Quantum Technology Use Cases in Aerospace & Automotive
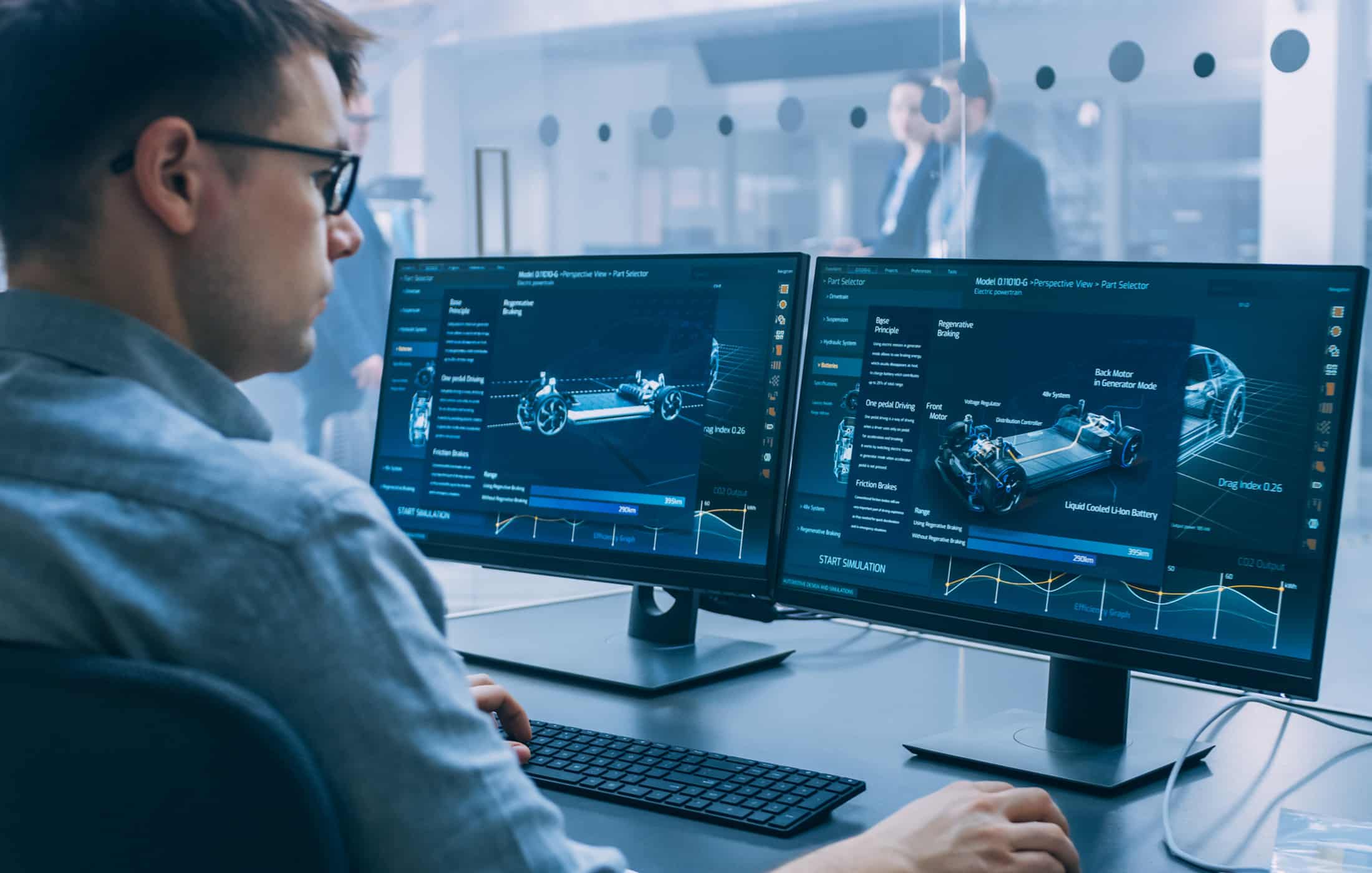
Table of Contents
Introduction
Quantum computing harnesses principles of quantum mechanics – like superposition and entanglement – to process information in ways that classical computers cannot. The allure is clear: large-scale, error-free quantum computers could theoretically perform tasks impossible for today’s supercomputers. This potential has massive implications for industries such as aerospace and automotive, where progress often hinges on crunching extremely complex computations. For example, aerospace engineers must simulate turbulent airflow around new aircraft designs – a feat so demanding that even the best classical simulations fall short, forcing reliance on costly wind tunnel tests. Likewise, automakers grapple with multifaceted challenges from vehicle dynamics to battery chemistry that tax conventional high-performance computing. Quantum computing promises orders-of-magnitude boosts in computing capability for these problems, making it a potential game-changer for designing better planes and cars, optimizing transportation networks, and accelerating innovation across both sectors.
