AWS Announces Ocelot Chip for Ultra-Reliable Qubits
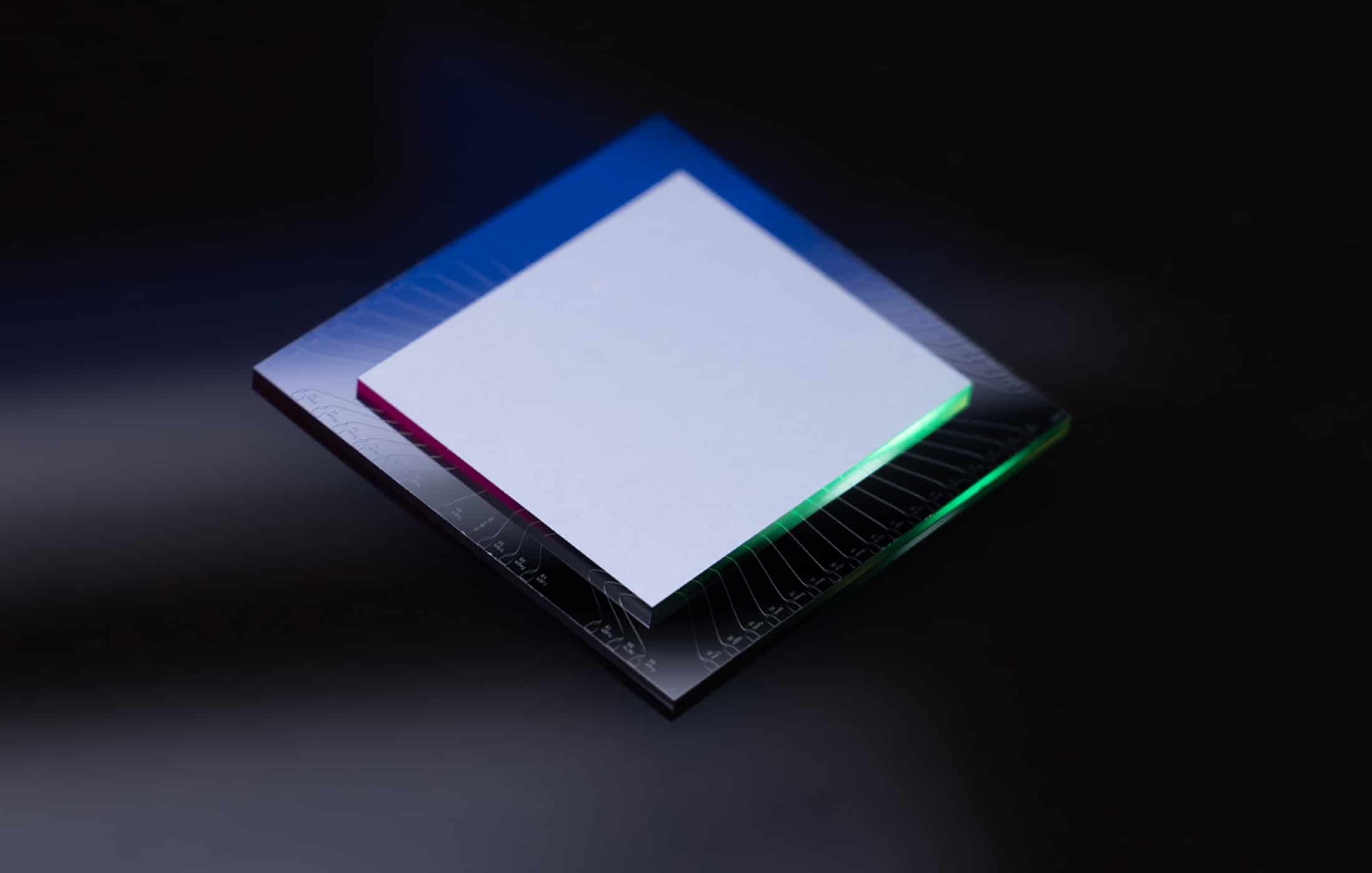
Table of Contents
Amazon Web Services (AWS) has officially unveiled Ocelot, its first in-house quantum computing chip, marking a significant milestone in the company’s quantum ambitions. Announced on February 27, 2025, Ocelot is a prototype processor designed from the ground up to tackle quantum error correction in a more resource-efficient way. AWS claims the new chip can reduce the overhead (and thus cost) of error correction by up to 90% compared to current methods. Developed at the AWS Center for Quantum Computing (on Caltech’s campus), Ocelot is described as a breakthrough toward building fault-tolerant quantum computers – machines that could one day solve problems “beyond the reach” of today’s classical supercomputers. This announcement positions AWS alongside other tech giants in the race for quantum computing, but with a distinct focus on error-corrected quantum hardware from the outset. AWS’s Director of Quantum Hardware, Oskar Painter, emphasized that with recent advances, “it is no longer a matter of if, but when” practical quantum computers arrive, calling Ocelot “an important step on that journey.” He noted that chips built on Ocelot’s architecture could be produced at roughly one-fifth the cost of current approaches, potentially accelerating AWS’s timeline to a practical quantum computer by up to five years.
