microsoft might get away with needing only 100k or so physical for that many logical. Google/USTC might be similar to IBM in needs.
Practical assembly: Ultimately, building a quantum computer with millions of qubits will be an enormous engineering project akin to building a new supercomputer or chip fab line. IBM and Microsoft (and maybe Google) have the industrial scale infrastructure to attempt that. China likely will devote large lab efforts or new institutes for that as well. The approaches like Ocelot and Majorana aim to cut down the scale of that project significantly by solving error correction elegantly, which is why they’re exciting even if they’re behind in qubit count currently.
Computational Capabilities and Use Cases
Each of these cutting-edge quantum processors is not just an experimental novelty; they are being developed with certain target applications or demonstrations of practical capability in mind. We will discuss what types of problems or use cases each chip is best suited for (either currently or in the future), and any practical applications or world-first demonstrations they have achieved or are aiming for.
AWS Ocelot
In its current form, Ocelot is essentially a demonstration of a fault-tolerant quantum memory – one logical qubit that can store quantum information longer and more reliably than a physical qubit can. This is a foundational capability rather than an application by itself. However, it is the building block for scalable fault-tolerant quantum computing, which in turn enables all the famous quantum algorithms. So, the significance of Ocelot is that it brings practical, error-corrected qubits closer. In terms of near-term use cases, a single logical qubit could be used to test algorithms that need quantum memory (e.g., phase estimation on one qubit, or a small quantum repeater node for quantum networking experiments). But the real goal is to scale to many logical qubits and tackle big problems: AWS explicitly mentions “solving problems of commercial and scientific importance beyond today’s conventional computers” as the mission. These include things like faster drug discovery, new materials design, optimization problems, cryptography, etc. With Ocelot, AWS is aiming squarely at fault-tolerant quantum computation – meaning they want to build a machine that can run deep algorithms like Shor’s factoring or Grover’s search on large databases, or simulate complex chemical systems accurately, all of which require long circuit depths (hence error correction). By reducing the overhead, they plan to reach such capabilities sooner. Concretely, once AWS can produce, say, ~50 logical qubits with Ocelot-like architecture, they could attempt algorithms like:
Quantum Simulation: simulate molecules to find new catalysts or drugs. For example, simulating the reaction mechanism of a complex enzyme or a high-temperature superconductor model – tasks classical computers struggle with. Fault-tolerance is needed to reach chemical accuracy for molecules beyond ~50 electrons. Ocelot’s approach could eventually allow that with fewer physical qubits.
Cryptanalysis: running Shor’s algorithm to factor large numbers (RSA keys). This famously requires thousands of logical qubits and billions of operations. AWS’s error reduction directly addresses the need for fewer qubits, making this goal (still far off, but eventually) more attainable. If Ocelot’s scheme scales, maybe factoring a 2048-bit RSA number might need on the order of 100k physical cat qubits instead of a million-plus transmons, within reach of a future AWS quantum data center.
Optimization: While NISQ devices attempt optimization via quantum approximate optimization algorithm (QAOA) or quantum annealing, these often run into error depth issues. A fault-tolerant quantum computer could run Grover’s algorithm or more sophisticated optimization algorithms to achieve a quadratic speedup in search or solve certain NP-hard optimization heuristics with better performance. With Ocelot-type logical qubits, one could run deeper QAOA circuits reliably or implement amplitude amplification for better sampling. AWS likely has customers interested in e.g. supply chain optimization, portfolio optimization, machine learning improvements, etc., which a robust quantum computer could address by accelerating combinatorial optimization tasks or high-dimensional sampling.
Quantum AI/Linear Algebra: AWS might integrate quantum with their classical cloud for ML tasks. A fault-tolerant QC could implement algorithms like HHL (quantum linear system solving) or quantum PCA which could potentially give exponential speedups in analyzing data structures. But those require stable circuits with many qubits and gates – again in the domain that Ocelot’s approach is targeting. So future Ocelot-based systems could be used for large-scale linear algebra problems that appear in machine learning (like solving huge linear systems faster than classical).
AWS’s strategy is to be the provider of quantum computing via their cloud (Amazon Braket). So a key use case is making these advanced error-corrected quantum capabilities available as a service for enterprises and researchers. Ocelot is a step toward that: a “logical qubit API” so to speak. We can imagine an AWS service in the future where users allocate some number of logical qubits and run algorithms without worrying about underlying errors – Ocelot is enabling that model by drastically reducing the qubit count needed. So in summary, Ocelot is best suited for enabling fault-tolerant algorithms early. It doesn’t solve a real-world problem by itself yet, but it’s a crucial enabler. The likely first practical demonstration AWS might do with Ocelot’s descendants is show a logical qubit memory outperform any physical qubit (which presumably they have or will do, similar to Google’s break-even but in a different way). Then, perhaps demonstrate a simple logical gate or two logical qubits performing a small algorithm (like a logical CNOT and maybe a 2-qubit algorithm like entanglement distillation). The ultimate use cases – breaking encryption, designing materials, etc. – are on the horizon once they scale it up. AWS often mentions “accelerating timeline by 5 years” – implying they believe with this tech they could hit those major applications in, say, early 2030s instead of late 2030s.
Microsoft Majorana-1
Microsoft’s quantum program explicitly markets itself as aiming for “industrial-scale” quantum computing to solve meaningful problems in years, not decades. The Majorana-based chip, if it scales as hoped, would be capable of integrating millions of qubits which could potentially handle some of the most demanding quantum algorithms. The key envisioned use cases include:
Cryptography and Security: With a million topological qubits, one could run Shor’s algorithm on large keys fairly directly (especially since overhead for error correction is low). Microsoft mentions cracking cryptographic codes as one of the goals once enough qubits are available. This ties into the need for post-quantum cryptography; ironically, Microsoft also researches cryptographic algorithms, but if they succeed in Majorana quantum computers, they might actually break current cryptosystems (RSA, ECC) in the future. One can think of Majorana qubits as paving the way to a CRQC (cryptographically relevant quantum computer) sooner.
