Zuchongzhi 3.0 Quantum Chip: Technical Analysis and Implications
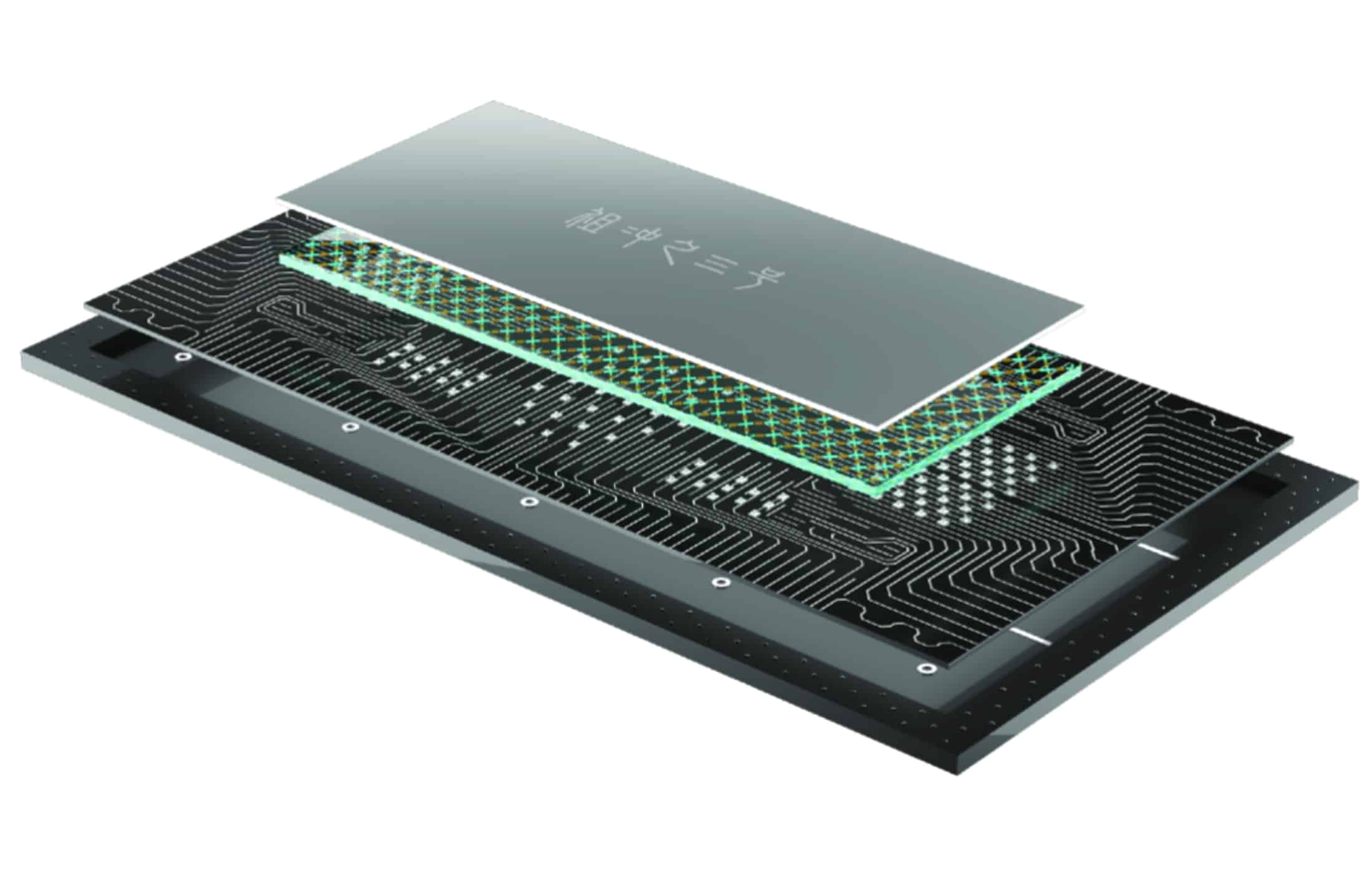
Table of Contents
China’s quantum computing powerhouse, the Zuchongzhi research teams, just unveiled Zuchongzhi 3.0, a new superconducting quantum processor with 105 qubits, marking a major leap in quantum computing performance. Announced in March 2025 by a University of Science and Technology of China (USTC) team led by Pan Jianwei, Zhu Xiaobo, and Peng Chengzhi, this prototype claims to have achieved unprecedented processing speed – reportedly quadrillion (1015) times faster than today’s best supercomputer and about one million times faster than Google’s latest quantum chip results announced just a few months ago. More on this benchmark later. Let’s dig into the announcement and the accompanying paper: Establishing a New Benchmark in Quantum Computational Advantage with 105-qubit Zuchongzhi 3.0 Processor.