Global interest and investment in quantum tech are soaring accordingly. In the United States, government funding for quantum computing R&D nearly doubled from $449 million in 2019 to about $968 million in 2024. Major aerospace and automotive companies are likewise pouring resources into quantum experimentation. The reason is simple: if quantum computers can eventually solve intractable equations for aerodynamics, materials science, or traffic optimization, the payoff would be transformative. Quantum algorithms might discover ultra-light yet strong composites for cars and aircraft, or instantly compute optimal routes for fleets to save fuel and time. In short, quantum computing’s ability to tackle “computationally intensive tasks” beyond classical limits positions it as a critical emerging technology for two of the world’s most technologically demanding industries.
Current Developments
Both the aerospace and automotive sectors have witnessed a flurry of quantum computing initiatives in recent years. Virtually all major automakers now have quantum projects or partnerships underway. Ford, BMW, Mercedes-Benz, Volkswagen, Hyundai and others have teamed with quantum hardware and software firms to explore practical use cases. For instance, Ford partnered with Quantinuum to apply quantum algorithms in modeling lithium-ion battery chemistry, while Mercedes-Benz has worked with IBM Quantum and Google Quantum AI on battery material research. Volkswagen collaborated with D-Wave, a quantum annealing company, to optimize urban traffic routing for buses, aiming to reduce congestion and fuel consumption. BMW Group has been particularly active – it not only launched several quantum pilot projects but even assembled an internal Quantum Computing team to publish research on algorithms for vehicle engineering and manufacturing. These corporate investments signal a broad recognition that quantum computing could unlock competitive advantages in design, efficiency, and innovation.
Public-private collaborations are also catalyzing progress. Airbus and BMW joined forces to launch the Quantum Mobility Quest, a global challenge inviting researchers and startups to tackle pressing aviation and automotive problems with quantum solutions. The competition, run with support from Amazon’s quantum services, highlights use cases ranging from quantum-enhanced aerodynamic design to machine learning for automated driving. In France, aerospace firm Thales is partnering with quantum startup Alice&Bob on a government-backed project (under the France 2030 plan) to develop algorithms for next-generation fault-tolerant quantum computers, with the goal of speeding up electromagnetic simulations for aircraft design. Similarly, the German Aerospace Center (DLR) has commissioned quantum computing ventures (QuantiCoM) with startups like Planqc and d-fine to accelerate the discovery of advanced materials. By leveraging quantum processors to simulate material properties, this DLR initiative aims to develop lighter alloys and more durable components for use in both airplanes and automobiles. Governments, academia, and industry are increasingly united in these efforts, reflecting a shared belief that early exploration of quantum technology will pay dividends as the hardware and algorithms mature.
Industry-Specific Use Cases
Quantum computing could profoundly influence how vehicles are engineered and manufactured. Automakers are already experimenting with quantum algorithms to design lighter cars, develop better batteries, and streamline production.