Ocelot’s debut is especially significant given AWS’s broader quantum computing strategy to date. Until now, AWS’s public quantum efforts have centered on Amazon Braket, a cloud service launched in 2019 that lets users experiment with quantum algorithms on third-party quantum hardware. Through Braket, researchers can access a range of quantum technologies (from superconducting qubits to ion traps and photonic devices) provided by AWS partners. In other words, AWS has so far acted as a quantum cloud platform, aggregating others’ quantum processors (e.g. D-Wave’s annealers, IonQ’s ion traps, Rigetti’s superconducting qubits) for customers to use. The development of Ocelot suggests a shift – AWS is moving from solely hosting external quantum machines to building its own. Rather than immediately aiming for a high qubit count or near-term applications, AWS invested in a long-term research effort (in collaboration with Caltech) to create a chip that fundamentally improves reliability and scalability. Ocelot thus complements AWS’s existing quantum services by seeding the AWS ecosystem with proprietary hardware innovations. (Notably, AWS has also been investing in quantum networking R&D and other initiatives, underlining that Ocelot is part of a broader, multi-pronged quantum program.) With the Ocelot prototype’s results now published in Nature, AWS is signaling that it intends to be a major player in quantum computing not just as a cloud provider, but as a hardware innovator driving the technology forward.
Technical Breakdown of Ocelot
Qubit Technology
Ocelot is built on superconducting quantum circuits, but with a twist: it uses bosonic “cat qubits” as the fundamental units of quantum information. A cat qubit isn’t a simple two-level system like a standard transmon qubit; instead, it encodes a qubit into two coherent states of a quantum oscillator (analogous to Schrödinger’s cat being “alive” and “dead” at once). In practice, this means Ocelot’s qubits are realized in high-quality microwave resonators (oscillators) on the chip, which can occupy quantum superpositions of two opposite-phase states. These oscillator-based qubits are coupled to conventional superconducting transmon qubits that act as auxiliaries for control and readout.
Ocelot’s prototype consists of five cat qubits (the main data qubits for computation) and four transmon ancilla qubits interleaved among them, along with nonlinear coupling circuits called “buffers” that stabilize the cat states. In total, the chip incorporates nine quantum bits (5 data + 4 ancilla) spread across two bonded silicon dies about 1 cm² in size. (Each cat qubit’s resonator is made from superconducting tantalum film, and AWS developed a special processing technique to maximize its quality factor.) The ancilla transmons are used to entangle with and measure the cat qubits, but importantly, the quantum information is stored in the cat qubits’ oscillator states. This hybrid approach – bosonic qubits stabilized by superconducting circuits – lies at the heart of Ocelot’s technical innovation.
Error Suppression and QEC Architecture
The motivation for using cat qubits is their intrinsic error resilience. In a cat qubit, the logical 0 and 1 states are represented by two distinct oscillator states (often, even vs. odd photon-number superpositions). Flipping between these logical states (bit-flip error) is not a simple single-particle error; it requires a large change in the oscillator’s state. In fact, increasing the number of photons in the oscillator makes bit-flip errors exponentially rare. AWS capitalized on this property: by biasing the qubit’s noise towards one type of error, Ocelot can essentially “freeze out” bit-flips. The team reported physical bit-flip error times approaching one second, which is orders of magnitude longer than in ordinary superconducting qubits. (For comparison, a typical transmon qubit’s coherence time might be on the order of 50–100 microseconds.) This means a cat qubit can hold its state (with respect to bit flips) for nearly a second before random flips occur – a huge stability boost. The trade-off is that the other error channel, phase-flip errors (where the relative phase of the cat state flips), still occurs more frequently – on the order of tens of microseconds for Ocelot (about 20 µs reported). To handle these phase errors, AWS built a simple quantum error correction (QEC) code into Ocelot’s architecture. Specifically, the five cat qubits are arranged in a linear repetition code: by entangling and encoding information across multiple cat qubits, Ocelot can detect and correct if one of them experiences a phase flip. The four transmon ancillas are used to perform multi-qubit measurements (via controlled-NOT operations) that flag which cat qubit, if any, suffered a phase error. Notably, AWS implemented a “noise-biased” CNOT gate between each cat qubit and an ancilla, designed to preserve the cat’s bit-flip protection while enabling phase error detection. This is reported as the first-ever demonstration of a bias-preserving gate – a crucial step, since a poorly designed gate could introduce unwanted bit-flip errors and ruin the advantage of the cat qubits. In summary, Ocelot’s error correction approach is two-layered: at the hardware level, each cat qubit suppresses bit-flips on its own, and at the logical level, a simple code (comparable to a repetition code) catches phase-flip errors using redundancy across qubits.
Operating Ocelot still requires the standard quantum computing setup of cryogenic isolation. The chip resides in a dilution refrigerator at temperatures close to 10 millikelvin (near absolute zero) to minimize thermal noise. Like all superconducting qubit devices, Ocelot is highly sensitive to environmental disturbances – stray electromagnetic fields, vibrations, or cosmic rays can induce errors. The design’s advantage is that many of those disturbances would manifest as bit-flip errors, which the cat qubits largely suppress. This means Ocelot’s qubits can be more stable against certain noise without expending extra resources on error correction at every moment. Still, phase errors and other imperfections remain, so the system continuously runs error-correction cycles to maintain the logical quantum state.
In testing, AWS researchers ran sequences of error-correction cycles on Ocelot to gauge its performance as a quantum memory. They demonstrated that using a distance-5 code (i.e. five cat qubits encoding one logical qubit) significantly lowered the logical error rate compared to a smaller distance-3 code (three cat qubits). In fact, when looking at just phase-flip errors, the larger code showed the expected exponential suppression with increasing distance. When all error channels were considered, the team measured a total logical error rate of ~1.7% per cycle at distance-3, improving to ~1.65% at distance-5. This modest improvement in the overall error rate indicates that Ocelot’s built-in bias was effective – even though the distance-5 code had more physical qubits that could potentially fail, the strong suppression of bit-flips kept the logical error essentially flat (slightly better, in fact). Crucially, Ocelot achieved the distance-5 logical qubit using only 9 physical qubits, whereas a standard surface code would require 5×5 = 25 data qubits plus 24 ancilla (49 qubits total) to reach distance-5. In other words, AWS’s chip achieved with 9 qubits what would normally demand 5 times as many – a striking validation of the hardware-efficient approach.