Technical Advancements of Zuchongzhi 3.0
Architecture and Qubit Design
Zuchongzhi 3.0 is a 105-qubit superconducting processor fabricated with a two-dimensional grid (rectangular lattice) architecture. The qubits are coupled via a dense network of 182 tunable couplers, enabling flexible two-qubit interactions across the chip. This 2D layout (with an average connectivity of ~3.5 neighboring qubits per qubit) maximizes entanglement opportunities while mitigating signal cross-talk. The chip adopts a “flip-chip” integration technique, where two chips are bonded face-to-face, achieving high-density interconnects with minimal signal loss. This innovation, along with a sapphire substrate and improved circuit materials (tantalum-aluminum), significantly reduces electromagnetic noise and enhances thermal stability. The result is an extended qubit coherence: Zuchongzhi 3.0’s qubits have an average energy-relaxation (T₁) time of ~72 µs (and dephasing T₂ ~58 µs) – a notable improvement in stability over its predecessor.
Qubit Count and Fidelity Improvements
With 105 programmable qubits, Zuchongzhi 3.0 nearly doubles the 66-qubit scale of the earlier Zuchongzhi 2.1, pushing China’s hardware into the 100+ qubit realm. Just as important as qubit count, however, is qubit quality. The USTC team reports state-of-the-art fidelities: single-qubit gate operations succeed 99.90% of the time, two-qubit gates 99.62%, and readout measurements 99.13%. These fidelities are a significant upgrade from the previous 66-qubit device and were achieved through refined chip fabrication and noise mitigation techniques. By comparison, Google’s latest 105-qubit “Willow” chip has slightly higher fidelities (~99.965% single-qubit, 99.86% two-qubit) and longer coherence (~98 µs T₁), but Zuchongzhi 3.0’s metrics are in the same competitive class. The Chinese chip’s gate speeds are also fast (single-qubit ~28 ns, two-qubit ~45 ns per operation, on par with tens of nanoseconds for Willow). These advances mean Zuchongzhi 3.0 can execute deeper and more complex quantum circuits within the qubits’ coherence time, compared to earlier Chinese processors.
Unique Features
Beyond raw numbers, Zuchongzhi 3.0 showcases engineering breakthroughs that set it apart. The use of a flip-chip, heterogeneous 3D packaging approach is novel in USTC’s designs, helping cram more qubits and couplers without sacrificing signal integrity. Its sapphire-based chip (a low-loss dielectric material) and custom microwave attenuators minimize decoherence, effectively extending qubit lifetimes. These design choices collectively boosted the chip’s quantum volume and reliability. The researchers also employed improved calibration and error mitigation to squeeze out every bit of performance. Peer reviewers have lauded Zuchongzhi 3.0 as “state-of-the-art” and a “significant upgrade from the previous 66-qubit device” in terms of benchmarking a new superconducting quantum computer. In short, the chip marries high qubit quantity with high quality, a critical combination for pushing toward practical quantum computing.
Performance Claims and Benchmarking
Quantum Advantage Demonstration
Zuchongzhi 3.0’s headline achievement is demonstrating the strongest quantum computational advantage to date on a superconducting platform. The team ran a demanding random quantum circuit sampling (RCS) task, a common benchmark for “quantum supremacy/advantage.” In this experiment, the processor sampled the output of a random sequence of quantum gates applied to 83 qubits over 32 cycles, generating one million measured samples. This type of computation is designed to be extremely hard to simulate on a classical computer, as the quantum circuit’s complexity grows exponentially with the number of qubits and depth.