Automotive – Vehicle Design, Materials, Batteries & Efficiency
In the automotive arena, quantum computing is being applied to some of the most complex aspects of vehicle development. Vehicle design and engineering stand to benefit through faster, more detailed simulations. Today’s cars require intensive computational fluid dynamics (CFD) and finite-element analysis to refine aerodynamics, crash safety, and other performance factors – processes that can take days or weeks on classical supercomputers. Quantum methods promise to speed up such simulations. BMW, for example, has partnered with a quantum startup (Pasqal) to explore solving partial differential equations with quantum processors, reporting progress on simulating metal-forming processes for car parts. The long-term vision is that quantum solvers could one day handle high-resolution CFD or structural simulations in a fraction of the time, giving engineers more freedom to iterate designs. As NASA researchers noted, if quantum computers could “directly compute turbulence” for real-time design optimization, it would obviate many physical wind-tunnel tests and greatly improve designs – a paradigm shift that automotive designers eagerly anticipate as well.
Materials innovation is another key focus. Automakers are constantly seeking stronger, lighter materials (for chassis, body panels, etc.) to improve energy efficiency and safety. Quantum chemistry algorithms can model the behavior of molecules and materials at the atomic level far more naturally than classical methods. This makes them ideal for discovering novel alloys, polymers, or battery materials. In practice, companies are already using today’s small quantum processors in variational simulations to study material properties. Several carmakers have targeted battery chemistry in particular: Ford and Quantinuum used quantum algorithms to investigate lithium-ion battery reactions, while Hyundai and IonQ applied quantum simulations to optimize electrolytes and catalysts for next-generation batteries. The goal is to design batteries that are higher-density, faster-charging, and safer by understanding chemical interactions at a quantum level. Similarly, the DLR-led quantum materials project exemplifies cross-industry synergy – its quantum simulations are expected to yield lighter structural alloys and durable composites that could reduce vehicle weight and improve fuel efficiency in both cars and aircraft. By developing the “atoms of batteries with atoms” (as quantum pioneer Richard Feynman envisioned), automakers hope to leapfrog current limitations in energy storage and materials science.
Quantum computing also offers new ways to boost energy efficiency and overall vehicle performance. Beyond improving the materials themselves, quantum optimization can refine how vehicles use energy. For instance, Volkswagen’s experiment with D-Wave optimized public transit routes in a city to minimize stop-and-go traffic. A similar approach could optimize the power management within electric vehicles or find more efficient driving routes for logistics fleets, saving fuel or battery life. Even vehicle design choices can be framed as optimization problems – e.g. finding the ideal placement of sensors or the lightest structural topology that meets all strength requirements – which quantum algorithms might solve more effectively than brute-force classical searches. In the near term, many of these automotive quantum applications remain in prototype or simulation stages. Yet incremental successes are accumulating, reinforcing the expectation that quantum computing will progressively augment conventional R&D. Early trials have already achieved solutions “beyond the classical barrier” on specific optimization tasks, hinting at the enormous potential as devices improve. From concept to showroom, quantum computing is poised to touch every phase of the vehicle lifecycle: design, material selection, manufacturing, and even how cars are driven or operated.
Manufacturing & Supply Chain Optimization
Automotive and aerospace companies alike are exploring quantum approaches to streamline manufacturing and logistics – the behind-the-scenes backbone of these industries. Many of these challenges boil down to complex optimization problems, where quantum computers could evaluate countless variables simultaneously to find the best solution. Production scheduling and factory operations are prime examples. At an automotive plant, scheduling thousands of tasks (parts fabrication, robot movements, assembly line sequencing) is extraordinarily complex. BMW has piloted a quantum optimization project with Amazon Web Services to optimize robotic path planning on the factory floor. This is akin to solving a traveling salesman problem for assembly robots, finding the shortest paths that avoid collisions and minimize time. Classical algorithms struggle as the problem size grows, but quantum algorithms have shown promise in evaluating many possible robot trajectories in parallel to identify an optimal schedule. Smoother production flows can raise throughput and reduce costs.
