Variational Quantum Eigensolver (VQE) Breakthroughs
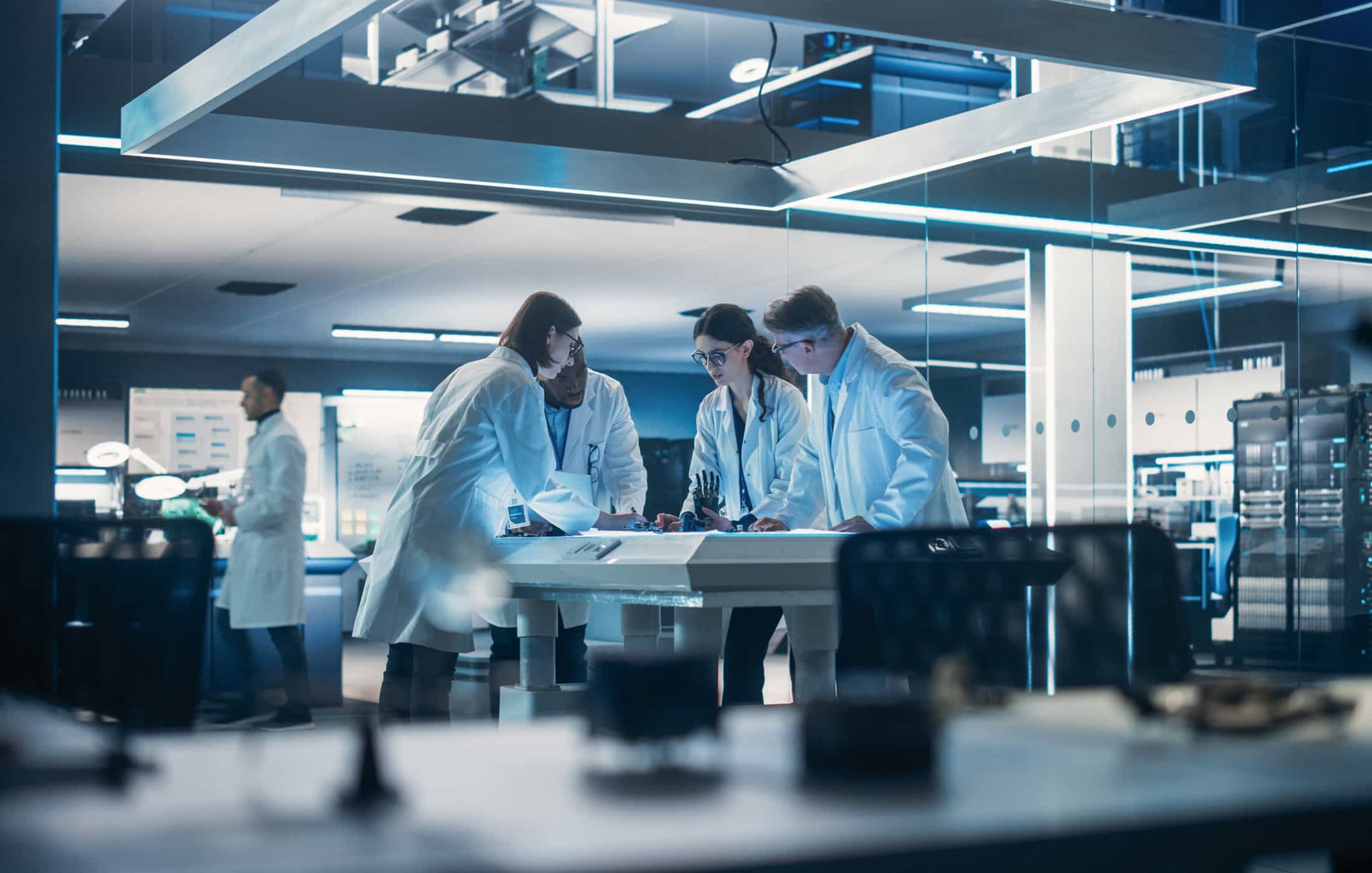
Table of Contents
The Variational Quantum Eigensolver (VQE), introduced in 2014, has rapidly become a flagship algorithm for simulating ground-state properties on today’s noisy quantum computers. Rather than running long quantum circuits, VQE uses short circuits and a classical optimizer in tandem: the quantum processor prepares a trial wavefunction with adjustable parameters, and a classical computer iteratively tweaks those parameters to minimize the measured energy. This hybrid approach was first developed to find molecular ground-state energies , and it has since been adapted to a broad range of problems in chemistry and physics. In the past few years, researchers in academia and industry (including IBM, Google, and Microsoft) have achieved a series of notable VQE milestones – from accurately computing the energies of real molecules to probing strongly correlated materials and even simple nuclear systems.