The results are striking: Zuchongzhi 3.0 produced the million samples in mere hundreds of seconds (on the order of a few minutes). By contrast, the best-known classical algorithms would take astronomically long to do the same. According to the USTC team’s analysis, simulating the 83-qubit, 32-cycle random circuit would require the Frontier supercomputer about 6.4 billion years (roughly half the age of the universe) to match what Zuchongzhi did in minutes. In other words, the quantum processor outpaced the fastest classical supercomputer by an incredible 1015-fold factor (15 orders of magnitude) on this task. This “quantum computational advantage” – performing a calculation essentially infeasible for any classical computer – sets a new record in the superconducting quantum computing arena.
Equally attention-grabbing is Zuchongzhi 3.0’s speedup over Google’s recent quantum processor. The Chinese team reports their prototype is about one million times faster than Google’s latest published result. In late 2024, Google’s Quantum AI lab had demonstrated a random circuit sampling with a 105-qubit chip (Willow) completing in under 5 minutes, a task they estimated would take a classical supercomputer on the order of 1025 years (10 septillion years) to simulate. That achievement corresponded to a ~109-fold quantum speedup (nine orders of magnitude) over classical, re-establishing quantum advantage for Google after improved classical methods had caught up to earlier experiments. Zuchongzhi 3.0’s experiment, by comparison, yields a 1015-fold speedup, besting Google’s October 2024 result by about 6 orders of magnitude.
Validity of Claims and Benchmark Methods
It’s important to scrutinize these performance claims in context. The million-fold speedup pertains to a very specific benchmark (random circuit sampling) rather than a general-purpose computation. RCS is essentially a proof-of-concept task – it doesn’t solve a useful problem but is a stress test for quantum hardware vs. classical simulation. The USTC team took care to compare against the best available classical algorithms for this task, even those developed by their own researchers. Notably, in 2023 USTC scientists had optimized classical simulation of Google’s 2019 supremacy experiment, reducing a 10,000-year task to just ~14 seconds using 1,400 GPUs. That work famously overturned Google’s 2019 “quantum supremacy” claim, showing that improved classical algorithms and bigger supercomputers (like Frontier) could challenge earlier quantum advantage results. Armed with that insight, the Zuchongzhi 3.0 team benchmarked their new processor against the optimal classical simulation methods known in 2025, ensuring the quantum speedup is genuine under current knowledge. By dramatically widening the gap – 1015 vs 109 in the Google case – they have restored a comfortable quantum lead (for now) in this ongoing leapfrog competition.
The benchmarking methods used were rigorous. The team ran many trials of random circuits and used statistical metrics (like cross-entropy benchmarking) to verify the quantum outputs, since direct classical verification of the full 83-qubit circuit is impossible. They also employed “patch circuit” fidelity checks, breaking down the large circuit into smaller pieces that can be classically simulated to estimate the overall accuracy of the quantum sampling. The reported fidelity of the quantum sampling results matched expectations, suggesting the processor was indeed operating as intended. While we must trust the experimenters’ analysis (because no classical computer can double-check the full result), the publication in a peer-reviewed journal (Physical Review Letters) indicates the methods passed scientific scrutiny. In fact, PRL’s reviewers called the work “benchmarking a new superconducting quantum computer, which shows state-of-the-art performance.”
That said, caution is warranted in interpreting “million times faster” beyond the narrow scope of this experiment. History has shown that quantum advantage claims can be transient as algorithms improve. Google’s 2019 feat was nullified by classical advances by 2023. It’s conceivable (though increasingly challenging) that clever algorithmic breakthroughs or future exascale supercomputers could shave down the 6.4 billion-year classical gap cited for Zuchongzhi 3.0. For now, the claim stands solid – Zuchongzhi 3.0 has demonstrated an unassailable lead on a specific computational problem – but the race between quantum hardware and classical simulation continues. Both the USTC and Google teams acknowledge they were effectively measuring different aspects of progress: Zuchongzhi 3.0 pushed scale and speed, whereas Google’s Willow emphasized accuracy via error-corrected qubits. As such, each claim of supremacy/advantage comes with caveats about what was measured. The USTC experiment maximized circuit size and complexity (while keeping error rates just low enough), whereas Google’s experiment validated that logical qubits can surpass physical qubits in reliability. Both are crucial milestones on the road to useful quantum computers.