Chemistry and Materials: Designing new drugs, catalysts, and materials is often cited by Microsoft. They talk about fighting pollution, developing new medicines, predicting material properties. For example, simulating nitrogen fixation (to design better fertilizers) or carbon capture chemistry might require simulating molecules like FeMoco (a large molecule) which is beyond classical simulation. A quantum computer with thousands of stable qubits could potentially simulate such molecules exactly, leading to breakthroughs in clean energy or climate tech. Similarly, designing high-temperature superconductors or novel battery materials by simulating quantum phases of matter is another dream application.
Scaling existing quantum solutions: There are quantum algorithms for optimization (like quadratic unconstrained binary optimization solved by QAOA), machine learning (quantum support vector machines, etc.), and others that currently can’t run at useful scale on NISQ hardware. If Microsoft’s approach yields a machine with, say, 1000 nearly error-free qubits, they could tackle larger instances of these problems directly. For instance, solving a large optimization problem (like optimizing traffic flow in a city or supply chain logistics for a global company) might become feasible. They have mentioned solving “meaningful” problems for customers in the nearer term as a goal.
Microsoft often emphasizes full-stack development: they are preparing software (Azure Quantum, Q# language) that will interface with the hardware when it’s ready. This means they likely have some specific algorithms in mind that they want to run on their machine. They published a paper in 2023 on quantum algorithms for chemistry and materials (with estimates of required qubits) – such algorithms (like quantum phase estimation for molecular energies) are high on their list.
One unique capability of topological qubits is reliability – if each qubit is stable for long times, very deep circuits can be executed. That opens up algorithms like quantum error correction of logical qubits themselves to an even higher level (concatenation for extremely low error) or long simulation circuits that iterate many times. For example, some quantum algorithms require iterative processing (like quantum linear system solvers with many iterations); these would benefit from the long coherence.
In terms of demonstrated applications so far, Majorana-1 is still at the physics demonstration stage. They haven’t solved any algorithmic problem with it yet, since they just confirmed the qubit existence. The next steps might be to show a topologically protected qubit operation (like a braid resulting in a deterministic phase – which is like a robust gate) and then potentially demonstrate a simple algorithm like a 2-qubit Deutsch-Jozsa or Grover search on a small database but done in a fault-tolerant way. Those would be toy problems but would show the viability of quantum logic operations with the new qubits.
Looking ahead, if Majorana qubits scale to many, Microsoft could leap directly to solving things that others would need error correction for. For example, factoring a 256-bit number might require maybe 1000 Majorana qubits with some overhead (if T-gate injection needed, maybe a few thousand). That’s far fewer than the millions of physical transmons a competitor might need. So one potential first real application of a Majorana-based quantum computer could indeed be factoring a large number or computing a discrete logarithm, essentially breaking a specific instance of RSA or ECC. This would be a headline-grabbing achievement with practical significance (demonstrating the need to switch to post-quantum cryptography).
Another near-term smaller application: quantum sensing / topological qubit memory. Because Majorana qubits are stable, one could imagine using a single Majorana qubit as a memory to store a quantum state (like from a sensor or another quantum system) for an extended period and then retrieve it. That’s more on the quantum networking side – like a quantum repeater memory element. Microsoft’s tech could contribute there: a Majorana qubit could hold entanglement until needed, which is useful for long-distance entangled network (though their current readout is local, with some integration it could connect to photonic links).
In summary, Majorana-based quantum computers are pitched for the hardest computational problems: breaking cryptography, accurately modeling complex molecules and materials, and solving large-scale optimization or linear algebra problems that are far beyond classical reach. Microsoft explicitly says a topological quantum computer of sufficient size “will be able to solve meaningful, industrial-scale problems,” which includes things like improved fertilizer production (addressing world hunger), discovering new drugs faster (healthcare), and perhaps even machine learning acceleration (imagine running quantum algorithms to speed up training of AI models). Their emphasis on “years, not decades” is bold – they are essentially suggesting they could reach some of these in, say, <10 years. If their approach works out fully, that might be within reason. But as of now, Majorana-1’s demonstrated use case is limited to being a stable qubit – the next year or two will reveal more as they publish results on multi-qubit operations.
Google Willow
Google’s use-case focus has been two-fold: showing quantum advantage (which they did with random sampling) and pushing towards a “useful beyond-classical computation” relevant to real-world problems. They openly stated the next challenge is to demonstrate a beyond-classical computation that actually has practical relevance, e.g., in materials science or machine learning, using the Willow-generation chips.
Currently, Google has used their chips for:
Quantum supremacy experiments: This was not useful per se, but proved a point.
Quantum error correction experiments: This is a stepping stone to future algorithms, showing they can preserve info longer.
Physics simulations: Google has done experiments like simulating a discrete time crystal on a quantum processor (with 20 qubits on Sycamore) – this is a physics application (studying a non-equilibrium phase of matter). With Willow’s longer coherence and more qubits, they could simulate larger quantum systems or observe new phenomena in quantum dynamics that classical simulation cannot. For example, they might simulate quantum chaos in a 100-qubit spin system or do a larger quantum chemistry calculation than before.
Basic algorithms: They have implemented small instances of algorithms like Grover’s search and QAOA on prior chips (mostly as demos). With Willow, they could run these on more qubits or at greater depth. For instance, they might attempt QAOA for a max-cut problem on a graph large enough that classical algorithms struggle, to see if any advantage emerges. Or run a version of the HHL algorithm to solve a linear system that’s just beyond a classical brute force. These would be baby steps toward useful quantum computing, and might not beat classical methods yet, but they set the stage.
