Google’s Sycamore Achieves Quantum Supremacy
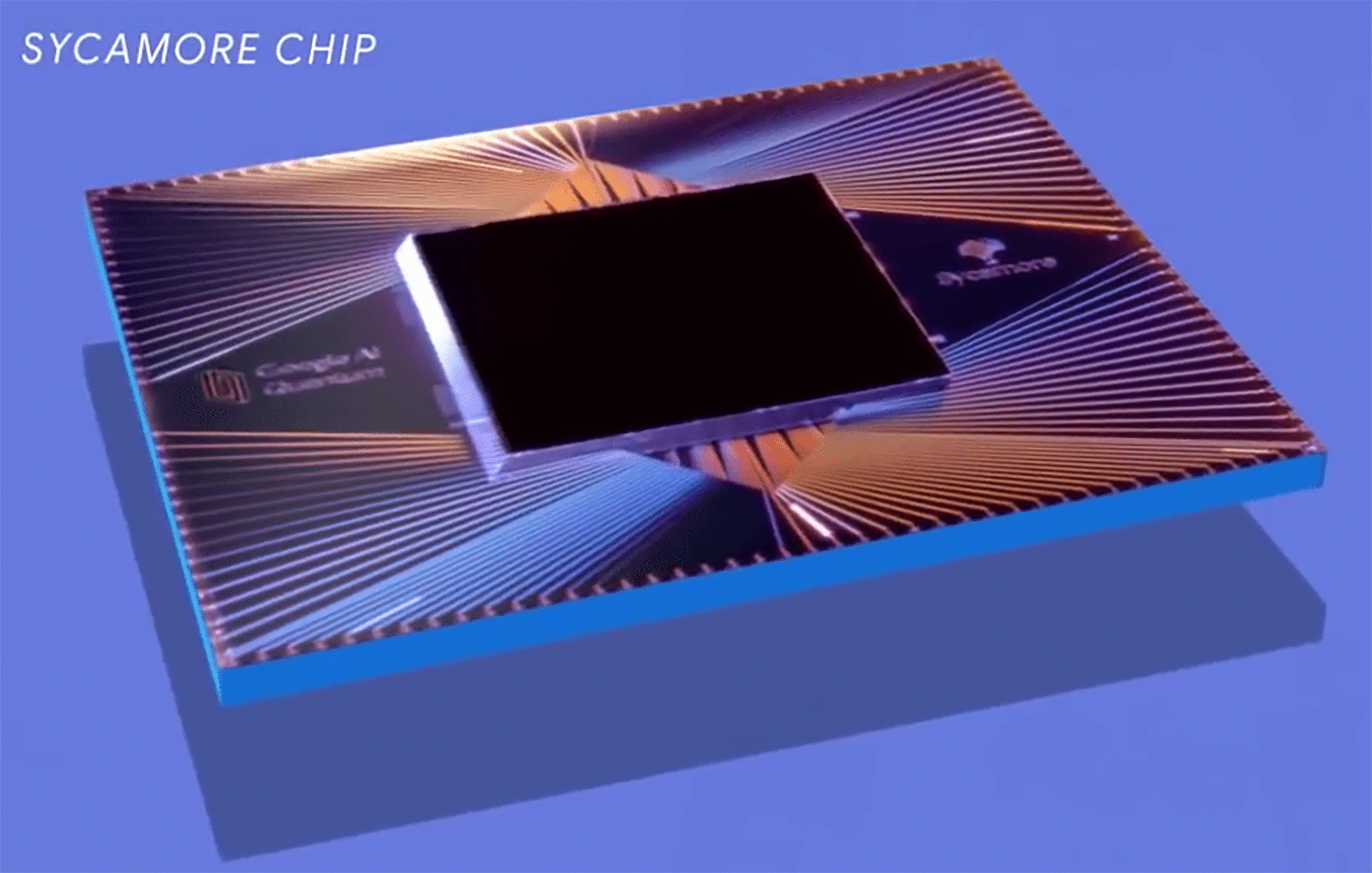
Table of Contents
Mountain View, CA, USA (Oct 2019) – Google announced that its 53-qubit quantum processor, Sycamore, has achieved a long-anticipated milestone known as “quantum supremacy.” In a paper published in Nature, the Google AI Quantum team reported that Sycamore performed a specific computation in approximately 200 seconds – a task they estimated would take the world’s fastest classical supercomputer at least 10,000 years to complete. This achievement marks the first time a quantum computer has outpaced classical computers for a real computational problem, heralding a new era in computing research. Researchers at NASA and Oak Ridge National Laboratory, who collaborated on benchmarking the feat, lauded the result as a “transformative achievement” demonstrating computation in seconds that would take “the largest and most advanced supercomputers” millennia.