Supply chain and logistics optimization is another lucrative area. Large manufacturers operate vast supply networks – sourcing parts from hundreds of suppliers, then distributing finished vehicles or aircraft worldwide. Even minor improvements in route planning or inventory management can yield huge savings in such scale. Quantum computers excel at exploring combinatorial possibilities, which is useful for routing and distribution challenges. One of the highlighted use cases in the Airbus-BMW Quantum Challenge is using quantum optimization for a “more sustainable supply chain,” aiming to reduce waste and emissions in transporting parts and products. In practice, Volkswagen’s earlier mentioned project with quantum annealing cut bus transit times by rerouting around congestion, and D-Wave worked with Toyota on optimizing traffic light timing at intersections – both demonstrators of traffic logistics problems that also apply to supply chains. These pilots suggest that quantum optimization could help coordinate everything from trucking routes to cargo loading in more efficient ways than classical heuristics. A McKinsey analysis noted that mobility and logistics stand to gain significant value from quantum computing in coming years, potentially tens of billions of dollars by 2035.
Quantum optimization isn’t the only tool in the kit here; quantum machine learning may also assist manufacturing. Quantum-enhanced ML could help with predictive maintenance (e.g. forecasting machine failures on an assembly line) or quality control (identifying defects). Although still experimental, researchers are investigating quantum algorithms to detect anomalies in sensor data faster, which could minimize downtime in factories. In short, whether it’s scheduling production, routing deliveries, or managing inventory levels, the labyrinthine logistics of aerospace and automotive production provide fertile ground for quantum solutions. Any improvement in these domains can translate to leaner operations and a stronger bottom line – hence the intense interest in being early adopters of quantum optimization technology for industry 4.0 applications.
Autonomous Vehicles & AI
The push toward self-driving cars and smarter aircraft control systems presents another frontier where quantum computing could make a difference. Autonomous vehicles rely heavily on artificial intelligence (AI) and sensor data processing. Here, quantum computing may augment both the training of AI models and their real-time decision-making capabilities. One immediate application is in machine learning for perception – teaching vehicles to recognize and react to their environment. In a recent collaboration, Hyundai and IonQ used a quantum machine learning approach for image classification, aiming to improve how autonomous car sensors identify road features and hazards. Quantum algorithms can, in theory, sift through and find patterns in large datasets (like camera or LiDAR inputs) more efficiently, potentially accelerating the training of vision models for self-driving cars.
Quantum computing might also enhance path planning and decision-making for autonomous systems. Self-driving cars must constantly make split-second judgments (when to brake, where to steer) based on myriad inputs. Classical AI can struggle with the combinatorial explosion of possible scenarios on busy roads. Quantum computers, however, are naturally adept at evaluating many possibilities in parallel. Researchers have proposed quantum algorithms to help autonomous vehicles predict the behavior of human drivers around them by analyzing vast driving datasets. By doing so, a quantum-enhanced system could better anticipate, say, that the car in the next lane might suddenly merge or that a pedestrian is about to jaywalk, and adjust accordingly. McKinsey analysts suggest that in the future, quantum computing may “improve the performance of sensors and vehicle systems, allowing them to make instant decisions when confronted with an obstacle in the road.” Faster, more reliable decision-making could be literally lifesaving in autonomous driving, where reaction time is critical.
Another consideration for autonomous and connected vehicles is security. As cars become “computers on wheels” that communicate with infrastructure and each other, protecting them from cyberattacks is paramount. However, the advent of powerful quantum computers also poses a risk: these machines could eventually crack standard encryption algorithms that keep vehicle communications secure. Automakers and their tech partners are already preparing for this by exploring quantum-safe cryptography. Post-quantum encryption schemes and even quantum communication methods (like quantum key distribution) are being evaluated to secure vehicle-to-vehicle and vehicle-to-cloud links. Quantum computing might assist on the defensive side too – for example, helping to generate unbreakable encryption or to detect intrusions by analyzing network traffic patterns. In fact, quantum-generated randomness and entangled communication could underpin the “quantum-safe” connected car of the future. Thus, even as quantum opens new capabilities for autonomous mobility, it forces the industry to rethink security from the ground up. Balancing these opportunities and threats will be a key part of the autonomous vehicle roadmap in the quantum era.