Google’s ultimate targets are similar to others:
Quantum chemistry: Pichai (Google’s CEO) has mentioned possible breakthroughs like developing new fertilizers or optimizing batteries as motivators for quantum computing. Google AI and Google Research have teams working on quantum simulation algorithms for chemistry. We might see Google use Willow or its successors to simulate a molecule like diazene or ethylene with full configuration interaction, something only small classical clusters can do now. Eventually, they’d aim for something like FeMoco (nitrogenase’s active site) or a complex reaction mechanism.
Machine learning: Google has the TensorFlow Quantum team exploring hybrid quantum-classical ML. A use case could be using a quantum computer to accelerate parts of an ML pipeline, e.g., using quantum kernels for classification on a dataset that is hard for classical kernels, or using quantum sampling to help train a Boltzmann machine. If they find an advantage, they could incorporate that into Google’s AI offerings (e.g., quantum-assisted recommendation systems or quantum generative models). This is longer-term, but Google’s dual strength in AI and QC means they are well-positioned to try quantum machine learning applications when hardware allows.
Optimization/Finance: While Google hasn’t targeted finance as explicitly as IBM (who works with banks for quantum risk analysis), these are general algorithms any QC can do. Google could demonstrate, for example, a proof-of-concept portfolio optimization with QAOA beyond 5 qubits, or a scheduling optimization (like their own server or traffic routing optimization tasks) using a quantum approach.
Materials and physics: Google might use their QC to simulate condensed matter models – e.g., exploring high-temperature superconductivity by simulating the Fermi-Hubbard model on a lattice of qubits. They published a Science paper simulating a simplified version with 4×4 = 16 qubits on Sycamore. With Willow’s 100 qubits, they could simulate a larger 10×10 Hubbard lattice, which could yield insight into electron correlations relevant to superconductors. That’s cutting-edge physics research with practical implications for material design.
In terms of demonstrated practical uses so far: none that solve a previously unsolved real-world problem. But Google has indicated some milestone goals:
Quantum advantage in a useful task: maybe demonstrating that for a particular problem (like sampling from a quantum distribution relevant to a chemistry problem or solving a small instance of a combinatorial optimization) the quantum does better than classical heuristics. If they achieve that, it would be a huge validation for real-world applicability. They are actively chasing this “useful quantum supremacy.”
Google’s partnership with Volkswagen in the past looked at traffic flow optimization on a D-Wave annealer (which wasn’t clearly better, but they experimented). With a gate QC like Willow, they might revisit such logistic optimization in a different algorithmic way.
Another interesting use case is quantum error-corrected memory as service: If Google perfects logical qubits, they could conceive offering secure quantum memory or verification services (like verifiable random number generation which they already did experiments on with a quantum chip generating certifiable randomness, which is useful in cryptography) – that was one of the outcomes of the random sampling experiment (it generated random bits with a certificate of fidelity).
For now, the most practical thing Google has done is generate those certified random bits (which some argue could be used for cryptographic keys, but given the sampling time and overhead, it’s not commercial yet). They also collaborated with others to use quantum computing for specific scientific computations (like simulating chemical kinetics of a reaction).
In summary, Google’s Willow is best suited for near-term tasks in quantum simulation and for demonstrating the path to fault-tolerance. They likely will use it or its next iterations for:
- Probing quantum physics (quantum phase transitions, dynamics)
- Small-scale quantum algorithm demos (to validate algorithms in practice, such as variational algorithms for small problems)
- Paving the way for error-corrected algorithms (they’ve already shown logical qubits, next is to perform a logical operation or an algorithm on a logical qubit).
Once they have a handful of logical qubits, likely use cases will be precision simulation (e.g., calculating a molecular energy to chemical accuracy, which is something classical can’t do beyond small molecules). That could be a first useful demonstration, impacting chemistry and materials science communities by providing a result they couldn’t get otherwise. Google has a partnership with Fermilab on quantum simulations for physics, so maybe simulating a small lattice gauge theory (relevant to particle physics) could also be a target – which would be a scientific contribution outside of computing itself.
IBM Heron R2
IBM’s approach to use cases is very broad and user-driven, thanks to their IBM Quantum Network of partners (research labs, businesses exploring quantum solutions). They have actively been pursuing use cases in chemistry, finance, and optimization/AI with their early hardware via quantum experience and Qiskit Runtime.
Currently, IBM has had some success in:
Quantum chemistry: IBM demonstrated the largest quantum simulation of a molecule’s ground state energy using 127 qubits (though with heavy error mitigation) – they simulated part of a spin model that mimics a molecule’s behavior (they call it the “utility” experiment which was related to battery chemistry). They have worked with Daimler on quantum chemistry for batteries, and with JSR on quantum chemistry for materials. While these are still exploratory, IBM aims to eventually integrate quantum computing into the workflow of chemical discovery. Heron R2’s ability to run 5,000 gate circuits means they can do more accurate simulations or deeper variational ansätze than before, which could yield better results for these clients.
Finance: IBM collaborates with financial institutions like JPMorgan and Mitsubishi UFJ to explore option pricing and risk analysis using quantum algorithms (like quantum amplitude estimation for Monte Carlo simulation). A small speedup was demonstrated by IBM in option pricing by doing amplitude estimation with error mitigation on a 7-qubit system vs classical Monte Carlo. In the future, with an error-corrected quantum computer, amplitude estimation can give quadratic speedups in Monte Carlo (important for risk calculations, portfolio optimization, etc.). So IBM sees financial risk analysis, portfolio optimization, derivative pricing as key use cases. They have done prototypes on current hardware (like a 2-qubit proof-of-concept of quadratic speedup in derivative pricing).