Quantum Supremacy – a term coined by Caltech professor John Preskill in 2012 – refers to the moment a programmable quantum computer performs a calculation that no conventional computer can feasibly solve. Google’s experiment validated this concept by having Sycamore tackle a carefully chosen task: sampling the output of a random quantum circuit. In essence, the processor had to generate a set of one million bitstrings (random 53-bit numbers) with probabilities dictated by quantum interference, and do so faster than any known classical algorithm could simulate. The difficulty of this task grows exponentially with the number of qubits; even for the most powerful classical supercomputer (IBM’s 2019 Summit), direct simulation of Sycamore’s 53-qubit circuit is impractical. Google’s team estimated such a simulation might require $$2^{53}$$ computational states – about 10 quadrillion – making it “exponentially costly” in time and memory. By performing the computation in minutes, Sycamore effectively demonstrated the “quantum supremacy” threshold had been crossed for this specific problem.
What Was Achieved, and How?
Google’s Sycamore processor is based on superconducting transmon qubits, which are tiny resonant circuits kept at cryogenic temperatures to exhibit quantum behavior. Sycamore’s 54 qubits (one qubit was inactive during tests, leaving 53 in use) are laid out in a rectangular lattice with nearest-neighbor couplings. The team focused on executing a pseudo-random quantum circuit: a sequence of operations (single-qubit rotations and two-qubit entangling gates) applied across the chip in many layers. After about 20 cycles of these randomized gates, the chip’s quantum state becomes an entangled superposition of $$2^{53}$$ possible outcomes. Sycamore’s task was to sample from this complex output distribution and produce bitstrings that could be verified against classical simulations for smaller subsets of the circuit.
Crucially, Sycamore’s hardware was engineered for high fidelity in both single- and two-qubit operations. Google reported performing 1,113 single-qubit gates and 430 two-qubit gates in the largest circuits, with an expected total output fidelity of about 0.2%. While 0.2% might sound extremely low, it meant the quantum processor’s output had a small but measurable correlation with the correct quantum probability distribution. By collecting a million samples, the team could statistically verify that Sycamore was indeed producing the correct distribution of bitstrings (as opposed to just random noise). Achieving this required minimizing errors: each qubit’s operations had to be synchronized and calibrated so that the overall circuit remained coherent just long enough. Google’s researchers developed fast, simultaneous gate techniques and a rigorous validation method called cross-entropy benchmarking to ensure the whole chip performed as intended. The end result was that Sycamore could complete the sampling run in about 3 minutes and 20 seconds, whereas a state-of-the-art classical simulation was estimated (by Google) to take on the order of 10,000 years.