Aerospace – Propulsion, Materials, and Space
Aerospace companies are likewise pursuing quantum computing to tackle challenges in aircraft and spacecraft design, propulsion, and materials. One of the most tantalizing prospects is using quantum computers to design better propulsion systems and aerodynamics. Aircraft propulsion (be it jet engines, rockets, or emerging hydrogen fuel cells for planes) involves highly complex physics. Quantum simulations could significantly aid in this realm. Rolls-Royce, a leading jet engine manufacturer, has been exploring “quantum-inspired” computational fluid dynamics to improve engine aerodynamics. By incorporating quantum algorithms into the design process (for example, to simulate airflow or combustion at a granular level), engineers hope to discover designs that yield more thrust with less fuel. In fact, quantum simulations paired with digital twin models could allow rapid virtual testing of new engine configurations, shortening development cycles. Airbus, for its part, is looking at quantum computing to help with hydrogen fuel cell propulsion for future zero-emission aircraft. Fuel-cell chemistry is extremely hard to model with classical computing, but an Airbus researcher noted that quantum methods may finally crack this problem – “we need fuel cells… [which] are very difficult to model in software using classical computers,” and quantum computing could be the key to accurate simulations. Mastering such simulations would be crucial for Airbus’s plans around hydrogen-powered aviation.
In aerospace materials and manufacturing, the needs are similar to automotive but often even more extreme. Planes and spacecraft demand materials that are lightweight yet can endure high stress, temperature, and radiation. Quantum chemistry and materials science algorithms are thus of great interest for aerospace R&D. The same DLR quantum project aimed at new materials for vehicles explicitly targets aerospace use-cases: developing lighter alloys to reduce aircraft weight (hence fuel burn) and more durable materials to extend component life. Quantum computing’s ability to handle the quantum mechanics of novel composites or high-temperature alloys could lead to breakthroughs in airframe construction or rocket materials that traditional methods might miss. Moreover, aerospace manufacturers are interested in quantum optimization for processes like composite layup or 3D printing of parts (so-called space manufacturing). For future space missions, assembling structures in orbit or on the Moon will require meticulously optimized sequences of operations, and quantum algorithms could aid in planning those to ensure reliability and efficiency.
Even operations in the aerospace sector could get a quantum upgrade. Airlines and space agencies face massive logistical puzzles that echo the supply-chain issues discussed earlier: coordinating fleets of aircraft, plotting efficient flight routes, scheduling satellite launches, etc. The aerospace industry is “replete with problems of optimization”, notes an Aerospace America report, such as determining how to route many flights to many cities with minimum fuel or time. Quantum computers are naturally suited to such multivariable optimizations. In the coming years, an airline might use quantum computing to dynamically optimize its flight schedule and air traffic routings based on weather, airspace restrictions, and demand – a problem far too complex for brute-force classical solutions. Additionally, quantum sensing and communications are emerging technologies in aerospace (beyond computing per se) that merit mention. Quantum sensors can provide ultra-precise measurements of acceleration and rotation, which could revolutionize aircraft navigation systems, and quantum-encrypted communications could secure satellite links against eavesdropping. These complementary quantum technologies, alongside quantum computing, stand to enhance the entire aerospace ecosystem, from how we build planes to how we fly them and even how we explore space.
The Arrival of Universal Quantum Computing
Thus far, most practical applications have used today’s NISQ (Noisy Intermediate-Scale Quantum) devices – machines with tens or hundreds of imperfect qubits that are prone to errors. While useful for experimentation, NISQ systems can only handle relatively small problem instances before decoherence and noise overwhelm the calculations. The real disruption is expected when fault-tolerant quantum computers (FTQC) arrive. These future machines will incorporate error-correcting codes and large numbers of stable qubits, enabling reliable, large-scale quantum computations. Quantum industry experts are optimistic on this timeline: over 65% of leading researchers and executives surveyed anticipate “the arrival of fault-tolerant QC by 2030.” In other words, within this decade we may have quantum computers capable of solving industrial problems that are currently out of reach. There is debate on what exact form “fault tolerance” must take – some argue useful applications may emerge even slightly before full error-correction at scale – but the consensus is that we are steadily marching toward far more powerful quantum hardware.