Quantum Chemistry: Simulating Molecules and Reactions
Early successes of VQE centered on small molecular systems. In 2017, IBM researchers demonstrated a hardware-efficient VQE on a superconducting processor that optimized up to six qubits to find the ground-state energies of molecules like H₂, LiH, and even beryllium hydride (BeH₂). This was a leap beyond prior experiments (which mostly involved only hydrogen or helium) – the IBM team showed that a carefully designed ansatz could handle Hamiltonians with over a hundred terms and still recover accurate energies up to BeH₂. They also used the same approach to solve a quantum magnetism problem, underscoring VQE’s flexibility across chemistry and condensed matter. Around the same time, trapped-ion systems achieved similar feats: a 2018 experiment used four qubits in a trapped-ion quantum simulator to compute the ground-state energies of H₂ and LiH, implementing VQE with different encoding methods. Despite the limited qubit count, this IonQ/NIST experiment was the first scalable ion-trap VQE demonstration, and it highlighted error mitigation techniques needed to reach “chemical accuracy” on hardware.
Since then, VQE has steadily progressed to more complex molecules and larger quantum processors. A headline result came in 2020, when Google’s quantum team performed VQE simulations for a hydrogen chain with 12 atoms – one of the largest chemistry simulations run on a quantum device to date. Using 12 superconducting qubits, they modeled the binding energy curves of H₆, H₈, H₁₀, and H₁₂ chains, as well as the cis–trans isomerization of diazene (N₂H₂). In this study, VQE prepared the Hartree–Fock wavefunctions for these systems, and the team introduced error-mitigation strategies (enforcing physical symmetries of the electronic wavefunction) to dramatically improve fidelity. The 12-qubit results showed good agreement with exact Hartree–Fock calculations, demonstrating that even today’s NISQ hardware can capture non-trivial chemistry (highly entangled, but classically tractable states) as a validation step. This achievement laid groundwork for tackling correlated electron effects on larger quantum devices in the future.
Very recent VQE experiments have started to probe chemical reaction pathways and more challenging compounds. In late 2024, an IBM-led team reported the first quantum computation of a reaction’s activation energy – using VQE-based methods to map out a Diels–Alder reaction profile. They used up to 8 qubits on an IBM quantum processor to calculate the energy of the transition state in a cyclopentadiene + ethylene reaction, accounting for both static and dynamic electron correlation. By combining entanglement forging (to reduce qubit requirements) with quantum subspace expansion and perturbative corrections, the hybrid quantum-classical workflow could predict the activation barrier in close agreement with high-level classical chemistry models (CASCI). This is an exciting step because reaction kinetics and transition states are key targets for quantum chemistry. More broadly, improved VQE algorithms and ansatz design have expanded the menu of molecules accessible on quantum hardware. Researchers have begun exploring larger organic molecules and complex inorganic chemistry: for example, recent studies have used VQE or its variants to simulate benzene under various distortions, the isomerization of butadiene, and even a bimetallic chromium complex, by cleverly reducing the problem size or partitioning the computations. In addition, specialized VQE adaptations have been developed to handle vibrational spectra and excited states – enabling calculations of molecular vibrational levels (e.g. in CO₂, formaldehyde, and formic acid) within the VQE framework. While these advanced cases often involve significant classical preprocessing or offloading some tasks to classical solvers, they showcase the growing reach of VQE in quantum chemistry research.