Geopolitical Implications and Tech Race Dynamics
The Zuchongzhi 3.0 announcement underscores how quantum computing has become a strategic frontier in the U.S.–China technology race. Achieving quantum computational advantage is not just a scientific milestone but also, as Chinese media put it, a “direct indicator of a nation’s research strength in this field.” Currently, China and the United States are the two global frontrunners in quantum computing research, with each country alternating breakthroughs in recent years. The U.S. took an early lead (Google’s Sycamore in 2019 was first to claim quantum supremacy), China answered with photonic and superconducting prototypes (Jiuzhang and Zuchongzhi) achieving quantum advantage in 2020–2021, and the back-and-forth has continued. This competitive narrative is reinforced by the Zuchongzhi 3.0 team themselves, who told the press that their latest experiment “is keeping China on par with the U.S. in quantum computing research.” Each new milestone is watched closely as a barometer of national progress in a critical emerging technology.
National Strategies and Investment
Both nations have elevated quantum technology as a priority area, backed by large investments. China’s government, for example, has poured funding into quantum R&D, including a $10 billion National Laboratory for Quantum Information Sciences in Hefei that opened around 2020. This sprawling supercenter is intended to make China the global leader in quantum computing and sensing, and is the lab where Zuchongzhi 3.0 was developed. It’s part of a broader Chinese strategy (outlined in the 13th and 14th Five-Year Plans) to achieve breakthroughs in quantum communications, computing, and metrology – fields with both civilian and military significance. The U.S., for its part, launched the National Quantum Initiative Act (2018) and subsequent programs to coordinate quantum research, investing on the order of $200 million per year in quantum computing R&D at national labs, universities, and companies. American tech giants (Google, IBM, Microsoft, AWS) and startups are heavily funded, and the U.S. government considers quantum computing a critical field for federal support. In short, both countries view leadership in quantum computing as strategically vital – akin to a new “space race” or “arms race” in computation (though the “arms” in this case are more about potential code-breaking capabilities and computing dominance than physical weapons).
Techno-Strategic Impact
The advances in Zuchongzhi 3.0 will likely intensify this competition. Achieving quantum advantage in superconducting qubits bolsters China’s credibility in quantum tech, possibly accelerating its national quantum programs. It may also influence policy in the U.S.; for example, increased funding for quantum research or tightened export controls on certain quantum-related technologies. (Notably, some components of quantum computing – such as cryogenic systems or specialized electronics – could become subjects of trade restrictions if they are seen as conferring strategic military advantage in code decryption or otherwise.) Both nations are also eyeing the long-term economic impact: quantum computing could revolutionize industries from pharmaceuticals to finance. Being at the forefront means access to future breakthroughs and high-tech leadership. This competitive drive, however, is coupled with a spirit of scientific openness – the USTC team published their findings in international journals and on arXiv, and Google’s results were in Nature. In the near term, we may see a “quantum gap” narrative emerge, analogous to the “AI gap,” where each side touts their latest achievement and strives not to fall behind. As of 2025, China’s Zuchongzhi 3.0 and Google’s Willow each demonstrate different bragging rights – China claims the speed crown in raw quantum processing, while the U.S. claims an edge in error-corrected quantum computing. This dual development path highlights that the race is multifaceted: it’s not just about qubit count, but also qubit quality and scalability.
Comparison with Other Leading Quantum Processors
Zuchongzhi 3.0 enters a landscape of rapid advances by several players. Here’s how it compares to Google’s Willow and other cutting-edge quantum processors from IBM, AWS, and Microsoft:
Google Willow (2024, superconducting): 105 qubits, like Zuchongzhi 3.0, with a similar 2D grid architecture. Willow’s focus is on quantum error correction – it was the first chip to demonstrate that logical (error-corrected) qubits can outperform physical qubits in fidelity. Using the surface code, Google showed a logical qubit error rate below 0.2% per cycle, surpassing the physical qubit error (~0.6%). This was a breakthrough toward fault-tolerant quantum computing. Willow also performed a high-complexity RCS benchmark (67–100 qubit range) in under 5 minutes, which would take a classical supercomputer an estimated 1025 years to simulate – a 109× speedup indicating quantum advantage. Zuchongzhi 3.0 and Willow are quite similar in hardware capabilities (same qubit count and connectivity). Willow has a slight edge in coherence and gate fidelity (e.g. 99.86% two-qubit fidelity vs Zuchongzhi’s 99.62%) , reflecting Google’s long refinement of transmon qubit design. However, Zuchongzhi 3.0 utilized its qubits to run a larger scale circuit (83 qubits × 32 layers) for a sampling task, whereas Google limited its test to a somewhat smaller circuit (e.g. 67 qubits × 32 layers in the published experiment). In short, Zuchongzhi aimed for raw computational power, while Willow aimed for reliability and building blocks for a scalable machine. Both approaches are complementary. Zuchongzhi 3.0 achieved a record quantum speedup with physical qubits, and Willow achieved a milestone in reducing errors that will matter for real algorithms.