Google’s claim was met with both applause and some healthy skepticism in the scientific community. Notably, IBM – which operates its own 53-qubit superconducting processor in 2019 – argued that the task, while extremely hard, was not completely out of reach for classical computers. IBM researchers pointed out that by using clever algorithmic tricks and vast disk storage, one could simulate the same 53-qubit circuit in roughly 2.5 days on Summit, rather than thousands of years. They demonstrated that a simulation approach trading memory for computation (a hybrid “Schrödinger-Feynman” algorithm) could drastically cut down the time, calling Google’s 10,000-year estimate into question. By the strict definition Preskill proposed (quantum advantage that no classical computer can realistically replicate), IBM suggested the milestone “has not been met.” Google’s team acknowledged IBM’s improvement, but noted that even 2.5 days of running on a supercomputer (with petabytes of disk) is a far cry from 200 seconds – a ~1,000× speedup in favor of the quantum machine. In any case, the consensus is that Sycamore’s experiment is a landmark demonstration: it shows definitively that a programmable quantum device can outperform all known classical algorithms for a well-defined task, crossing a pivotal threshold in computing.
How Sycamore Compares to Earlier Quantum Computing Milestones
Google’s announcement builds on two decades of rapid progress in quantum computing. Before 2019, researchers worldwide had achieved impressive (if smaller-scale) feats on various quantum technologies. However, none had conclusively shown a beyond-classical computation until Sycamore. Here we compare Sycamore’s performance and approach to several major quantum computing efforts leading up to 2019:
IBM’s Superconducting Qubits
IBM has been a leader in superconducting quantum processors, using transmon qubits similar to Google’s. Years prior to Sycamore, IBM demonstrated steady growth in qubit count and quality – from a 5-qubit device put on the cloud in 2016 to a 16-qubit and then 20-qubit system by 2017. In late 2017, IBM unveiled a prototype 50-qubit processor, a scale long seen as a potential “quantum supremacy” threshold. These processors didn’t yet outperform classical computers on any task, but IBM introduced the concept of “quantum volume” as a holistic benchmark, factoring in qubit count, coherence time, connectivity, and gate fidelity. By 2019, IBM’s best systems achieved a Quantum Volume of 16 or higher (meaning they could reliably run 4×4-qubit circuits, for example) – a measure of incremental progress. IBM’s approach placed heavy emphasis on error reduction and practical usefulness; the company even launched IBM Q System One, a fully integrated 20-qubit quantum computer in early 2019 as the first commercial quantum system. Still, IBM did not claim to reach quantum supremacy and was cautious with the term. In fact, when Google’s result leaked, IBM researchers quickly responded by optimizing classical simulations to narrow the gap, underscoring that 53-qubit quantum circuits are right on the edge of classical tractability. This friendly rivalry highlights a key point: supremacy is not a permanent condition but a moving target as classical algorithms improve. Nonetheless, Sycamore’s demonstration stands out because IBM’s prior experiments – while highly advanced (e.g. a quantum chemistry simulation on 7 qubits in 2017, or a 50-qubit prototype) – had not crossed that infeasibility threshold for classical computation.
D-Wave’s Quantum Annealers
D-Wave Systems, founded in 1999, took a very different route with quantum computing. Rather than gate-based circuits, D-Wave built quantum annealers – specialized devices designed to solve optimization problems by finding low-energy states in a programmable magnetic system. Years before Google’s news, D-Wave made headlines by selling the world’s first commercial quantum computers and steadily increasing their qubit count (1,000 qubits in the D-Wave 2X machine by 2015, and 2,048 qubits in the D-Wave 2000Q by 2017). However, the crucial distinction is that D-Wave’s qubits are not general-purpose logic qubits; they are tuned for a narrow type of problem (essentially, finding the minimum of a given cost function) using quantum annealing. In 2015, Google and NASA themselves experimented with a D-Wave 2X, reporting it was up to 100 million times faster than a classical simulated annealing algorithm on a specific task. This sounded like an astonishing speedup, but experts noted that the comparison was against one specific classical algorithm on a carefully chosen problem – not a general proof of quantum superiority. Indeed, later studies found that with better classical optimization techniques, much of the D-Wave speedup could be negated. D-Wave machines do use quantum effects (entanglement and tunneling) and have shown they can sometimes outrun classical solvers, but the scientific consensus before 2019 was that they had not demonstrated a broad “quantum supremacy” moment. Unlike Sycamore (which performed a generic computation that’s hard for any classical computer), D-Wave’s advantage is problem-specific – and for many problems, algorithms on classical supercomputers could still keep up or win. In short, D-Wave achieved a different kind of milestone: it proved that hundreds or thousands of qubits could be built and operated together, and it found niche problems with impressive quantum speedups. But Google’s Sycamore was the first to show a programmable, universal quantum processor doing something that classical computing (so far) cannot, which is a stronger claim.