This result aligns with AWS’s projection that the cat-qubit architecture can cut the number of physical qubits per logical qubit by a factor of about 5–10 in general. Indeed, a forthcoming AWS research paper analyzes how scaling up Ocelot’s approach could reduce error-correction overhead by up to 90% relative to conventional surface-code methods.
Comparison with Other Quantum Chips
In terms of raw qubit count and conventional performance metrics, Ocelot is a small device. Competing superconducting processors by IBM and Google contain one or two orders of magnitude more qubits. For instance, IBM’s 127-qubit Eagle processor (2021) and 433-qubit Osprey (2022) greatly surpass Ocelot’s qubit count, and IBM recently introduced Condor – a 1,121-qubit quantum processor – as the first to break the 1000-qubit barrier. Google’s Sycamore chip, famous for a 53-qubit quantum supremacy experiment, has likewise been succeeded by larger prototypes (Google has tested 72-qubit and 100+ qubit devices in its labs). However, those systems do not yet natively incorporate error correction – they operate in the so-called NISQ (noisy intermediate-scale quantum) regime, where computations are limited by short qubit lifetimes and high error rates.
By contrast, AWS chose to build Ocelot with error mitigation as the top priority, even at the expense of qubit count. This means a direct spec sheet comparison is somewhat misleading: Ocelot’s nine physical qubits can act as one robust logical qubit, something that IBM or Google chips would need dozens of physical qubits to emulate. In a 2023 experiment, for example, Google researchers used a 49-qubit array to encode a single logical qubit with a distance-5 surface code (and observed a slight error reduction vs. a smaller code). Ocelot achieves a similar logical qubit with 9 qubits thanks to the cat architecture.
Another way to compare is via coherence times: Ocelot’s effective bit-flip lifetime (~1 s) is about 1000× longer than the coherence times of IBM’s transmon qubits (typically ~0.1–0.2 ms) – a dramatic improvement in one error dimension. Its phase-flip time (~20 µs) is shorter than the best transmons (which can have T2 in the 50–100 µs range), but Ocelot compensates by actively correcting those phase errors. In essence, AWS traded hardware complexity for error longevity. The strength of Ocelot’s approach is that it can reach the low error rates needed for scaling without brute-force replication of qubits: it relies on more efficient qubits. The limitation is that Ocelot currently is only a single logical qubit demonstrator – it doesn’t perform multi-qubit algorithms yet – whereas chips from IBM and Google have executed small quantum programs (with errors) and demonstrated two-qubit gate operations across dozens of qubits.
It’s worth noting that AWS isn’t alone in pursuing error reduction at the qubit design level. IBM, for instance, has been improving the quality of its qubits alongside increasing quantity – their newer 156-qubit Heron processor uses improved materials and tunable couplers to boost fidelity, achieving 3–5× better performance than the earlier Eagle chip. IBM’s roadmap envisions combining large qubit counts with error mitigation techniques to approach “quantum utility,” the point where quantum computers do useful work beyond experiments. Google’s team similarly has focused on scaling and error correction: after demonstrating a logical qubit with 49 physical qubits (distance-5), they improved their chip and in 2024 managed to cut the logical error rate in half by moving to a 72-qubit system (distance-5 vs. distance-3). These efforts, however, still involve a lot of overhead (many physical qubits per logical qubit). AWS’s Ocelot leapfrogs in efficiency, at least at the small scale, by baking redundancy into each qubit. Microsoft is taking yet another route – trying to create qubits that are inherently stable via topological physics. Microsoft has been researching Majorana-based qubits, where quantum information would be stored non-locally in exotic quasiparticles, theoretically making them immune to certain errors. Just days before AWS’s Ocelot debut, Microsoft announced progress toward a so-called “Majorana qubit” (sometimes nicknamed Majorana 1), claiming evidence that its approach is getting closer to producing a functional qubit. This approach is very different from AWS’s. Ocelot is essentially a more conventional superconducting chip, similar to ones developed by Google and IBM, whereas Microsoft’s topological chip is a fundamentally new paradigm. It’s too early to say which approach will win out – Microsoft’s topological qubit could, in theory, eliminate a lot of error correction overhead if it works, but it remains unproven in a multi-qubit setting. By contrast, AWS has something tangible in Ocelot, leveraging well-understood superconducting circuit tech with a clever twist. As AWS’s Oskar Painter put it, “the biggest challenge isn’t just building more qubits… It’s making them work reliably.” Ocelot embodies that philosophy by focusing on reliability per qubit.
In summary, Ocelot’s capabilities stand out for their error-corrected design and noise biasing, while its limitations are that it’s a first-generation prototype (with only one logical qubit realized so far). IBM and Google currently have the upper hand in raw qubit counts and have demonstrated small quantum computations, but they are still grappling with error rates that are “a billion times” too high for useful algorithms. AWS’s bet is that by solving error correction now at the qubit level, it can catch up in the long run with far fewer qubits. The competitive landscape thus presents a trade-off: AWS is pursuing long-term scalability at the expense of short-term capabilities, whereas others have shown more immediate computing demonstrations but will face scalability challenges as they attempt to error-correct those larger systems.