Supply Chain and Optimization: IBM has worked on using QAOA for scheduling problems (e.g., optimizing shipyard operations with Mitsubishi Heavy Industries, or telecom network optimization with Vodafone). Their approach often uses current machines to solve small instances and compare to classical, to learn how to improve algorithms. Once they have bigger machines (like Heron R2 and beyond), they can tackle larger instances. They foresee that with 1000+ high-quality qubits, QAOA or other algorithms might reach regimes where classical methods struggle (especially if combined with clever classical post-processing).
Machine Learning: IBM has explored quantum kernels and variational classifiers. They have an eye on quantum natural language processing and other novel ML methods (one of their researchers created QNLP algorithms tested on small hardware). With more qubits, they could embed larger datasets in Hilbert space for classification tasks or do feature mapping that classical kernels can’t easily replicate.
Scientific computing: IBM collaborates with national labs on simulating physics. For example, simulating lattice models in nuclear physics or simulating turbulence (applying quantum algorithms to differential equations) are topics they look at. They also used a previous 65-qubit device to simulate a simple quantum field theory (Z2 gauge theory on small lattice). With 156 qubits, they could simulate a larger lattice or more complex model.
Quantum-assisted HPC: IBM’s vision of quantum-centric supercomputing means integrating quantum processors as accelerators in classical HPC workflows. A concrete scenario: using a quantum module to compute something like a partition function or a matrix element that’s hard for classical, within a larger simulation. This might apply in climate modeling or materials design HPC codes.
IBM’s first likely practical application might come in the form of quantum advantage in a specific industry problem. They are attempting things like:
- Proving a quantum advantage in option pricing or financial risk calculation. For instance, if they can do a high-dimensional Monte Carlo faster with a quantum routine on an error-corrected machine than the best classical Monte Carlo on a supercomputer, that would be a clear application (banks could price complex derivatives faster or more accurately).
- Accurately simulating a medium-sized molecule or material – something like the reaction rate of a chemical catalyst, which chemists can’t get from classical simulation alone. Achieving chemical accuracy in such simulation would directly impact chemical engineering.
- Optimizing a real-world system – like optimizing train scheduling for a national railway or supply chain routing for a global retailer better than classical operations research methods. If they do that, it could save real money and be a commercial success. This likely needs error-corrected qubits for a substantial boost, but perhaps a mid-term scenario is hybrid algorithms that produce good approximate solutions with quantum help.
IBM has been preparing clients via their Quantum Accelerator program to be ready to use quantum solutions as soon as they outperform classical. They often mention “quantum advantage applications” expected in the 2020s in areas like drug discovery and financial risk. So they clearly target those fields.
Meanwhile, IBM also has done fun use cases like quantum music generation or quantum games (for outreach), but those are not practical uses, just educational.
Currently, IBM has 5,000+ gate fidelity circuits meaning they can start tackling deeper circuits required by algorithms like amplitude estimation (which need deep circuits). They recently showed a 50x speedup in running those circuits, meaning through CLOPS improvements and fidelity, they basically can do in a couple hours what used to take days on earlier hardware. This suggests that in the near term, IBM might be able to perform something like a 100-iteration amplitude estimation for a finance problem – which would be a small quantum advantage demonstration if they manage to surpass classical sampling error at same compute time.
IBM also emphasizes error mitigation as a way to get useful results earlier. They recently used error mitigation to execute a 127-qubit, 60-layer circuit to estimate a physical quantity with some success. That is sort of a useful computation (if that quantity was something needed in a simulation).
So IBM’s plan is a continuum: use error mitigation on present hardware to do as much as possible in chemistry/materials/finance optimization, then transition to error-corrected hardware (like late 2020s) to unlock full solutions.
The key use cases remain:
- Chemistry (new catalysts, materials for batteries or semiconductors)
- Finance (risk, optimization, fraud detection maybe via ML)
- Supply chain/logistics (big optimization problems, e.g., route optimization for delivery, manufacturing process optimization)
- AI/ML (less emphasized by IBM compared to Google, but they do explore e.g. quantum generative models for data).
IBM’s broad approach with partnerships means we might see one of their partners announce a quantum-enhanced result in their domain as one of the first real-world applications. For example, maybe an IBM partner in automotive materials finds a new lightweight alloy by quantum simulation guidance, or a bank finds a portfolio strategy slightly better via quantum risk analysis. These might be incremental improvements at first, but even a small advantage in finance or materials can be valuable.
USTC Zuchongzhi 3.0
The primary focus so far has been fundamental demonstrations (quantum advantage in random sampling). However, China’s quantum program certainly eyes real-world impacts as well, especially in:
Cryptography: China is very interested in quantum communication and security (they have a quantum satellite, QKD networks, etc.) A powerful quantum computer (like Zuchongzhi’s future iterations) could break conventional encryption – a reason they invest in both quantum computers and post-quantum cryptography. So, like others, factoring and discrete log are eventual use cases. Chinese researchers have optimized algorithms like factoring; they’d surely attempt them if hardware permits.
Pharmaceuticals and Materials: China has a large pharmaceutical and materials industry, and a quantum computer could aid in discovering new drugs or better materials (like catalysts for green energy, which is a national priority for carbon peaking goals). USTC likely will apply quantum algorithms to simulating molecular structures or reaction dynamics in conjunction with their chemists. They have strong chemistry and physics departments possibly collaborating. For instance, simulating complex chemical reactions relevant to industrial chemistry (ammonia synthesis, CO2 reduction) could be an application.
AI: The Chinese government has a big initiative in AI. Quantum machine learning might appeal if it can give an edge. Some Chinese groups work on quantum neural networks and such. If Zuchongzhi improves, they might try to run quantum neural network models (like quantum Boltzmann machines or quantum sequence modeling). But this is still academic; no clear advantage has been shown.