Trapped-Ion Quantum Computers
Another major approach uses trapped ions (electrically charged atoms) as qubits. Trapped-ion systems, pursued by groups at University of Maryland/IonQ, Innsbruck, NIST and others, have achieved some of the highest gate fidelities in quantum computing. By 2019, ion-trap devices could reliably perform single- and two-qubit gates with over 99% accuracy. A hallmark achievement came in 2011, when a team in Innsbruck entangled 14 ions – creating a 14-qubit Greenberger–Horne–Zeilinger (GHZ) state, which was a world record for entanglement at the time. Trapped ions also excel at maintaining coherence: qubits can remain quantum for seconds or minutes, far longer than superconducting qubits (which typically last microseconds). This enabled demonstrations of small quantum algorithms and error correction codes on ion platforms. For example, in 2016, researchers used a 5-ion system to run a simple algorithm and in 2019 a 11-ion device from IonQ was benchmarked on algorithmic tasks. The trade-off is speed and scalability: gate operations on ions are often 1,000 to 1,000,000 times slower than in superconducting circuits (because they rely on laser interactions), and controlling dozens of individual ions with lasers and RF traps is extremely challenging. Before 2019, no trapped-ion experiment had enough qubits to attempt a quantum supremacy challenge; most were focused on <20 qubits but with very high fidelity. Thus, while trapped-ion computers didn’t reach the “supremacy” regime, they set other milestones – like record accuracies and successful execution of quantum logic on fully connected qubit networks. These achievements complement the superconducting approach: ion systems proved that quantum computing is not limited by error rates, and superconducting systems like Sycamore proved that quantum computing is not limited by qubit count. Ultimately, both will need to converge (many qubits and low error rates) to tackle useful problems.
Other Notable Milestones
Several other quantum technologies and experiments paved the way for Sycamore’s breakthrough. Photonic quantum computing, for instance, offered a proposal known as boson sampling as a route to quantum advantage. In theory, a photonic network with ~50 photons could perform a sampling task faster than classical computers. Early boson-sampling experiments in 2013–2018 used small numbers of photons (3 to 6) to validate the concept, but they hadn’t yet scaled to a regime beyond classical simulation. Similarly, in academia, small-scale quantum algorithms were demonstrated on various platforms: e.g. in 2001, IBM’s 7-qubit NMR quantum computer famously factored the number 15 (3×5) using Shor’s algorithm, a historic proof-of-concept that quantum algorithms work. Superconducting qubits, ions, and even quantum optics all saw steady improvements through the 2000s and 2010s, each hitting new benchmarks (higher qubit counts, longer coherence, entangling more particles, etc.). By the late 2010s, quantum hardware had entered what Preskill termed the NISQ era – Noisy Intermediate-Scale Quantum – meaning devices of 50–100 qubits that are not error-corrected, but might perform tasks surpassing classical capabilities. Google’s Sycamore was essentially the first NISQ-era platform to conclusively check that box of exceeding classical computation. It built on many prior innovations (from the first logic gates and entanglement experiments in the 1990s, to the integrated architectures of companies like IBM and D-Wave) and pushed into uncharted computational territory.
Why Does It Matter?
The immediate achievement of Sycamore’s experiment is somewhat esoteric – random circuit sampling is not a useful application by itself. However, the significance lies in the demonstration of capability. It’s a proof of concept that quantum computers, even with present-day imperfections, can outperform classical supercomputers on at least one task. As John Preskill noted, quantum supremacy is more of a scientific milestone than a practical one – “a sign of progress” that boosts confidence in the field. In the eyes of researchers, crossing this threshold is akin to the Wright brothers achieving the first powered flight: the flight itself didn’t go far, but it proved that the new paradigm (heavier-than-air flight, or here quantum computation) works. This validation matters because it justifies the decades-long effort to build quantum hardware and suggests that with further refinement, quantum computers will tackle ever more meaningful problems.