AWS’s Broader Quantum Strategy
AWS’s journey with quantum computing started not with hardware, but with cloud services and partnerships. Amazon Braket, launched in late 2019, is AWS’s managed quantum computing service that gives users access to quantum processors from multiple hardware providers via the cloud. Using Braket, a developer can run experiments on devices like IonQ’s trapped-ion computers, Rigetti’s superconducting qubits, or even analog quantum annealers, without owning any quantum hardware. This cloud-centric strategy has positioned AWS as a facilitator in the quantum ecosystem – essentially, AWS became the platform where emerging quantum technologies are made available to researchers, much as AWS’s EC2 service did for classical computing. In parallel, AWS established dedicated research centers to advance quantum technology behind the scenes. The AWS Center for Quantum Computing at Caltech (opened around 2020) and the AWS Center for Quantum Networking (launched in 2021 in Boston) were clear signals that Amazon was investing in foundational quantum R&D. The fruits of these investments are now becoming visible with Ocelot’s debut.
By unveiling Ocelot, AWS is shifting from solely a quantum cloud provider to also a quantum hardware developer. This doesn’t mean AWS will stop supporting other hardware on Braket – on the contrary, Braket’s value is in offering diverse architectures to customers. But it means AWS is no longer content to just host others’ innovations; it wants to innovate itself. Ocelot is a proof-of-concept that AWS’s internal research can produce leading-edge results. It also strategically positions AWS to have proprietary technology in quantum computing. We’ve seen a similar pattern in classical cloud computing: AWS developed its own silicon (e.g. custom CPUs like Graviton and AI chips like Trainium) to improve performance and differentiation in the cloud. Now AWS seems to be following that playbook in quantum. “We looked at how others were approaching quantum error correction and decided to select our qubit and architecture with quantum error correction as the top requirement. We believe that if we’re going to make practical quantum computers, QEC needs to come first,” said AWS’s quantum hardware team in explaining their approach. This is a notable departure from the typical approach of building a big quantum chip first and worrying about error correction later. By engineering error tolerance into the hardware (via cat qubits), AWS hopes to leapfrog some of the scalability issues that competitors will face. In effect, AWS’s broader strategy is to attack the hardest problem—error correction—up front, even if it means the first device has a “rudimentary” computational capability. Ocelot, as AWS admits, has only a tiny fraction of the computing power needed for useful quantum applications. Yet it is meant to prove a point: that AWS’s chosen architecture is viable and can be scaled into a larger, fault-tolerant machine.
Alongside hardware development, AWS continues to enhance its quantum services. Amazon Braket regularly adds new partner machines (for example, adding a photonic quantum computer from Xanadu in late 2022, and devices from QuEra for neutral-atom computing). AWS also offers the Amazon Quantum Solutions Lab, which is a consultancy service to help businesses explore quantum algorithms, and it provides high-performance quantum circuit simulators on its cloud for development and testing. All these pieces fit into AWS’s long-term plan: be the go-to platform for quantum computing, whether via others’ hardware or eventually its own. With Ocelot, AWS is indicating that its future platform might feature Amazon’s home-grown quantum processors sitting alongside the likes of IBM’s or IonQ’s, accessible on demand. It also suggests AWS is aiming to be at the forefront of quantum hardware innovation, not just a reseller. If Ocelot’s approach proves out, AWS could become a leader in the race to build a fault-tolerant quantum computer, potentially offering quantum hardware as a cloud service (much as it offers EC2 instances) in the coming years. In the meantime, AWS is leveraging partnerships to stay relevant: by working with academic institutions (Caltech, Harvard, etc.), government research programs, and quantum startups (through partnerships and possibly funding), AWS ensures it’s plugged into developments across the quantum field. The broader strategy is clear – invest now for a long-term pay-off.
As Gartner’s hype cycle and history have shown, quantum computing is a marathon, not a sprint. AWS is preparing its arsenal (cloud infrastructure, research talent, and now proprietary hardware like Ocelot) to position itself as a central player when quantum computing reaches maturity. In summary, Ocelot contributes to AWS’s quantum vision by giving it a unique technological stake. It’s a first step toward AWS being not just the “AWS of quantum” in terms of cloud access, but also a creator of quantum technology that others might access through AWS. This dual role – hardware innovator and cloud provider – could eventually make AWS a one-stop shop for quantum solutions, similar to its dominance in classical cloud computing.
Industry Comparisons and Competitive Landscape
The quantum computing landscape is increasingly crowded with tech giants and startups, each pursuing different hardware approaches. AWS’s introduction of Ocelot comes on the heels of major announcements from IBM, Google, and Microsoft, among others, and inevitably invites comparison.