Optimization: China has huge logistics networks (for example, their delivery and manufacturing networks). A quantum computer could be applied to optimize factory scheduling, traffic routing, or power grid management. Chinese academia (like CAS and others) do research on quantum optimization algorithms as well. Possibly they will test these on their hardware for specific government relevant problems (like optimizing high-speed rail schedules or something).
Scientific research: Simulating condensed matter physics (like high Tc superconductors or frustrated magnets) might be a scientific goal since China invests in physics research heavily. If their QC can simulate a model that yields insight into a phenomenon (like why a certain material superconducts at X temperature), that would be a scientific breakthrough with eventual tech implications (designing new superconductors).
Right now, Zuchongzhi’s known “application” was doing a task (random sampling) extremely beyond classical reach – which, interestingly, has been pitched as a way to generate certified random numbers (just like Google’s output). In a Chinese context, ultra-high-quality random numbers could be used for national security (e.g., one-time pads, but you’d need them to trust the generation method). So maybe they consider using their quantum computer’s random circuit outputs as a random source (though they have QKD, etc., so they have other random sources).
They published a paper where they describe the million-sample RCS as “firmly establishing a new benchmark in quantum computational advantage” and mention it opens the door to investigating “circuit complexity in solving real-world problems”. That hints that now that they achieved this technical milestone, they want to pivot to complexity of “real-world” circuits, meaning more structured tasks.
With 105 qubits, they could try a small chemistry simulation like Google did. Perhaps they will, to not fall behind in that area.
Also, China’s national projects likely encourage using quantum computing for things like drug design (especially new antibiotics or antivirals) – which is strategic – or new materials for manufacturing and defense.
They also invest in quantum AI institutes (e.g., Baidu has a quantum computing division focusing on quantum ML). Possibly, Chinese tech companies like Baidu or Alibaba might collaborate to use Zuchongzhi prototypes for tasks like recommendation system optimization or data analysis to show a “quantum advantage in AI”.
For now, USTC’s Zuchongzhi is a research tool. It hasn’t done a practical calculation beyond proving a point. But the capabilities it showed (lots of qubits entangled, moderate fidelity) could be steered toward a demonstration in quantum simulation soon. I suspect an upcoming milestone could be:
- Simulating a spin model (like Ising or XY model) with >60 qubits and measuring something like magnetization or phase transition that classical sim can’t easily do at that size, thereby providing new physics insight.
- Implementing an error correction code (like surface code distance 3 or 5) to show they too can do QEC. This is not an application but a necessary step to any future application – likely in near term.
- Attempting QAOA or Grover’s on a small real dataset to see if they can beat classical for that specific case.
As China also has a strong state interest in beating Western achievements, they might aim to be first in some demonstration. They already matched Google in quantum supremacy. Perhaps they will try to be first to demonstrate, e.g., a whole algorithm factoring a large number (maybe not RSA 2048, but something that surpasses prior records using a combination of classical pre/post-processing and quantum steps). If they can factor, say, a 9 or 10-digit number using Shor’s (which no one has done yet fully error-corrected), that would be a big news albeit not yet threatening RSA. It would show progression towards that goal.
Another key interest is quantum communication and networks: they might integrate their quantum computer with quantum communication lines, like teleporting a state from a photon into the superconducting qubits or vice versa. This would be a demonstration linking quantum computing with quantum communication (something the West also tries, but China leads in long-distance QKD so they might try to incorporate a quantum repeater with a small quantum computer node to correct errors in entanglement distribution).
In summary, Zuchongzhi 3.0’s future uses align with those of Google/IBM: quantum simulation of chemistry and materials, optimization for industrial processes, and cryptography. Since it’s a national lab project, they’ll focus on things with scientific or strategic impact. The next applications will likely be still within research – e.g., solving a scientific problem that was open. Then in longer term, piggybacking on that for practical outcomes (like if they simulate a material and find a new catalyst that reduces industrial energy consumption by 10%, that’s a huge practical win).
They haven’t engaged with industry as openly as IBM (no mention of Chinese companies using their machine yet, as far as I know), but that may happen behind closed doors with state-owned enterprises or so. Possibly the Chinese electric grid or a large chemical company might quietly test quantum algorithms with USTC’s team.
Summary of Computational Capabilities and Use Cases
Overall, across all five: The endgame use cases converge: breaking encryption (national security), discovering new materials/drugs (economic and societal benefit), optimization (logistics, finance, etc. – directly saving money or time), and machine learning/AI (improving algorithms that are central to technology).
The timeline is different: AWS and Microsoft have fewer qubits now but aim to get to those endgame uses by focusing on fault tolerance leaps. IBM and Google have more qubits now and are trying intermediate uses with error mitigation while building towards fault tolerance a bit more gradually.
Many of the demonstrated uses so far are scientific or proof-of-concept (no quantum computer has truly solved a commercial problem better than a classical computer yet). But within the next few years, we expect to see the first instances of quantum advantage for a useful problem – maybe a chemistry simulation giving a result not obtainable classically, or an optimization where quantum gives a better heuristic result than known classical ones. Each of these systems is poised to contribute to that:
- IBM might show advantage in an optimization or simulation via heavy error mitigation or early error correction.
- Google might do it via a careful beyond-classical simulation of a physical system or a combinatorial problem using their advantage in fidelity.
- AWS/Microsoft might do it later but more decisively via error-corrected qubits if their tech matures (like running a full Shor’s algorithm on a non-trivial number, or accurately simulating a complex chemical system).
- USTC might surprise by leveraging sheer qubit count and decent fidelity to attempt a new quantum advantage demonstration in some structured task (like quantum linear algebra).