Condensed Matter: Ground States of Strongly Correlated Models
Simulating strongly correlated materials and lattice models is another arena where VQE has made tangible strides. The algorithm has been applied to find the ground states of spin models and fermionic lattice Hamiltonians that are intractable by simple classical means. One early demonstration (by the same IBM group in 2017) showed that a hardware-efficient VQE ansatz could find the ground energy of a small quantum magnet – essentially a few-spin model – using the available two-qubit gates in a superconducting device. This hinted that VQE might tackle condensed matter problems beyond molecules. The real breakthrough, however, came with improved hardware and algorithms by 2022. In a landmark experiment, a collaboration led by academic startup Phasecraft used VQE to capture key physics of the Fermi–Hubbard model, a quintessential strongly correlated electron system. They implemented a low-depth VQE circuit on 16 superconducting qubits to prepare the ground state of Hubbard model instances as large as a 1×8 chain and a 2×4 two-dimensional patch. Notably, these sizes go beyond what one can easily solve with brute-force classical algorithms (the 1×8 and 2×4 Hubbard lattices have 16 sites, which is challenging for exact diagonalization). Even with the limitations of a noisy device, the variational approach was able to reproduce qualitative features of the Hubbard ground state, including signatures of the metal–insulator transition and antiferromagnetic order emerging in the 1D and 2D systems. In the 1×8 Hubbard chain, for instance, the team observed Friedel oscillations and the expected decay of correlations indicative of a Mott insulator forming at half-filling. Achieving these physics benchmarks on real hardware required an array of techniques to tame the noise – the researchers enforced symmetries of the Hubbard Hamiltonian and deployed error mitigation methods tailored to fermionic simulations, as well as a new Bayesian optimizer to navigate the energy landscape . The success of this experiment was a strong proof-of-principle that VQE, with clever ansatz and mitigation, can handle medium-scale quantum materials problems that push the edge of classical computational tractability.
Beyond the Hubbard model, VQE has also been used to study simpler lattice models like the Schwinger model (a 1D lattice gauge theory akin to quantum electrodynamics) and various spin chains. Even before the 16-qubit Hubbard study, smaller VQE experiments had shown it can find ground states of spin-$$\tfrac{1}{2}$$ models like the transverse-field Ising chain or Heisenberg model on a few qubits, often as test cases for ansatz development. In one case, trapped-ion qubits were used to prepare the ground state of a fully-connected Ising model via VQE, leveraging the native all-to-all connectivity of ions (though results were largely classical verification due to noise). What’s particularly encouraging is how VQE scales when combined with problem-specific knowledge. In 2024, a team from the University of Washington introduced “scalable circuits” ADAPT-VQE to prepare the ground state of the Schwinger model and successfully ran it on up to 100 qubits of IBM’s quantum hardware. They exploited the physical structure of the model – namely the finite correlation length in a gapped lattice gauge theory – to construct an ansatz that can be grown systematically for larger lattice sizes. Using this approach, circuits optimized on small instances (20–28 qubits) were extrapolated to much larger systems. The team prepared the vacuum state of the Schwinger model on a 100-qubit portion of IBM’s 127-qubit Eagle processor and measured observables like the chiral condensate and charge correlations . Thanks to a novel error-mitigation technique (dubbed “operator decoherence renormalization”) the results closely matched those from classical tensor network simulations . This remarkable feat – preparing a physically meaningful 100-qubit entangled state – stands as one of the clearest demonstrations that VQE-based algorithms can leverage increased qubit counts with noise under control. While the Schwinger model is a special case, the scalable ADAPT-VQE approach hints at how we might tackle other strongly correlated systems by breaking them into manageable pieces.