IBM: IBM has been a front-runner in superconducting quantum computers for years. Its strategy has emphasized scaling up the number of qubits while incrementally improving their quality. In 2021 IBM unveiled Eagle (127 qubits), in 2022 it showed Osprey (433 qubits), and by late 2023 it announced Condor, the world’s first 1,121-qubit quantum processor. These chips are based on IBM’s transmon qubit technology and a heavy-hexagon lattice topology to reduce error crosstalk. IBM’s roadmap anticipates crossing the 1000-qubit mark as a stepping stone to eventually building million-qubit-level systems capable of error correction. However, IBM has not yet demonstrated a fully error-corrected logical qubit on these large processors. Instead, they have been focusing on shorter-term “quantum advantage” experiments and developing an ecosystem (Qiskit software, cloud access, etc.) around their hardware. IBM’s approach to error correction is currently centered on software and compiler optimizations, alongside plans to implement the surface code in the future. In contrast, AWS’s Ocelot took a very different tack: starting with a small number of qubits but making them error-resilient by design. IBM’s 127 or 433 qubit machines can execute algorithms that Ocelot’s 9 qubits cannot (simply due to qubit count), but those algorithms are limited by noise and quickly decohere. Ocelot can’t yet run a meaningful algorithm, but the single logical qubit it represents is far more stable against errors. In essence, AWS is playing a long game versus IBM’s broader short-to-mid-term approach. It’s interesting to note that if IBM wanted to achieve the same kind of logical qubit Ocelot has, using its current method (surface code), IBM would have to sacrifice dozens of its physical qubits for just one logical qubit. Indeed, estimates for the surface code suggest that thousands of physical qubits might be needed for one high-quality logical qubit (depending on error rates). This is why IBM’s vision involves millions of physical qubits for a full fault-tolerant machine. AWS is attempting to shrink that ratio drastically with its cat-qubit architecture. That said, IBM holds an advantage in engineering experience – they have learned to control hundreds of qubits on a single chip, developed cryogenic packaging and wiring for large systems, and built a robust software stack. AWS will need to catch up on those fronts as it scales Ocelot beyond the lab. From a competitive positioning standpoint, IBM might appear “ahead” in hardware maturity and ecosystem, but AWS is aiming to leapfrog a key barrier (error correction overhead) that IBM still must surmount. If Ocelot’s approach proves scalable, AWS could potentially achieve a useful fault-tolerant processor with far fewer qubits than IBM’s brute-force approach would require. It effectively puts AWS and IBM on diverging trajectories: one focusing on quantity of qubits, the other on quality of qubits.
Google: Google’s quantum effort (within Google Quantum AI) also uses superconducting qubits and is comparable to IBM’s in many respects. Google famously demonstrated “quantum supremacy” in 2019 with its 53-qubit Sycamore chip, showing a task that a classical supercomputer would struggle to simulate. Since then, Google’s focus shifted strongly to quantum error correction experiments. In 2021, Google showed the first experimental evidence that increasing the size of a surface code (from distance-3 to distance-5) can suppress logical error rates – a crucial milestone. That experiment involved 17 physical qubits for distance-3 and 49 qubits for distance-5 (including data and ancilla qubits), and the larger code achieved a slightly lower error rate than the smaller, indicating they were operating below the error-correction threshold. More recently, in 2023–2024, Google improved their hardware and demonstrated a ~50% reduction in logical error rate when moving to a distance-5 code on a new 72-qubit chip. These are remarkable achievements, showing that Google is incrementally approaching fault-tolerance, but they also highlight how resource-intensive the standard approach is – dozens of qubits to protect one qubit, and only marginal gains so far. Enter AWS Ocelot: by using a different QEC scheme (bosonic codes), AWS achieved a comparable distance-5 logical qubit with just 9 qubits. In principle, this puts AWS in the same ballpark as Google in error correction progress, but with far fewer physical qubits. However, Google’s systems can do more than just quantum memory; Google has run small algorithms and two-qubit gates across its chips (for example, simulating simple chemical reactions or running optimization problems on Sycamore). Ocelot, as a new prototype, has not yet demonstrated any algorithm or multi-logical-qubit operation – it was used to store quantum information and test QEC cycles. So Google currently leads in system integration and algorithmic demonstrations, whereas AWS leads in error correction efficiency per qubit. Another point of comparison: Google and AWS both recognize the importance of error mitigation – Google has invested in better qubit materials, AI-assisted error decoders, and is exploring bosonic qubits as well in research (Google has experimented with cat states in cavities, though not as extensively integrated as AWS has now done). It wouldn’t be surprising if Google takes note of AWS’s success and accelerates its own efforts on bosonic or bias-preserving qubits. In the competitive landscape, Google and AWS have a bit of a shared goal: reaching fault-tolerance. Google started earlier and chose the conventional route (surface code on transmons), AWS started later but chose a novel route (cat code on resonators). Both have published in Nature showing improved logical qubit performance. The race will come down to who can scale further without encountering insurmountable engineering roadblocks.
Microsoft: Microsoft’s approach stands apart from both the superconducting camp (IBM/Google/AWS) and other modalities. They are pursuing topological quantum computing, attempting to harness Majorana zero modes in superconductors as qubits. In theory, a topological qubit could store quantum information in a way that is intrinsically protected from local sources of error (leveraging the mathematics of topology). For over a decade, Microsoft has invested in this approach, but progress was slow and at times questioned. In late 2022 and into 2023, Microsoft announced evidence that they had created the necessary conditions for Majorana modes and were on track to demonstrate a topological qubit. In January 2025 (just a month prior to Ocelot’s reveal), Microsoft reportedly unveiled a prototype dubbed “Majorana 1”, claiming a significant step toward a qubit that could be scaled to millions through topological protection (this was widely covered in tech media). If one took Microsoft’s optimistic view, their qubit could eventually allow a quantum computer with far fewer physical qubits for the same computation – because each qubit would be far more stable (perhaps needing only 10-50 physical Majoranas per logical qubit, rather than thousands of transmons). However, as of now, Microsoft has not yet shown a functional logical qubit or algorithm with their approach. An outside expert comparing AWS and Microsoft summed it up well: Ocelot is built on “more conventional superconducting” technology (just used in a clever way), while Microsoft’s is a very different concept altogether. From a competitive standpoint, AWS and Microsoft are almost in opposite corners: AWS took an existing platform (superconducting circuits) and improved it incrementally, delivering a working prototype; Microsoft is trying for a leap to a potentially revolutionary qubit, but still in the physics experimentation stage. Each carries risk – Microsoft’s risk is that topological qubits might take much longer (or might never pan out as hoped), while AWS’s risk (shared with IBM/Google) is that even with improvements, error correction could remain extremely challenging and resource-intensive. If Microsoft’s approach suddenly succeeded, it could render a lot of the current incremental approaches obsolete (since a topological qubit would reduce the need for complex error-correcting schemes). On the other hand, if Microsoft’s approach falters or takes another decade, AWS and others will continue making headway with what’s available now.