Finally, it’s worth noting one practical spin-off: random number generation via quantum supremacy circuits (Google and USTC both mention it). That could actually be commercialized as a service (certified random bits for cryptography). It’s niche but a direct application of their current capability. It’s arguable how “practical” that is (since simpler methods exist to get random bits), but if one needs provably unpredictable numbers with minimal assumptions, quantum supremacy circuits can provide that.
In conclusion, each of the five latest chips contributes to moving quantum computing from pure demonstration to a tool for solving real problems:
- AWS Ocelot – focuses on reliability to eventually run any algorithm that requires long circuits (enabling the big breakthroughs in cryptography, chemistry, etc., on fewer qubits).
- Microsoft Majorana-1 – aims at massive scalability for similarly broad applications (with hopes of addressing some of humanity’s toughest computational problems like drug discovery and material science within a decade).
- Google Willow – currently a workhorse for exploring quantum physics and algorithm prototypes, with the near-term goal of first useful quantum advantage (likely in simulation or specialized computation), and the long-term goal of a universal fault-tolerant computer for all applications.
- IBM Heron R2 – currently powering experiments in varied fields via their ecosystem; they likely will be among the first to integrate quantum computing into real business workflows (like risk analysis or material design) in a limited but meaningful way, perhaps within a few years if their error mitigation and scale progress allow a slight edge over classical in some niche cases. Longer term, IBM clearly targets universal fault-tolerant computing for broad applications like climate modeling, global optimization, etc., delivered via cloud.
- USTC Zuchongzhi 3.0 – so far used for fundamental benchmarks, but with the backing of a national effort that will pivot to applying quantum computing to strategic problems (chemistry for energy, cryptography, advanced materials for technology and defense, etc.). In a few years, we might see Chinese quantum computers used to, say, simulate a new high-efficiency solar cell material or optimize a complex supply network, as demonstration of catching up or surpassing Western efforts in useful quantum computing.
Each chip, through its advancements, brings the community closer to the era where quantum computers move out of labs and into solving impactful problems in science, industry, and national security. The synergy of improving qubit count, coherence, and error correction bodes well for achieving those coveted practical applications in the near future.
Implications for Q-Day
The collective advancements from AWS, Microsoft, Google, IBM, and Zuchongzhi significantly strengthen the outlook for achieving quantum supremacy (or beyond-classical computing) on practical problems and hasten the approach of what cybersecurity experts call “Q-Day,” the day a quantum computer can break public-key cryptography.
Q-Day refers to when a quantum computer can break standard cryptography like RSA or ECC by running Shor’s algorithm for factoring or discrete log. To break, say, 2048-bit RSA, estimates often cite the need for on the order of 20 million noisy qubits, or perhaps a few thousand error-corrected logical qubits with long coherence.
Before these recent developments, many experts predicted a timeline of at least a decade or two for that scale – often mid-2030s or beyond. How do these announcements affect that? They certainly indicate faster progress towards more stable qubits. Microsoft’s claim of a million-qubit chip, if realized in the next 5–10 years, would be a game-changer – a million topological qubits might be able to break RSA with far fewer than that in logical qubits (since each topological qubit is like a logical qubit already). Even if Microsoft is optimistic, their success would at least spur competitors. AWS’s reduction of overhead by 10× means that instead of needing, hypothetically, 1 million physical qubits for a strong cryptographic attack, one might need only 100k (if their approach can be scaled to logical gates, which remains to be proven). Google’s threshold demonstration is more incremental but it basically proves the paradigm needed for a cryptographically relevant quantum computer works: you can scale superconducting qubits with error correction.
It’s now a matter of engineering to reach the required size. IBM’s integration and modular approach is directly aimed at scaling—IBM openly talks about million-plus qubit systems by combining modules in the coming decade (their roadmap envisions hitting 1121 qubits in 2024 with Condor, then beyond into modular systems). With all this momentum, some forecasts might shift a bit earlier. Where previously many thought a CRQC might not appear until ~2035 or later, I am now predicting early 2030s. These new developments likely increase that probability. Already, government agencies (like NIST and NSA in the US) are moving to post-quantum cryptography in anticipation of potential breakthroughs before 2030.
It’s important to stress that there is still a long way between demonstrating a handful of logical qubits and factoring a 2048-bit number. To run Shor’s algorithm for RSA-2048, one estimate is needing around 4,000 logical qubits with error rates around 10-15 and billions of gate operations. Google’s experiment showed error ~10-3 with 1 logical qubit; they’d need to get to ~10-15 which is another 12 orders of magnitude suppression – requiring more qubits and more levels of encoding or longer concatenation. However, these developments suggest the building blocks are coming together: if one company’s approach falters, another’s might succeed. For instance, if superconducting qubits can’t reach the needed scale in time, but Majorana qubits do, the outcome (a large quantum computer) still happens. Or bosonic qubits could complement superconducting to reduce overhead drastically. There’s even a scenario where these innovations combine – which the user specifically asked us to consider: What if these companies integrated each other’s best innovations?
If AWS, Microsoft, Google, IBM, and Zuchongzhi somehow pooled their advances, one could imagine a hybrid machine with, say, Microsoft’s topological qubits as the foundation (for stable memory), Google’s surface-code algorithms running on top of them to handle any residual errors, AWS’s bosonic qubits used for high-performance memories or communication links (since bosonic modes can also be used for communication in principle), and IBM’s modular and software integration linking it all together. Such a machine could, in theory, dramatically compress the timeline. For example, Microsoft’s million-qubit chip (if achieved) plus Google’s proven error correction could yield thousands of logical qubits fairly rapidly (since if each 1 of 4 Majoranas is a qubit with error 10-6, one could then surface-code correct those down to 10-15 with maybe distance ~3 or 5 since they’re so good already). IBM’s know-how in building large cryogenic systems and fast classical co-processors would be essential to control a million-qubit array (no single lab has run that many qubits; IBM’s experience with 433 and multi-chip integration would help). AWS’s bosonic qubits might be particularly useful in the readout and communication layer: for instance, cat states could be used to distribute entanglement in a error-protected way between modules (this is speculative, but cat states have been considered for quantum communication because of their error bias).