One area of impact is quantum algorithm development. Knowing that a real quantum processor can surpass classical limits encourages computer scientists to design algorithms for nearer-term quantum machines. Until now, many quantum algorithms (like Shor’s factoring algorithm or Grover’s search) assumed large, error-corrected quantum computers that might be years away. The supremacy experiment used a different type of algorithm (random circuit sampling) tailored to NISQ devices. This may inspire new algorithms that, while perhaps not solving “useful” problems yet, can do something uniquely quantum in the NISQ regime. It also drives research into improved classical algorithms; as we saw, IBM quickly found ways to simulate larger quantum circuits more efficiently. This back-and-forth will continue to raise the bar, sharpening our understanding of the boundary between classical and quantum computing.
From an engineering perspective, Sycamore’s success provides a blueprint for building larger quantum systems. The techniques developed – like simultaneous gate calibration across a 2D qubit array and cross-entropy verification – can be applied to future processors with even more qubits. It also exposed the next big challenge: error correction. Sycamore’s 0.2% fidelity meant that with 53 qubits and 20 cycles, errors dominated the output. To do any useful computation (say, running Shor’s algorithm on a large number or simulating a chemistry problem), quantum computers will need far lower error rates or an error-correcting code to sustain much deeper circuits. Thus, the race is on to improve coherence and gate fidelity further, or to incorporate quantum error correction into chips – all while scaling up the qubit count. Google’s team and others are already working on next-generation chips (Google’s beyond-2019 roadmap discussed scaling to 100+ qubits with error correction). The 2019 supremacy experiment, therefore, serves as a benchmark: it’s the maximum problem size a quantum computer could do that a classical one couldn’t, at that moment. Future devices will keep extending this benchmark to more complex tasks, ideally ones with practical value.
Implications for Industry and Computing
Google’s announcement reverberated far beyond academic circles – it signaled to the tech industry that quantum computing is rapidly transitioning from science fiction to reality. In the short term, this achievement fuels the competition (and collaboration) among tech giants and startups working on quantum hardware. Companies like IBM, Intel, Rigetti, and IonQ, as well as research institutions worldwide, all redoubled their efforts, knowing that a new benchmark had been set. While Google used a superconducting platform, others are pursuing alternate technologies (superconductors, trapped ions, photons, topological qubits, etc.), and now each can point to the supremacy milestone as proof that investing in quantum R&D can pay off in demonstrable breakthroughs.
For the broader computing industry, quantum supremacy underscores that classical computing is nearing some fundamental limits for certain problems. Classical supercomputers will of course continue to improve, but not at the exponential pace needed to catch up with exponential quantum state spaces. This is prompting a re-evaluation of how future high-performance computing (HPC) centers might integrate quantum processors as co-processors or accelerators. For example, researchers are exploring hybrid quantum-classical algorithms, where a quantum processor handles the parts of a computation that are intractable for a CPU/GPU, and the classical system tackles the rest. Google’s experiment was essentially an extreme case of this hybrid model (the verification of Sycamore’s outputs was done with help from classical supercomputers like NASA’s Pleiades and ORNL’s Summit on smaller scales). In the coming years after 2019, we expect to see quantum chips being used in tandem with classical HPC for tasks in optimization, simulation, and machine learning. Industry roadmaps by IBM and others already talk about “quantum advantage” for real-world problems, which is the next goal beyond the academic supremacy demonstration.