Unique Advantages vs. Catching Up: Does Ocelot give AWS a unique edge, or is AWS playing catch-up in the quantum race? The answer is a bit of both. In terms of unique advantage, Ocelot clearly sets AWS apart by demonstrating a hardware-efficient error correction in practice. No other major player has shown a logical qubit with as few resources or a gate that preserves noise bias as AWS has done here. This positions AWS as an innovator in quantum error correction – potentially a critical advantage, since error correction is the obstacle to scaling quantum computers. If AWS can maintain this 5×–10× hardware efficiency as they scale up, they could achieve fault-tolerant computing with far fewer qubits than anyone else, translating to less complex machinery and possibly faster deployment of a useful quantum computer. It’s a bit like a space company finding a way to reach orbit with a much smaller rocket – it changes the economics and feasibility of the mission. Additionally, AWS’s deep expertise in cloud infrastructure could allow it to tightly integrate any quantum hardware it develops with classical computing resources, enabling a powerful hybrid computing environment. On the other hand, AWS is indeed playing catch-up in several respects. Companies like IBM and Google have been building quantum processors for a decade and have solved many practical engineering problems (packaging, I/O, fabrication scaling) that AWS is only now encountering with Ocelot’s scaling. Those companies also have a head start in terms of offering quantum services to users (IBM’s Quantum Experience has run billions of quantum circuits from external users, giving them tons of data on performance). Until Ocelot, AWS was mostly leveraging others’ hardware for Braket – which meant AWS didn’t have to solve hardware problems, but also didn’t develop that hardware know-how in-house at scale. Now that is changing, but it will take time for AWS to mature its hardware. In the immediate term, AWS’s 9-qubit prototype can’t perform useful computations, whereas IBM’s 127-qubit machine, while noisy, can at least run small instances of quantum algorithms or be used for prototyping algorithms. One might say AWS voluntarily stepped back on qubit count in order to leap forward on error correction. It’s a calculated trade-off: short-term lag for long-term lead. The quantum computing race is not just about who has the most qubits in 2025; it’s about who can get to a scalable, reliable quantum computer first. From that perspective, AWS has put a stake in the ground that it’s pursuing a different path. It’s also worth noting that AWS’s entry with Ocelot signals a broader shift: cloud providers are becoming hardware players. Just as Google built its own quantum hardware and IBM offers cloud access to its quantum hardware, AWS is now committing to its own hardware development. This blurs the line between the competitors – we might soon see AWS not only hosting third-party QPUs but competing directly with those third parties with its own QPU offerings. In fact, Ocelot suggests AWS is no longer content to “play catch-up” at all; it wants to define the cutting edge. Time will tell if Ocelot’s unique technical approach will yield a decisive advantage or if others will incorporate similar ideas. For now, AWS has made a bold move that distinguishes its strategy in the quantum arena.
Cryptographic and Security Implications
Every advance in quantum computing raises the question: are we getting closer to quantum machines capable of breaking modern cryptography? Ocelot, as impressive as it is for error correction research, is still a long way from posing a threat to encryption. It contains effectively one logical qubit; by contrast, breaking RSA or similar cryptographic systems via Shor’s algorithm would require on the order of thousands of logical qubits operating reliably for millions (or more) of quantum gate operations. The consensus among experts is that we are not at the era of cryptographically relevant quantum computers (CRQCs) yet – but we are steadily working towards it. In fact, Ocelot’s very purpose is to overcome the scalability obstacles that currently stand between today’s quantum prototypes and tomorrow’s powerful machines. AWS’s successful demonstration of more efficient error correction does inch the field closer to the day when a quantum computer could threaten cryptography. By potentially accelerating the timeline to a fault-tolerant quantum computer by a few years, Ocelot shortens the window in which our current encryption remains absolutely safe.
To put things in perspective, Oskar Painter (AWS/Caltech) noted that quantum error rates need to improve by about a billion-fold to reach the level required for useful algorithms like Shor’s factoring. At the recent pace of improvement (a rough doubling of coherence every couple of years), it might have taken many decades to get there. Approaches like Ocelot aim to dramatically speed up that progress by attacking the problem structurally. If Ocelot’s architecture (or similar schemes) can cut down the qubit overhead by 90%, as claimed, that directly impacts how quickly a CRQC could be built. Fewer physical qubits needed per logical qubit means fewer total qubits for a given task, less complexity, and likely a shorter path to algorithms like Shor running successfully. It’s telling that AWS explicitly framed Ocelot as a step towards “real-world applications” and mentioned accelerating timelines – code words that include cryptographically relevant applications in their scope.
So, should governments and enterprises be alarmed by Ocelot and hasten their transition to post-quantum cryptography (PQC)? The prudent view is that PQC migration should continue with urgency, not because Ocelot by itself changes the game overnight, but because it reinforces that the game is being changed steadily and perhaps faster than some expected. As of a 2023 survey, most experts believed the chance of a CRQC appearing in the next 5 years was very low, but by 15–20 years out, a majority saw a significant possibility of such a machine existing. Developments like Ocelot support those longer-term probabilities – they show concrete progress toward making quantum computers more viable. In response to the expected threat, standards bodies have been proactive. In 2022, the U.S. National Institute of Standards and Technology (NIST) selected several post-quantum encryption algorithms to replace RSA and ECC, and in 2024 NIST issued guidelines recommending that by 2035 all systems transition to quantum-resistant cryptography. That 2035 date is not arbitrary; it roughly aligns with expert forecasts of when quantum attacks could become feasible. NIST’s timeline effectively assumes that by the early-to-mid 2030s, a cryptographically relevant quantum computer might be operational. The unveiling of Ocelot, along with recent strides by Google and others, lends credence to the notion that the 2030s could indeed see such capabilities. Importantly, even if the first CRQC doesn’t appear until, say, 2040, the threat to today’s data is already present in the form of “harvest-now, decrypt-later” attacks. Adversaries could record encrypted communications now and decrypt them once a quantum computer is available. This means that sensitive data – government secrets, personal health information, financial records – that must remain confidential for decades should be protected with quantum-resistant algorithms as soon as possible.