Nuclear and High-Energy Physics: Towards Quantum Simulation of Hadrons
VQE’s promise extends even to nuclear physics and simple high-energy physics models, where classical computational methods struggle with exponential complexity or sign problems. A pioneering example was the 2018 simulation of a deuteron (hydrogen-2) nucleus binding energy using VQE. In that study, physicists from Oak Ridge National Lab mapped a nuclear Hamiltonian (derived from an effective field theory of nucleons) onto two qubits and ran VQE on IBM’s cloud quantum processors. They employed a hardware-efficient version of the unitary coupled cluster ansatz and successfully calculated the deuteron’s ground-state energy to within a few percent of the true value. This result, published in Phys. Rev. Lett., was the first ab initio calculation of a nuclear binding energy on a quantum computer. It demonstrated that VQE could handle non-chemistry Hamiltonians and paved the way for more complex nuclear simulations.
Since then, quantum computing groups have explored VQE for other nuclear phenomena on a small scale. For example, researchers have shown that combining VQE with the quantum subspace expansion (QSE) allows access to excited-state properties like scattering. In late 2023, a team reported using VQE+QSE to calculate nuclear scattering phase shifts in a two-nucleon system. They targeted the spin-triplet S-wave scattering channel of the deuteron (³S₁ state) using a simple pionless EFT Hamiltonian, and ran their algorithms on up to 5 qubits of IBM’s superconducting hardware. The variational algorithm prepared approximate excited states (quasi-continuum states) which the QSE then refined to extract phase shift data. The computed phase shifts from the quantum hardware showed reasonable agreement with theory at low energies, although reaching higher accuracy will require further noise reduction. This work illustrates that VQE-based methods can explore aspects of nuclear reactions and spectra, not just ground states, albeit in minimal models.
In the realm of high-energy physics, we already saw the Schwinger model vacuum prepared on 100 qubits as a proxy for 1D quantum electrodynamics. There have also been proof-of-concept VQE studies on small lattice gauge theory problems. For instance, researchers have proposed bosonic VQE variants to handle gauge fields with infinite-dimensional Hilbert spaces (using microwave cavity qubits instead of standard qubits). And a recent simulation examined the phase structure of the Schwinger model with a topological $$\theta$$-term by preparing ground states via VQE on an IBM device (using 14 qubits to represent a tiny lattice). These are early steps, but they suggest VQE could eventually tackle phenomena like vacuum structure and phase transitions in particle physics models. The key takeaway is that even in domains like nuclear and high-energy physics – far from VQE’s original chemistry focus – the algorithm is being tested and refined on current quantum hardware, inching toward regimes that challenge classical simulation.
Scaling Up: Algorithms, Hardware, and Error Mitigation
Achieving the milestones above required not just bigger quantum chips, but also significant algorithmic innovations to make VQE more scalable and noise-tolerant. One line of progress has been in designing better ansätze (trial wavefunctions) that use hardware resources efficiently. The “hardware-efficient” ansatz introduced by IBM in 2017 (a layered sequence of native gates) was one approach, but it can suffer from optimization difficulties as systems grow. To address this, researchers developed ADAPT-VQE – an adaptive algorithm that builds the ansatz iteratively by adding only the most important operators one by one. This adaptively constructed ansatz often yields the exact ground state with far fewer parameters than a fixed ansatz, as demonstrated in 2019 for small molecular examples. More recently, in 2023, an improvement called Overlap-ADAPT-VQE was proposed to avoid getting trapped in local minima during the ansatz growth. Instead of greedily minimizing energy (which can lead to redundant or over-parameterized circuits), Overlap-ADAPT grows the wavefunction by maximizing overlap with a precomputed target state that already captures some correlation. This strategy guides the VQE ansatz through a smoother landscape, producing ultra-compact circuits that still recover high accuracy. Tests on strongly correlated electron systems showed that Overlap-ADAPT-VQE can achieve chemical accuracy with shallower circuits than standard ADAPT, making it a promising candidate for larger molecules or materials. Such algorithmic refinements are crucial for extending VQE to problems beyond the reach of brute-force classical methods.