IBM Heron R2 (2024, superconducting): 156 qubits in a heavy-hexagon lattice architecture (tunable-coupler transmon qubits). Unveiled at the IBM Quantum Summit 2023, Heron R2 is IBM’s highest-performance quantum processor, emphasizing a modular and scalable design. IBM reported that the R2 chip plus its Qiskit software stack can execute complex circuits up to 50× faster than previous systems. Specifically, it can reliably run circuits with 5,000 two-qubit gate operations – double the prior record – thanks to improvements in both coherence and compiler optimizations. IBM has not explicitly claimed a “supremacy”-type sampling experiment with Heron R2; instead, IBM’s rhetoric is about reaching “quantum utility”, i.e. the point where their quantum system can do useful scientific computations beyond what classical can do in a reasonable time. Comparison: Heron R2 has more qubits than Zuchongzhi 3.0, but IBM often runs many of them at relatively lower circuit depth or with error mitigation, targeting practical problems (like simulating molecules or running small optimization algorithms). IBM’s heavy-hex lattice has fewer connections per qubit on average (to reduce cross-talk), and each qubit’s fidelity is high but somewhat lower than Google/USTC’s best (IBM reported ~99.5% two-qubit fidelity on earlier 127-qubit chips, and continuing improvements). A key differentiator is IBM’s modular approach – Heron qubits are designed to be the basis of a tile that can be scaled out and connected via communication links in a larger quantum system. While Zuchongzhi 3.0 smashed a specific benchmark, IBM’s strategy is to integrate hardware and software for more broadly useful performance. As evidence, IBM recently demonstrated a 127-qubit experiment achieving quantum advantage in simulating a physical system (a noisy spin-model dynamics) where the quantum computer outpaced leading classical methods. So, IBM’s Heron R2 is a strong competitor, trading blows in qubit count and aiming at real-world applications rather than pure speed tests.
AWS Ocelot (2025, superconducting cat-qubits): Amazon Web Services’ first quantum chip, introduced in February 2025, is a departure from the transmon approach. Ocelot uses “cat qubits” (Schrödinger cat state qubits) – microwave resonators that encode qubits in superpositions of two oscillator states – which intrinsically suppress certain error types. It’s a small-scale prototype (the exact qubit count isn’t publicly emphasized, but it’s on the order of only a few qubits) meant to test AWS’s hardware-efficient error correction ideas. AWS claims Ocelot’s architecture could reduce the resources for error correction by 5–10× (up to 90% less overhead) compared to traditional qubits. They achieved this by building error correction in from the ground up: the cat qubits plus additional circuitry form a repetition-code that passively corrects some errors, drastically lowering the extra qubit count needed for fault tolerance. In a Nature paper, AWS showed Ocelot can detect and correct certain single-qubit errors in real time, an encouraging step for scalability. Ocelot is focused on error-corrected qubit design rather than raw computation right now. It lags far behind in qubit number (likely <10 physical qubits in current tests) and isn’t trying to demonstrate quantum advantage. Instead, its significance is that it might pave the way for hardware-efficient, scalable quantum computers that don’t require thousands of physical qubits per logical qubit. If Zuchongzhi 3.0 and Willow are like racecars pushing performance, Ocelot is an experimental vehicle testing a radically different engine. In the long run, if Ocelot’s cat qubits can be scaled up, they could offer an alternative path to a large, stable quantum computer with far fewer total qubits needed. For now, Chinese and Google chips hold the speed records, while AWS’s chip offers a promising concept in the quest to tame quantum errors.