Ocelot’s advancements underscore that there’s no fundamental physics barrier stopping quantum computers from improving; it’s a hard engineering problem that is being actively solved. Each breakthrough (like better error rates or a new scalable qubit design) should serve as a reminder that a CRQC is on the horizon, even if that horizon is years away. In the case of AWS, a company with vast cloud infrastructure and services used by enterprises worldwide, one can imagine that once AWS has a powerful quantum computer, it might offer “quantum-as-a-service.” This could be a boon for customers wanting to solve complex problems – and a nightmare for anyone relying on pre-PQC cryptography. Therefore, enterprises should treat the advent of devices like Ocelot as a timely prompt to accelerate their post-quantum transition plans. Many organizations (financial institutions, tech companies, etc.) have already begun evaluating PQC algorithms and planning upgrades, often following government directives. For example, the U.S. government’s Cryptographic Modernization program mandates agencies to inventory cryptographic systems and begin adopting PQC in the next few years. These efforts need to continue in earnest.
In summary, Ocelot itself cannot crack your RSA encryption – it’s not even close. But it is a harbinger of the rapidly evolving quantum technology that will, in time, render current cryptography obsolete. The wise course for security professionals is to stay ahead of that curve. The timeline for PQC deployment should be measured in years, not decades, given that the timeline for a potential CRQC is now commonly estimated at within two decades or sooner. AWS’s achievement should thus be taken as validation that the quantum computing community is overcoming obstacles on the road to a CRQC. Entities that haven’t yet begun preparing for a post-quantum world may want to start, and those already on the path have more reason to continue without delay. The cost of procrastination could be very high in terms of security. As one might say, the quantum clock is ticking – and Ocelot just moved the hands forward a bit.
Future Outlook and Challenges
AWS’s Ocelot is an exciting proof-of-concept, but significant challenges remain if AWS hopes to build a large-scale quantum computer. First, scaling from a single logical qubit to many is non-trivial. Ocelot is still an early-stage prototype – essentially a quantum memory demonstrating error correction principles. To perform useful computations, AWS will need multiple logical qubits that can interact (entangle) with each other. That means scaling up the number of physical qubits substantially (dozens of cat qubits for a few logical qubits, then eventually hundreds, etc.) and implementing logical gates between them. Each additional qubit and each added gate increases the complexity and potential error sources. One immediate hurdle is engineering complexity: Ocelot’s design of 5 cat qubits and 4 ancillas already involves 14 resonator/qubit elements on chip. A next-generation device might double or triple that. As the number of components grows, so do cross-talk and calibration challenges. The analog nature of cat qubits (relying on oscillator modes) means controlling and reading out each one with precision. AWS will have to ensure that the error bias (the strong suppression of bit-flips) persists as the system scales – excessive coupling or noise could introduce new error modes that degrade the bias. Thermal management and control electronics are also considerations; more qubits mean more microwave control lines into the cryostat, and potentially the need for cryogenic logic to handle feedback for error correction in real-time.
Another challenge is maintaining performance at scale. Ocelot showed bit-flip times ~1 second on a small device. Will each cat qubit in a larger device still achieve that, or will larger systems see shorter coherence due to fabrication variability or interference? AWS will likely need to invest in even better materials (e.g., superconductors with fewer losses, extremely low-noise electronics) to keep the qubit quality high as quantity increases. The company has already noted that improvements in component performance will be part of their roadmap. For instance, increasing the photon number in cat qubits (to further suppress bit-flips) could be explored, but that might require more microwave drive power and could lead to diminishing returns or new instabilities. Error correction overhead, while reduced in Ocelot, doesn’t vanish – going from a 5-qubit code to larger codes (say 7 or 9 cat qubits for one logical) will further reduce logical error rates, but also means more physical qubits and more complex error syndrome extraction. AWS will need to ensure their bias-preserving gates and error detection schemes scale accordingly. They mentioned plans for “future versions of Ocelot” with increased code distance and improved components to exponentially drive down logical error rates, indicating they are aiming for even larger cat-qubit arrays and perhaps better noise biasing. Each increment in code distance (e.g., going to distance-7 with 7 cat qubits) should, in theory, suppress logical errors further, but only if physical errors are sufficiently low and uncorrelated. Achieving those conditions is an on-going battle in the lab.
One of the largest looming challenges is executing logical gates between two logical qubits. Thus far, Ocelot has shown it can maintain one logical qubit. A full quantum computer requires entangling logical qubits (for example, a logical CNOT between logical qubit A and B). Designing a logical gate that respects the noise bias (so as not to introduce too many bit-flip errors) will be crucial. It might involve coupling cat qubits from different logical groups or using additional ancillary systems. This could introduce a whole new layer of complexity. Other architectures have found that two-logical-qubit gates often require substantially more resources (for instance, connecting patches of surface code demands additional ancilla qubits and careful scheduling). AWS will have to invent a method to perform logical operations that doesn’t forfeit the benefits of the cat architecture. Perhaps they will use microwave photons to connect logical qubits, or incorporate tunable couplers that can temporarily link two cat qubit arrays. Whatever the approach, it will be a delicate task to implement without blowing up error rates. It’s possible AWS might demonstrate a basic logical-to-logical gate in a future paper as the next milestone.