In reality, of course, the companies are competing and each approach is somewhat incompatible to directly integrate (transmons vs Majoranas vs resonators are all different hardware). But conceptually, integration of best ideas is already happening at the intellectual level. For example, IBM and Google might adopt bias-preserving gates (an idea from bosonic qubits) in their transmon setups by using multi-photon drives. Google and AWS might adopt some topological error correction ideas (like lattice surgery) that IBM pioneered in software. Microsoft might use a surface-code-like arrangement of Majorana qubits (they mention the chip is tile-able, likely meaning they will still use some planar code across Majorana qubits to correct those non-topological T errors). So cross-pollination of innovations could accelerate progress regardless of direct hardware integration. If one approach clearly outperforms, others might pivot to it as well.
In summary, these five advancements collectively move the needle forward significantly toward a cryptographically relevant quantum computer. They attack the problem from different sides: error rates down (Google/IBM,Zuchongzhi), overhead down (AWS), and qubit count up (Microsoft). If all three of those happen concurrently – even moderately – the timeline for a CRQC shortens. Where one might have said in 2020 that it’s 15–20 years away, now one might say perhaps 6-8 years, with a non-zero chance of <6 years if some bold assumptions pan out. It underscores why the world is already transitioning to post-quantum encryption. None of these companies is explicitly trying to break cryptography (their stated goals are more about useful applications in chemistry, materials, AI, etc.), but the byproduct of their success will be machines that can run Shor’s algorithm. As a precaution, governments and industry are acting as if Q-Day could come as soon as the early 2030s. The announcements from AWS, Microsoft, Google, IBM, and Zuchongzhi give more credence to those precautions – they show that the fundamental obstacles to large-scale quantum computing (stability, error correction, interconnects) are being overcome one by one, faster than many expected.
Predictions and Future Outlook
Given the rapid advancements signaled by these announcements, the next few years in quantum computing are poised to be transformative. Here I’ll provide a forecast for the timeline of quantum advantage and error correction, and how cross-industry collaborations (or the lack thereof) might shape progress.
Timeline to Quantum Advantage
We can expect incremental quantum advantage demonstrations within the next 2–3 years on specialized problems. With Google Willow’s error-corrected qubits now outperforming uncorrected ones, it’s likely that the Google team will next tackle a problem of practical interest (perhaps simulating a small chemical reaction or solving a simplified optimization problem) using their encoded qubits. This would be a stepping stone from “beyond-classical” to “commercially relevant.”
IBM, on the other hand, may leverage its 127-qubit and 433-qubit processors with advanced error mitigation to show a quantum advantage in areas like machine learning or combinatorial optimization (for example, running a variational algorithm that converges to a better solution than a classical heuristic within a given time). IBM has already hinted at quantum advantage in certain circuit simulations; extending that to a clear, application-level milestone is plausible by 2025 or 2026.
AWS’s Ocelot suggests that if they can build a few logical qubits with cat codes, those could be used for a simple algorithm (even something like a small database search or an error-corrected Grover’s algorithm) to show a logical-qubit-level advantage by mid-decade.
Microsoft’s timeline is perhaps the most uncertain: if Majorana 1 is successfully scaled to, say, tens of qubits by 2026–27, and if each of those is stable, they might demonstrate a prototype topological quantum computer solving a problem with far fewer qubits than competitors (because each qubit is inherently more powerful). However, given the past delays, a cautious outlook would put a practical demonstration from Microsoft toward the late 2020s.
Practical error correction
Practical error correction (meaning sustaining a logical qubit through many operations with low error) is now on the horizon.
Google’s result was a big proof, but to use it in algorithms, they’ll need to perform logic gates between logical qubits (entangling logical qubits, etc.). The outlook is that within ~5 years (by 2029), we’ll see a small network of, say, 3–10 logical qubits working together with full error correction. This could achieve something like a logical CNOT between two encoded qubits with higher fidelity than any physical CNOT. Achieving that will mark the true dawn of fault-tolerant computing.
IBM is likely on a similar schedule; their roadmap suggested aiming for a demonstration of a fault-tolerant quantum circuit (involving logical qubits) around 2027–2028. IBM’s modular strategy might allow them to dedicate one Heron chip to one logical qubit, another chip to a second logical qubit, and link them – showing error-corrected two-qubit gates.
AWS, if they continue the concatenated bosonic approach, might by then have a couple of Ocelot-like modules to entangle, essentially performing a logical gate between bosonic-encoded qubits. The timeline could be similar — late this decade.
Scale of devices
By 2030, I predict quantum processors will routinely have hundreds to low-thousands of physical qubits with error correction active. IBM has publicly talked about a 1121-qubit chip (Condor) in 2024, and moving into modular systems after that. It is conceivable that by 2027–2030, IBM could have a system of 10,000+ physical qubits (for instance, 10 modules of ~1k qubits each). If error rates continue to drop, that might correspond to on the order of 50–100 logical qubits (depending on the code distances used).
Google likely will also be in the few-thousand physical qubit range by 2030 if they double qubit count every ~2 years (105 in 2024, ~200-300 by 2026, ~500-1000 by 2028, etc.).
Microsoft’s ambition of a million-qubit chip might be further out (maybe in the 2030s if all goes well), but we might see smaller but still large topological chips (say 1000 topological qubits) by 2030 if their approach shows consistent progress after this initial hurdle.