Another implication is economic and talent-related: a clear quantum computing milestone can trigger greater investment from governments and enterprises. In the late 2010s, countries like the USA, China, and those in the EU launched national quantum initiatives worth billions of dollars. Google’s supremacy result, being high-profile, has likely strengthened the case for these investments. Tech companies may also accelerate their quantum cloud services – IBM had offered public access to small quantum processors since 2016, and in 2019 companies like Amazon and Microsoft were beginning to announce quantum cloud platforms as well. The idea is that businesses and developers should start familiarizing themselves with quantum algorithms now, given that hardware progress is no longer purely theoretical. We can imagine, for instance, pharmaceutical or finance firms experimenting with Google’s or IBM’s quantum processors through cloud APIs, trying out small instances of algorithms (like molecule simulations or portfolio optimizations) to be ready for the day quantum hardware can handle them at scale.
One should temper the enthusiasm with practical reality: outside of a lab setting, useful quantum computing is still in its infancy. The Sycamore processor required a specialized setup (dilution refrigerators, custom control electronics, etc.) and the task it solved doesn’t yet translate to a commercial application. So in the immediate term, the impact on everyday computing tasks is nil – your laptop isn’t obsolete, and in fact will likely help design quantum algorithms through simulation. But in the long term, many industries are eyeing quantum computing breakthroughs that could disrupt their fields. Chemistry and materials science companies foresee quantum computers designing new molecules and catalysts by simulating quantum systems that classical computers can’t handle. Logistics and tech companies are interested in quantum solvers for complex optimization problems (for routing, scheduling, machine learning). Google’s 2019 result gives these stakeholders added confidence that quantum machines will continue to scale and that it’s worth preparing for the quantum future. In summary, the supremacy milestone doesn’t solve commercial problems yet, but it de-risks the field: it proves there’s light at the end of the tunnel, which for industry means it’s reasonable to invest resources and plan ahead for the quantum era.
Cybersecurity Concerns in the Quantum Era
Perhaps the most widely discussed implication of quantum computing – and one of the greatest concerns for society – is its impact on cybersecurity. Modern cybersecurity, from banking transactions to private communications, relies heavily on encryption protocols that are theoretically secure against classical computing attacks but not necessarily against quantum attacks. Google’s Sycamore experiment does not break any encryption – it was computing random circuit outputs, not cracking codes – but it is a stark reminder that more powerful quantum computers are on the horizon. The demonstration intensifies the urgency around quantum-proof cryptography and security strategies.
The primary threat comes from Shor’s algorithm, a quantum algorithm discovered in 1994, which can efficiently factor large integers and compute discrete logarithms – the mathematical problems underpinning RSA and elliptic-curve cryptography (ECC) that secure most internet communications. In simple terms, a full-scale quantum computer running Shor’s algorithm could break RSA/ECC encryption in polynomial time, whereas the best known classical algorithms would take astronomical time. Fortunately, Sycamore’s 53 qubits are nowhere near enough to run Shor’s algorithm on meaningful keys (breaking RSA-2048, for example, is estimated to require thousands of logical qubits and millions of operations, well beyond current capabilities). However, the trajectory is clear: if progress continues, quantum computers in the future will pose a direct threat to today’s public-key cryptography. The 2019 supremacy result, while not cryptographic, shows that the hardware is steadily advancing. It has put governments and companies on notice that they cannot assume current cryptosystems will remain secure indefinitely.
In fact, cybersecurity experts often talk about a “harvest now, decrypt later” threat. An adversary might record encrypted sensitive data today (for example, intercepting encrypted traffic or stealing encrypted databases) and simply store it, with the expectation that in 5, 10, or 20 years, quantum computers will be powerful enough to decrypt that data. If the data has long-term value (think diplomatic communications, trade secrets, medical records), this is a serious concern. Thus, even before a quantum computer exists that can break encryption, we need to begin deploying post-quantum cryptography (PQC) – new cryptographic algorithms that are believed to be quantum-resistant. This transition is a massive undertaking: standards bodies like NIST started a PQC standardization project in 2016, and as of 2019 researchers were evaluating dozens of candidate algorithms (based on mathematical problems like lattices or error-correcting codes that even quantum algorithms struggle with). The challenge is not only technical but also logistical – updating encryption protocols across the Internet, government and military systems, banking infrastructure, etc., could take many years. A 2018 U.S. National Academies report and other studies noted that it might easily take a decade or more to fully replace vulnerable cryptography with quantum-safe solutions, partly because of the “persistence of encrypted information” and legacy systems. In other words, we must act before quantum computers become a threat, not after. Google’s breakthrough serves as a timely proof to policymakers that quantum computing is advancing and is not just a theoretical curiosity.