Competition and integration: As AWS works on these technical challenges, so too will competitors be advancing. IBM plans to integrate error mitigation techniques and eventually error correction on its 1000+ qubit processors; Google is exploring new materials and even AI-designed pulses to extend coherence and reduce error rates. There’s also competition from entirely different technologies – ion trap quantum computers (from IonQ, Quantinuum, etc.) are also improving and have achieved fairly high-fidelity gates, and photonic quantum computers and neutral atom arrays are scaling up. While those may have different strengths (e.g., ion traps have very long coherence but slower gates), AWS will have to keep an eye on them. IonQ, for instance, aims to have modular trapped-ion systems that could reach many logical qubits by networking smaller units. If one of those approaches solves error correction in a simpler way, AWS’s bet on superconducting cat qubits would face stiff competition. That said, AWS can also incorporate those technologies via Braket – but from a hardware leadership perspective, AWS likely wants its own tech to be at the cutting edge.
One advantage AWS possesses is virtually unmatched cloud infrastructure and resources. Once the hardware is available, AWS can deploy it in its data centers and offer it globally via the cloud with relative ease (they already do this with other quantum machines on Braket). They can also combine quantum and classical resources seamlessly – for example, using AWS’s supercomputing for error correction decoding or for running parts of algorithms alongside the quantum part. This could mitigate some challenges; a powerful classical control system could handle complex error syndromes or calibrations on the fly. AWS is likely to leverage its expertise in scalable architectures (from data centers) to also manage a large quantum system’s operations. Painter has mentioned the concept of a “flywheel” of continuous improvement – integrating lessons from each scale-up back into the engineering cycle. This iterative approach, much like how AWS iterates on its cloud services, will be applied to quantum hardware development. We can expect several generations of Ocelot (or whatever feline code-names follow) in the coming years, each with more qubits and lower error rates. AWS explicitly states that Ocelot is their first chip with this architecture and that they have “several more stages of scaling to go through” in a multi-year roadmap.
In terms of long-term quantum ambitions, AWS is clearly aiming to become a leader in quantum computing, not just a participant. With Ocelot demonstrating a novel architecture, AWS is positioning itself at the forefront of quantum hardware research. If they can solve the next set of challenges, AWS could very well operate one of the first truly fault-tolerant quantum computers. Their approach is somewhat analogous to a startup within the company – start with a minimal viable product (a single logical qubit) and then scale aggressively. The encouraging results from Ocelot give AWS justification to pour more investment into this approach. We might see AWS hiring more quantum engineers and scientists (indeed, their Amazon Science article ends with a call for applicants interested in “joining us on this journey” ). Given Amazon’s vast resources, funding is unlikely to be an issue; the main question is time and technological hurdles.
AWS also has the flexibility to pivot or incorporate new ideas. If, say, topological qubits or spin qubits in silicon suddenly make a breakthrough, AWS could adjust its strategy – but for now, it appears committed to the superconducting bosonic route. In a way, AWS is hedging its bets by both partnering (through Braket) and innovating in-house (through Ocelot). This dual strategy means that even if one path faces a roadblock, AWS can leverage alternatives. But its ultimate goal will be to have proprietary technology that gives it an edge.
Looking ahead a few years, what might we expect from AWS? Possibly an “Ocelot-2” chip with maybe on the order of 20–30 qubits (allowing two logical qubits plus some ancillas) to demonstrate a logical two-qubit gate. Success there would be a huge milestone: it would mean AWS has a small fault-tolerant quantum processor, not just a memory. That could then be scaled into a larger multi-qubit system and integrated as a backend on Amazon Braket for select customers to experiment with. It’s conceivable that within, say, 5 years, AWS could offer a prototype error-corrected quantum computing service where users program logical qubits and the hardware handles the error correction underneath. Such a service would immediately differentiate AWS in the market – whereas competitors might offer more raw qubits that are noisy, AWS could offer fewer qubits but error-corrected ones that behave ideally for longer circuits. This is speculative, of course, and hinges on AWS conquering the technical mountains ahead.
In concluding, AWS’s road with Ocelot is both challenging and promising. They have made one small logical qubit for man, but it could turn into one giant leap for quantum-kind. Painter’s team acknowledges this is just “the beginning of a path to fault-tolerant quantum computation” and that continuous innovation and scaling will be needed. The next few years will test whether Ocelot’s design can indeed scale as envisioned. If it can, AWS might accelerate the arrival of useful quantum computers by years, helping to usher in the long-awaited quantum revolution. On the other hand, if unforeseen obstacles emerge (as they often do in cutting-edge tech), AWS will need to adapt – possibly exploring hybrid approaches (maybe combining cat qubits with other error-correction codes) or refining device fabrication further. Regardless, AWS has firmly established itself as a serious hardware contender with Ocelot, changing the perception that it was “just” providing quantum cloud access. The future outlook for AWS in quantum is an ambitious one: to go from this first chip to a scalable quantum computer that can tackle problems in chemistry, materials science, cryptography, and beyond. With Ocelot, AWS has taken a bold first step on that journey, entering a new phase where it will compete head-to-head with the likes of IBM, Google, and Microsoft in deep quantum technology. And if the promise of Ocelot is realized, AWS could very well help speed up the day when quantum computers transition from lab curiosities to indispensable tools – a day that the tech world, and indeed the security world, is watching closely.