When these universal quantum computers come online, the impact on aerospace and automotive industries could be transformative. Fully error-corrected quantum computers would let engineers tackle “grand challenge” computations head-on. For automakers, this might mean no longer approximating molecular simulations or optimization problems in chunks – an FTQC could handle them end-to-end with quantum algorithms. The oft-stated dream is to perform “full molecular and materials modeling” without shortcuts. With a sufficiently large quantum computer, a car company could simulate an entire battery’s chemistry at atomic detail, or explore thousands of material combinations for a vehicle frame to find the absolute optimal choice, something impossible on classical machines. This could yield ultra-efficient electric vehicles with batteries engineered for peak performance, or automobiles made of super-light materials optimized for strength and weight down to the molecular level. Aerodynamic designs could also be revolutionized. As NASA technologists speculate, a fault-tolerant quantum computer might directly solve fluid dynamics equations for a complete aircraft in real-time. Instead of weeks of supercomputing or months of wind-tunnel tests, designers could evaluate new wing or engine designs virtually and instantaneously, iterating toward radical configurations that break current performance barriers.
The operational optimizations discussed (traffic, routing, scheduling) would likewise scale to new heights with universal quantum computing. Complex coordination problems – like managing the routes of tens of thousands of autonomous cars simultaneously, or scheduling an entire year of airline operations for a major carrier – could potentially be solved exactly rather than via today’s heuristics. Such capabilities would disrupt business models: imagine perfectly efficient logistics networks or air traffic flows with minimal delays. Even entirely new concepts become plausible. For instance, some envision a “quantum-driven internet of vehicles” where cars communicate via quantum networks and share quantum-generated intelligence for traffic management. In aerospace, a network of satellites could use quantum optimization to configure itself for optimal global coverage or to dynamically respond to mission needs. All told, fault-tolerant quantum computers promise to unlock a wave of innovation: they’ll allow aerospace and automotive innovators to ask “what if?” on a grand scale and actually get answers. Of course, realizing this future requires surmounting major scientific hurdles in qubit stability and error correction – a task companies like IBM, Google, and many startups are racing to accomplish. But if and when they succeed, the road and sky ahead could look very different, powered by quantum-optimized designs and operations.
Sector Preparation & Responses
Given the high stakes, aerospace and automotive players are not waiting idly for quantum supremacy to arrive – they are actively preparing for the quantum era now. A key aspect of preparation is building expertise and partnerships. Many companies have formed dedicated internal teams or research programs focused on quantum computing. BMW Group, for example, has a Quantum Computing team that engages in research (as evidenced by their authorship of academic papers) and collaborates with external quantum firms. Volkswagen’s data lab has been experimenting with quantum algorithms for years, and Daimler (Mercedes-Benz) likewise set up in-house quantum specialists. These teams not only run pilot projects but also serve to educate the broader organization about quantum technology’s potential. Workforce development is crucial – there’s a recognized shortage of quantum-trained talent, so companies are upskilling engineers and recruiting quantum scientists to be ready for wider adoption. Government and academia are helping here: in the UK, for instance, the Digital Catapult runs a Quantum Technology Access Programme (QTAP) to provide aerospace companies with training, education, and access to quantum experts for solving industry problems. Such initiatives aim to bridge the gap between cutting-edge quantum research and real-world industrial use, ensuring a pipeline of knowledgeable practitioners.