AWS’s approach may not require sheer numbers of qubits as soon, but to perform computations they will need multiple logical qubits — possibly they’ll aim for a prototype logical register (say 5 logical qubits) by the end of the decade, which with their 10× overhead claim might be ~50 physical cat qubits (plus ancillas) — that could be a device with maybe ~100 physical qubits total. So ironically, AWS might achieve some fault-tolerance with fewer qubits, albeit each being more complex.
Fault tolerance by the early 2030s
The consensus in the community I am talking to, bolstered by these announcements, is that we will have demonstrable fault-tolerant quantum computing (meaning you can run a quantum algorithm for arbitrarily many steps without accumulating errors, using logical qubits) in the early 2030s. Possibly around 2030 ± 2 years, we might see the first fully error-corrected algorithm executed — something like factoring a small number (not RSA size, but maybe a 8-bit or 16-bit number with Shor’s algorithm using logical qubits, purely as a demo of fault tolerance). From there, it’s a matter of scaling logical qubit count.
The impact of cross-industry collaboration will be crucial in shaping this timeline. If these companies continue to share insights (through publications, conferences, and even direct partnerships like cloud access programs), it’s likely the field will avoid dead-ends and duplicate mistakes, accelerating progress. For instance, if Microsoft’s topological approach hits a roadblock, open discussion might help resolve it or inform others to adjust course. If, hypothetically, AWS’s bosonic qubits show a superior performance, IBM or Google could incorporate bosonic modes as a memory element in their processors (we already see some convergence: Google has experimented with bosonic error-correcting codes on their Sycamore chip in the past, and IBM is researching oscillator modes in parallel to transmons). Such hybridization could shorten the path to a large-scale quantum computer.
It’s also possible that as the stakes get higher (with glimpses of commercial advantage), companies might become a bit more guarded. However, given the complexity of the challenge, I believe collaborations will deepen: we might see, for example, partnerships where one company’s hardware runs another’s software tools. A speculative but not implausible scenario: IBM and Microsoft could collaborate where IBM’s quantum hardware is used to test Microsoft’s topological qubit error correction protocol (if Microsoft doesn’t yet have enough qubits, they might simulate aspects on IBM machines). Or AWS’s cloud might host Google’s processors for select academic users — after all, AWS already hosts other companies’ quantum devices. Such cross-use would blur competitive lines but could drive the field ahead faster.
Quantum computing in the next few years will likely also see involvement from governments and big consortiums, fostering collaboration. The US, EU, and China have large quantum initiatives that encourage academia-industry cooperation. IBM, Google, and others are part of the US National Quantum Initiative centers, where they work alongside each other and universities. These interactions ensure that even if corporate strategies differ, the scientific know-how disseminates.
To put a timeline in succinct form:
- 2025: More “proof-of-concept” demonstrations of error correction (e.g., distance-9 surface code from Google, small algorithm on logical qubits from IBM). Possibly Microsoft shows a basic braiding operation or two-qubit gate on Majorana qubits. AWS might show improved cat qubit coherence or a logical operation on two Ocelot qubits.
- 2026-2027: First instances of quantum advantage in useful problems (e.g., quantum chemistry simulation surpassing classical methods). IBM or Google could announce that they’ve run a short-depth algorithm with logical qubits that classical cannot simulate accurately. Error-corrected two-qubit gates are likely demonstrated reliably. Hardware around 1000 qubits physical becomes available on cloud with error rates ~0.1% or better.
- 2028-2030: Fault-tolerant computation achieved on a small scale. For example, running a simple algorithm like Grover’s search with logical qubits for many iterations, proving the circuit can be as long as needed. Perhaps factoring a small number with Shor’s algorithm using error-corrected qubits as a public milestone. Hardware scale: 10k+ physical qubits for IBM/Google; maybe >100 stable qubits for Microsoft if topological works; AWS demonstrating a multi-logical-qubit bosonic processor. At least one of these platforms might be executing >$$10^6$$ quantum gate operations reliably via error correction.
- 2030-2032: If all goes well, this is when one might see a cryptographically relevant demonstration (like factor a 128-bit RSA number, something beyond trivial but not yet threatening 2048-bit RSA). It might involve a combination of many logical qubits and heavy classical post-processing, but it will signal that the path to large-scale is merely one of engineering and scaling at that point.
Throughout this timeline, industry collaborations like open-source contributions, joint benchmarks, etc., can shave time. If companies were siloed and secretive, one might add a few extra years for rediscovering methods. Fortunately, the trend has been toward openness. As a result, the optimistic scenario of a major quantum breakthrough by 2030 is not out of reach. Conversely, if a promising approach fails (e.g., if Majorana qubits turn out not to be feasible), the others provide fallback options — diversity of approaches actually increases the likelihood that at least one will succeed on schedule.
Conclusion
The next few years will likely see quantum computers evolve from experimental devices to proto-commercial machines with the ability to solve some problems faster than classical supercomputers (quantum advantage around mid/late-2020s) and then to truly fault-tolerant computers tackling wide-ranging problems (in the early 2030s). The five announcements from AWS, Microsoft, Google, IBM, and Zuchongzhi have lit five different paths toward this endgame. It will be exciting to watch whether one path wins out or whether they converge. Given the collaborative spirit so far, it’s plausible the ultimate quantum computer will incorporate lessons and technologies from all of them. Each innovation — bosonic qubits, topological qubits, high-fidelity transmons, modular architectures — addresses a piece of the puzzle. By integrating the best of each, either in one machine or through a unified theory of quantum computing, the field could reach the milestone of a large-scale, error-corrected quantum computer sooner than many expect. What once looked like a distant theoretical possibility now has a concrete, if challenging, roadmap, and the developments of 2024–2025 have added considerable momentum to the journey.