It’s worth emphasizing that symmetric cryptography (like AES encryption) and hashing are also affected by quantum computing, though in a less dramatic way. Grover’s algorithm, another quantum algorithm, can theoretically brute-force search (e.g. test encryption keys) in roughly the square root of the classical time. This means a quantum computer could reduce the security of symmetric ciphers by effectively halving the key length (for instance, turning a 128-bit key into an equivalent of a 64-bit key in strength). Cybersecurity professionals have anticipated this as well: to be safe from Grover’s algorithm, one can double key sizes (AES-256 is considered safe against quantum attacks corresponding to AES-128’s classical security, for example). So, the more urgent upheaval is in public-key crypto (RSA/ECC), which needs entirely new algorithms, whereas symmetric crypto and hashing just need larger parameters.
Another angle to quantum and cybersecurity is quantum communication itself. Quantum computing’s rise is paralleled by developments in quantum cryptography, particularly Quantum Key Distribution (QKD). QKD allows two parties to share encryption keys with security guaranteed by the laws of physics (via entangled photons or other quantum states). Any eavesdropping on a quantum channel can be detected, making the communication theoretically interception-proof. QKD is already commercially available in some form (fiber-optic and satellite-based QKD demonstrations have been done), and it provides a way to mitigate future quantum decryption threats – if you distribute your keys via QKD instead of classical algorithms, a quantum computer can’t steal those keys because it can’t eavesdrop without detection. The catch is QKD requires specialized hardware and is distance-limited, so it’s not a drop-in replacement for current public-key infrastructure. Nonetheless, in response to quantum computing advances, more governments and companies are investing in quantum communication networks as a hedge for cybersecurity. For instance, in 2017 China launched a quantum satellite enabling QKD between continents, and the EU and US have quantum-secure network pilot programs. These efforts, while somewhat separate from computing, are part of the quantum arms race in cybersecurity – basically, using quantum technology (like QKD) to defend against quantum technology (like Shor’s algorithm).
From a policy and strategy perspective, Google’s quantum supremacy milestone has likely prompted organizations like the NSA, NIST, and equivalents worldwide to accelerate their guidance on cryptographic transition. As early as 2015, the U.S. National Security Agency warned that adversaries could eventually have quantum capabilities and advised plans to move to quantum-resistant cryptography. By 2019, the writing on the wall is clear: it’s time to start phasing in post-quantum encryption for sensitive data. The good news is that the cybersecurity community is responding – the PQC competition is well underway, and draft standards for quantum-safe algorithms are expected in the early 2020s. In parallel, companies are beginning to implement hybrid solutions (combining classical RSA with a quantum-safe algorithm, for instance) to test these new schemes in practice.
In summary, the cybersecurity implications of quantum computing add a note of urgency to an otherwise celebratory scientific achievement. Google’s Sycamore processor itself doesn’t threaten our passwords or bank accounts today, but it vividly illustrates that the quantum revolution is not hypothetical. The time frame for action – upgrading the world’s cryptography – is measured in years to decades, and that is roughly the same horizon on which many experts believe quantum computers capable of breaking cryptography could become operational. The prudent approach for industry and government is to act now: invest in post-quantum cryptography research, begin testing quantum-safe protocols, and develop migration plans for critical systems. The 2019 milestone serves as a public milestone saying “quantum computing is here – small, but here”. Cybersecurity must adapt accordingly, to be ready before quantum computers graduate from sampling random numbers to cracking real ones.