Another pillar of preparedness is strategic partnerships and ecosystem building. Automakers and aerospace firms are partnering with quantum hardware providers, software startups, and cloud computing platforms to jointly explore applications. These alliances give industry players early access to quantum machines and expertise, while tech companies gain valuable use cases and feedback. We’ve seen numerous examples: Airbus partnering with IonQ on quantum algorithms for flight optimization, Hyundai with IonQ for autonomous driving AI, Ford with quantum software startup QC Ware, Boeing investing in quantum research through government programs, and so on. Collaborations often extend to academia as well; companies are sponsoring research at universities and participating in consortiums (like the EU Quantum Flagship or U.S. National Quantum Initiative projects) that relate to transportation. Even competitors sometimes come together: the Airbus-BMW Quantum Challenge is a case where two industry giants jointly foster an ecosystem of innovators tackling mobility problems with quantum. By engaging in the quantum community early, aerospace and automotive firms hope to influence the direction of research towards their needs and avoid being caught off-guard by disruptive breakthroughs.
Corporate strategy is also evolving to incorporate quantum readiness. Some forward-looking companies are already considering the implications for infrastructure and security. For example, sensitive communications and data within automotive companies are being evaluated for migration to post-quantum cryptography standards, anticipating future cybersecurity threats. In aerospace, satellite operators and defense contractors are researching quantum-resistant encryption to protect communications against eventual quantum code-breaking. On the opportunity side, businesses are creating roadmaps for gradually ramping up quantum usage – starting with hybrid classical-quantum computing for certain optimization tasks, then expanding as hardware improves. There’s recognition that the learning curve is steep: integrating quantum workflows into existing engineering or IT processes is non-trivial. As a result, companies are doing trial runs now (proof-of-concepts, simulations on quantum cloud platforms) to uncover practical challenges in data integration, scaling, and algorithm tuning. All these preparations – talent development, partnerships, pilot projects, and risk mitigation – amount to a concerted effort by the aerospace and automotive sectors to be “quantum-ready.” The consensus is that quantum computing will bring disruption, and those who have built up experience and resources in advance will be best positioned to capitalize on it.
Challenges and Risks
For all the excitement, it’s important to acknowledge that quantum computing is not a magic bullet and faces considerable challenges, especially in these complex industries. Technical limitations are the most immediate hurdle. Current quantum hardware is still immature – qubits are prone to error, and scaling up their number remains daunting. Noisy qubits mean that many quantum algorithms can’t run at the size needed to beat classical methods yet. While small instances of optimization or chemistry problems have been solved on quantum devices, these often don’t translate to industrial-scale cases due to noise and decoherence. A 2024 Boston Consulting Group report highlighted that progress in quantum algorithms has been slower than initially hoped and that advances in classical computing (like faster GPUs and better AI algorithms) continue to raise the bar for achieving a clear quantum advantage. In practice, this means aerospace and automotive firms must navigate a period of uncertainty where quantum solutions might not consistently outperform conventional high-performance computing. The risk of hype is real – over-promising could lead to disillusionment. Some companies have been hesitant to invest too heavily right now because classical HPC “costs less and is equally likely to solve most problems, albeit more slowly,” as McKinsey notes. They worry about sinking costs into quantum experiments that may not yield immediate ROI. This conservative stance could cause late adoption, but over-caution carries its own risk if the technology progresses faster than expected.
Data security is another major concern. The same quantum power that could revolutionize computation also threatens the foundations of current cryptography. Aerospace and automotive companies deal with sensitive information (from proprietary designs to customer data to operational commands for vehicles). Encryption protocols that protect this data – such as RSA and elliptic-curve cryptography – could be broken by a sufficiently large quantum computer running Shor’s algorithm. This looming threat has been described as a “harvest now, decrypt later” scenario in cybersecurity circles: adversaries might hoard encrypted data now, with the intent of decrypting it once quantum capability is available. To counter this, industries must transition to post-quantum cryptography in the coming years. However, rolling out new encryption standards across global supply chains, millions of vehicles, and communication networks is a non-trivial task subject to regulatory oversight and compatibility issues. Until that transition is achieved, there is a window of vulnerability where data (including vehicle-to-cloud communications or satellite links) could be retroactively exposed. Companies are heeding recommendations to begin migrating to quantum-safe encryption sooner rather than later, but the path requires coordination with governments and tech providers. On top of that, intellectual property in the quantum domain is a concern – firms worry about protecting their quantum algorithms and findings, which could become competitive differentiators. As more partnerships form, managing IP and trade secrets adds a layer of risk (who owns a breakthrough algorithm co-developed by an automaker and a quantum startup, for example).