Another area of progress is in error mitigation and noise-resilient VQE. By design, VQE has some inherent robustness to noise (since the variational optimization may find a state that’s lower in energy given the noise); however, attaining quantitative accuracy typically needs additional techniques. Over the last couple of years, researchers have deployed a toolkit of error-mitigation methods in virtually every significant VQE experiment. These include zero-noise extrapolation, where one intentionally increases circuit noise and extrapolates the results back to zero noise, and symmetry verification, where measurements that produce unphysical symmetry-breaking results are discarded or corrected. For instance, the 16-qubit Hubbard simulation used symmetries of the particle number and spin sectors to post-select valid quantum states and a special fermionic “post-processing” correction to counteract certain errors. Similarly, Google’s H₁₂ chain simulation enforced $$N$$-representability conditions (i.e. physical constraints on the one- and two-electron density matrices) to filter out erroneous results and thereby restored the fidelity of the Hartree-Fock state preparation. On the IBM side, the 100-qubit Schwinger study introduced the aforementioned operator decoherence renormalization, which adjusted measured observables to account for correlated error effects, yielding physically meaningful expectation values. Thanks to these methods, VQE outcomes on noisy hardware have steadily crept closer to ideal results, even as qubit counts and circuit depths increase. It’s important to note that full error correction is still out of reach in these experiments – instead, these are error mitigation strategies that reduce the impact of noise without requiring extra qubits for encoding. The growing library of such techniques (including recent ideas like probabilistic error cancellation and machine-learning-based error inference) is helping VQE maintain accuracy until hardware improves further.
Finally, the range of hardware platforms executing VQE has diversified. Superconducting qubit platforms (like IBM Quantum and Google’s Sycamore) have been the workhorses for many achievements listed, offering high gate speeds and steadily increasing qubit numbers (dozens to over a hundred qubits) with improving quality. Trapped-ion quantum computers (IonQ and academic lab setups) play a key role for smaller problems where their all-to-all connectivity and long coherence can offset slower gates – as seen in the LiH simulation and various quantum chemistry VQE benchmarks on 4–11 ion systems. Even photonic qubits had an early contribution: the very first proof-of-concept VQE experiment was run on a small photonic processor, estimating the H₂ molecule’s energy using just two qubits (implemented as dual-photon entangled states). Each platform brings unique advantages, and researchers are beginning to tailor VQE ansätze to each hardware’s strengths – for example, using native ion-trap gate sets for efficient state preparation, or leveraging superconducting qubits’ faster circuit cycles to run many VQE iterations with different parameters. There is also active work on analog VQE-like approaches (using continuous variables or analog quantum simulators with a variational principle), but the most notable milestones so far have come from gate-based digital quantum computers as described above.
Outlook
Taken together, these achievements paint an encouraging picture of VQE’s evolution. In the span of about five years, VQE has graduated from toy models to meaningful simulations of chemical reactions, complex molecular systems, and strongly correlated materials – all on quantum hardware that is still imperfect. Each “first” (first simulation of a new molecule, first observation of a physics phenomenon, etc.) underscores both the progress and the remaining challenges. Importantly, no fundamental quantum advantage has been claimed yet in these VQE demonstrations; classical computers can still handle or double-check most of the results (sometimes with significant effort). But the scale and fidelity of VQE simulations are improving continuously. The variational approach has proven itself as the workhorse of near-term quantum simulation, and each new milestone – larger molecules, deeper physics, better accuracy – is a step toward the ultimate goal of quantum-enabled modeling in chemistry and materials science.