Microsoft Majorana 1 (2025, topological qubits): Microsoft unveiled “Majorana 1” in Feb 2025 as the world’s first quantum processor using topological qubits. This approach is radically different: it relies on exotic quasiparticles called Majorana zero modes formed in special semiconductor–superconductor structures (sometimes dubbed “topoconductors”). The promise is that qubits encoded in topological states will be inherently protected from many errors, potentially making quantum computers far more stable and scalable. Majorana 1 currently has 8 topological qubits on a chip, a modest count, but the chip is designed with a “Topological Core” architecture intended to scale to one million qubits in the future. Microsoft’s approach has been 17+ years in the making, overcoming the fundamental challenge of proving the existence and control of Majorana particles. In 2022–2023 they achieved key physics milestones (observing signatures of Majorana modes), and now with Majorana 1 they claim to have the “transistor for the quantum age” – a prototype qubit that could eventually allow error-resistant quantum computation at large scale. Comparison: In terms of near-term processing power, Majorana 1’s 8 qubits don’t compete with 100-qubit superconducting chips at all – it cannot yet perform any task faster than a classical computer. Its importance lies in the long-term game: if topological qubits can be scaled, Microsoft believes quantum computers capable of “solving meaningful, industrial-scale problems in years, not decades” might be possible. A million-qubit topological quantum computer is projected to do things like break modern encryption or simulate complex chemicals with ease – tasks far out of reach today. The catch is that Majorana qubits are still unproven in large numbers; even the existence of stable Majorana modes is an ongoing research effort, and Microsoft’s claims will need thorough peer review. In summary, Majorana 1 vs Zuchongzhi 3.0 is like a distant-future bet vs a present accomplishment: the Chinese chip shows what can be done today with superconducting qubits, while Microsoft’s chip explores what might be possible tomorrow with a fundamentally different qubit that could circumvent the toughest challenges of today’s technology.
Mathematical and Scientific Insights
While the engineering feats are impressive, it’s worth highlighting some mathematical and scientific concepts behind Zuchongzhi 3.0’s breakthrough:
Random Circuit Sampling Complexity
The task used to demonstrate quantum advantage – random circuit sampling – is grounded in computational complexity theory. Essentially, output probabilities of random quantum circuits form a distribution that is extremely hard to compute classically because it involves summing up 2n complex amplitudes for $$n$$ qubits. For Zuchongzhi 3.0’s 83-qubit circuit, the full quantum state has $$2^{83} \approx 10^{25}$$ terms. Simulating even the probability of a single bitstring output is intractable when $$n$$ and the circuit depth are high. This is why the classical estimated runtime (6.4 billion years) is so astronomical. The exponential scaling of classical computation versus linear scaling of actual quantum experiment time is the crux of quantum advantage. Mathematically, each additional qubit in such a random circuit potentially doubles the classical computational cost, and each additional layer multiplies the cost further. Zuchongzhi 3.0 leveraged this by running a circuit at the bleeding edge of size that the quantum hardware could handle (limited by error rates and coherence) such that classical algorithms are utterly overwhelmed. The fact that Google’s jump from 53 to ~67 qubits (plus improved circuit depth) raised classical difficulty from 104 years to 10{25} years shows how sensitive to scale these quantum vs classical comparisons are. Zuchongzhi 3.0’s 83-qubit demonstration pushes that envelope even further.
Error Rates and Quantum Error Correction
One key insight from comparing Zuchongzhi 3.0 and Google’s Willow is the trade-off between adding more qubits vs improving qubit fidelity. Mathematically, there is a threshold theorem in quantum computing: if physical error rates per operation can be pushed below a certain threshold (on the order of 1% or less, depending on the code), then quantum error correction (QEC) can in theory reduce logical error rates exponentially and allow indefinite computation. Google’s Willow made a notable scientific step by achieving an error per logical operation of ~0.1–0.2%, using 49 physical qubits to encode a single logical qubit in a surface code. This was below the ~0.5% error rate of their physical gates, meaning scaling up the code (more qubits in the logical qubit) actually improved fidelity – a first in the industry. In contrast, Zuchongzhi 3.0’s approach was to use all qubits directly (no QEC yet) but with very high fidelity gates (~0.4% two-qubit error). Scientifically, both approaches inform the path forward: Zuchongzhi 3.0 shows how far you can get by brute-force quantum computation with minimal errors, while Willow shows that fault-tolerance is within reach if we are willing to sacrifice a large fraction of qubits to redundancy. The USTC team recognizes this and is now pursuing QEC on Zuchongzhi 3.0: they reported working on surface code error correction with distance 7, and plans to extend to distance 9 and 11, which will use many of the 105 qubits to create a few robust logical qubits. Each increment in code distance should further suppress logical errors, paving the way for large-scale integration and control. This convergence of high qubit count and QEC will be essential for tackling useful problems.