Regulatory and adoption challenges also loom. In tightly regulated industries, introducing a novel technology like quantum computing requires navigating compliance and certification. In aviation, any new method that affects aircraft design or operation (even an algorithm that optimizes a flight plan) may need approval from regulators to ensure it meets safety standards. How do you certify the result of a quantum computation? Ensuring that quantum-optimized designs or AI decisions are safe and trustworthy will take work, possibly requiring new regulatory frameworks. Automotive regulations, too, may need updating – for instance, validating that an autonomous driving decision made with the aid of a quantum algorithm adheres to road safety rules. Regulators themselves face a knowledge gap on quantum topics, which could slow down acceptance of quantum-derived solutions until understanding matures. On the organizational side, companies must contend with the cost and complexity of adopting quantum tools. Quantum hardware is expensive and often accessible only via cloud services, raising questions about data governance (e.g., can a car company securely send proprietary design data to a quantum cloud?). There is also the talent gap: quantum specialists are in short supply, and retraining existing engineers in quantum computing concepts is challenging. Early adopter firms report that integrating classical and quantum workflows is tricky – it often requires custom software development and close collaboration between domain experts and quantum scientists.
Finally, there’s the risk of uncertain timelines. While many anticipate breakthroughs by 2030, it’s possible that mastering fault tolerance could take longer, or that some promised applications turn out to be less effective than hoped. Companies investing heavily now are, to an extent, betting on an uncertain future. They are funnelling resources into NISQ-era projects “despite little evidence of quantum advantage, or, at best, marginal advantage” so far. If progress stalls, there’s a possibility of a quantum winter where funding and interest dry up. Conversely, if progress accelerates unexpectedly, latecomers could be left scrambling. This uncertainty requires a balanced approach: stay engaged with quantum developments, but also be realistic about current capabilities. In summary, the road to quantum-enabled aerospace and automotive industries is not without potholes. Technical hurdles, security threats, regulatory puzzles, and adoption pains all need to be managed carefully. Many of these industries’ leaders emphasize that quantum computing is a long-term play – a marathon, not a sprint – and that steady, pragmatic advancement (with clear benchmarks and risk mitigation) is the way to cross the finish line.
Conclusion
Quantum computing is on the verge of reshaping the future of both aerospace and automotive sectors, even if the technology’s full maturation is still years away. In this article, we’ve seen that current developments – from corporate partnerships and research alliances to early quantum prototypes tackling real use cases – have already laid the groundwork. Automotive companies are using quantum algorithms in pilot projects to optimize everything from battery chemistry to factory logistics, and aerospace engineers are testing quantum methods for design optimization and materials discovery. These early successes, while limited in scope, are invaluable learning experiences. They signal what’s possible and are helping companies build internal expertise so they can quickly scale up when more powerful quantum computers become available.
Looking at industry-specific applications, it’s evident that the impact of quantum computing will be broad. In automotive, quantum computing could enhance vehicle design through better simulation, enable innovation in lightweight materials and high-capacity batteries, and improve the efficiency of manufacturing and mobility services. The aerospace industry could see quantum-driven advances in propulsion technology (like more efficient jet engines or viable hydrogen fuel systems), new composite materials for aircraft and spacecraft, and optimized operations from airlines to satellite constellations. Some of these use cases will materialize sooner (e.g. niche optimization tasks that even current quantum machines can handle), while others await the advent of universal quantum computing to truly blossom. When fault-tolerant quantum computers arrive, potentially in the next decade, they could trigger a paradigm shift – solving problems that today we can only approximate or dream about. That could mean virtually designing an entire airplane or car at the molecular level before building a single physical prototype, or managing global transportation networks with unprecedented precision and intelligence.