Entanglement and 2D Connectivity
The inclusion of 182 couplers for 105 qubits implies a highly entangled system. Zuchongzhi 3.0’s 2D grid connectivity allows each qubit to interact with up to four neighbors, enabling complex entanglement patterns. Entanglement is the resource that gives quantum computers their edge, but it also makes classical simulation exponentially hard. The science of entanglement in such chips is interesting: a 83-qubit random circuit likely generates near-maximally entangled states across the chip. Measuring and verifying this entanglement indirectly via statistical tests (like linear cross-entropy) is part of the experiment’s methodology. In essence, Zuchongzhi 3.0 is testing the foundations of quantum mechanics at scale: by running these massive entangled circuits and seeing the expected chaotic output distribution, they affirm that quantum mechanics works as expected for 100-qubit systems. It’s a validation of quantum physics in a regime never directly simulated before.
In summary, Zuchongzhi 3.0’s success required a careful balance of mathematics and physics: they pushed their hardware to a regime of quantum complexity beyond classical reach, while keeping error rates just low enough that the quantum computation remained valid. It highlights the interplay of quantity vs quality in qubits, and provides empirical evidence supporting theoretical predictions about quantum advantage.
Cybersecurity Implications
A Quantum Milestone, Not an Immediate Threat. Zuchongzhi 3.0 achieved a specialized task (random circuit sampling) in seconds that would take a classical supercomputer billions of years. However, this benchmark was a contrived problem designed to showcase quantum prowess and does not directly translate to practical applications. In particular, it doesn’t mean current encryption can be cracked. The experiment demonstrated computational power on a narrow task, not a general ability to break cryptographic algorithms, so classical cryptographic systems remain unaffected for now.
The gap between this research prototype and a cryptographically relevant quantum computer is vast. Qubit count is a fundamental limiter: with 105 physical qubits, Zuchongzhi 3.0 falls far short of the thousands of stable, logical qubits needed to run complex algorithms like Shor’s on real keys. Moreover, its breakthrough was achieved using only 83 of those qubits for the demonstration (to optimize fidelity). Equally important are error rates. While the processor boasts high fidelity (single-qubit gate ~99.9% and two-qubit ~99.6% fidelity), a 0.4% error per two-qubit operation is still huge when algorithms require thousands of sequential operations. Lack of fault tolerance means these errors accumulate. Zuchongzhi 3.0, like other NISQ-era devices, has no quantum error correction, so even minor noise can corrupt a long calculation. Errors in multi-qubit operations remain a hurdle as circuits grow in complexity. Significant advances in error correction and qubit stability are required before any quantum processor can execute the deep, precise circuits needed for cryptanalysis. In its current form, Zuchongzhi 3.0 is a powerful experimental device, but it cannot run the lengthy, complex computations required to brute-force passwords or factor large keys.
Outlook
Zuchongzhi 3.0’s success brings optimism that quantum computing is steadily moving from laboratory curiosity to a transformative technology. Each incremental improvement – a few more qubits, a bit more fidelity, a better error correction code – expands the class of problems that can be attempted. While still in early days, the pace of progress is encouraging. In 2019, 50 qubits was the frontier; by 2025, 100+ qubits with decent fidelity are operational, and error correction is starting to work. If this pace continues, we could see commercially relevant quantum advantage (e.g. a quantum computer doing a financial risk calculation or material design faster than a classical supercomputer) within the next several years. Industries such as pharmaceuticals, finance, logistics, and materials science are watching closely. Some have begun partnering with quantum tech companies to develop algorithms so that they are “quantum-ready” when the hardware matures.