Quantum Computing Use Cases in Materials & Chemicals
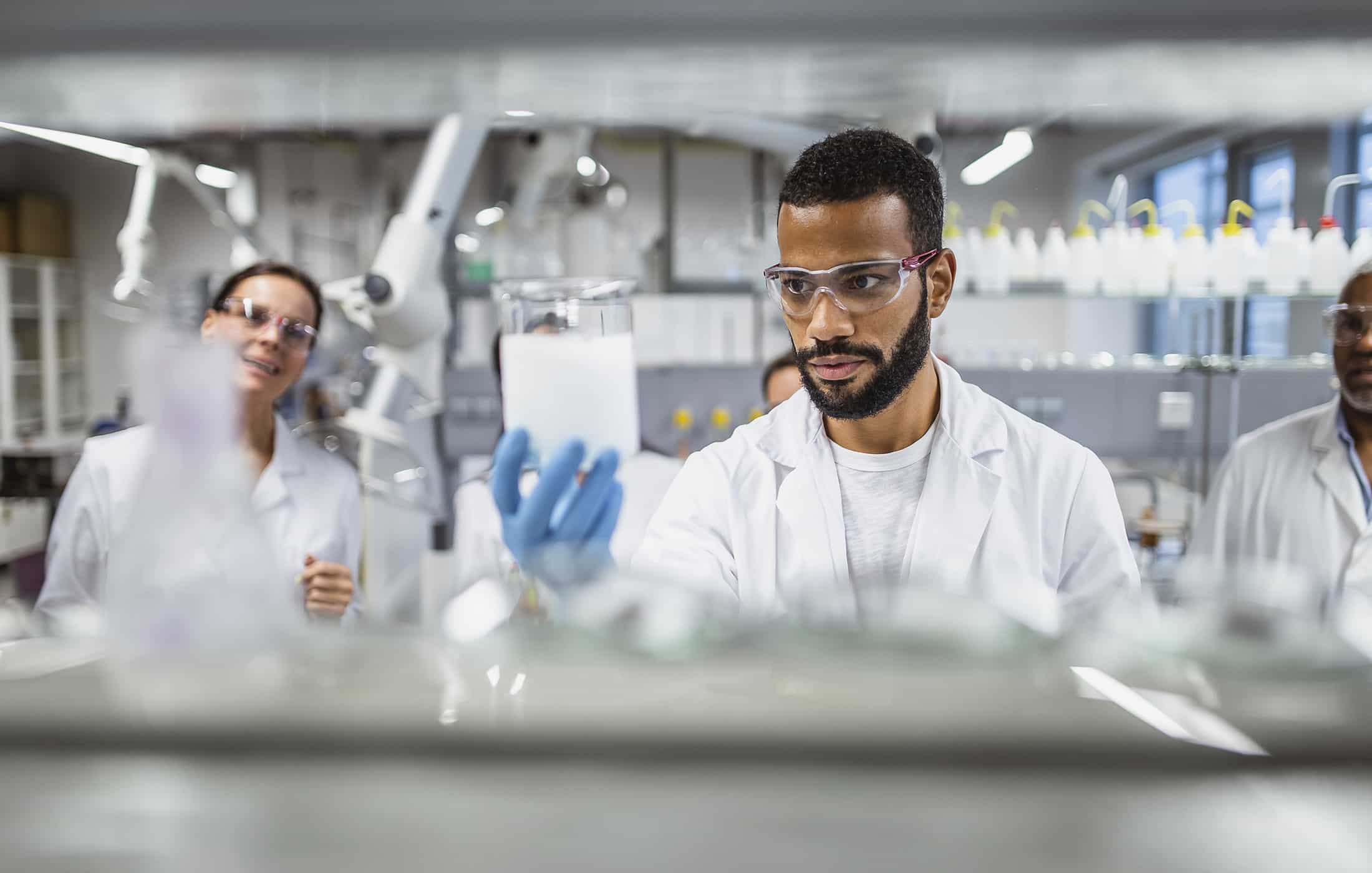
Table of Contents
Introduction
Quantum computing harnesses the counterintuitive properties of quantum physics – superposition, entanglement, and quantum interference – to process information in fundamentally new ways. Unlike classical computers limited by binary bits, quantum computers use qubits that can exist in multiple states simultaneously, enabling them to explore vast computational possibilities in parallel. This paradigm is especially promising for materials science and chemical engineering, where many challenges boil down to understanding complex quantum-mechanical interactions of atoms and molecules. In fact, using quantum computers for computational chemistry and materials science may allow researchers to tackle problems “intractable on classical computers,” such as accurately simulating molecular behaviors or novel material properties. Industry experts predict rapid growth in quantum computing over the next decade, driven largely by its potential in pharmaceutical, chemical, and materials applications. In short, quantum computing offers a revolutionary toolset for these sectors – one that could accelerate innovation by solving equations and simulations that were previously unsolvable, ultimately leading to breakthroughs in new materials, chemical processes, and technologies.
Current Developments
Major strides in recent years indicate that quantum technology is steadily moving from theory to practice in materials science. Governments are investing heavily: for example, the U.S. Department of Energy launched a $30 million program to leverage quantum computing for chemistry and materials simulations. This initiative (QC3) specifically targets breakthroughs like sustainable industrial catalysts, novel high-temperature superconductors for efficient power grids, and improved battery chemistries. Each project in the program is challenged to achieve quantum solutions that outperform classical methods by 100× or more, aiming for transformative impacts such as significant greenhouse gas reductions or big energy efficiency gains. At the same time, private-sector and academic collaborations are blooming. Large companies are partnering with quantum computing startups and research labs to explore domain-specific applications. For instance, Volkswagen teamed up with Canadian startup Xanadu on quantum algorithms for battery materials, seeking safer, lighter, and cheaper next-gen batteries. In a multi-year program, VW and Xanadu are co-developing quantum simulation methods to complement or surpass traditional techniques like density-functional theory, which are beginning to hit limits in battery research. Similarly, Boeing and IBM have joined forces to study metal corrosion using quantum simulations – a critical issue that costs industry billions. In 2023, their researchers demonstrated quantum methods to simulate a key corrosion reaction (water reduction) more accurately than classical chemistry approaches.
Industry investment is likewise accelerating. Tech leaders such as Google and IBM have achieved early milestones by using small quantum processors to simulate simple chemical systems. “Google built a quantum computer that could simulate a simple chemical reaction,” one report noted, hinting at quantum hardware’s growing ability to model molecular dynamics. Chemical companies are also getting involved: for example, Japan’s JSR and Mitsubishi Chemical joined the IBM Q Network (a quantum computing consortium) to access 20- and 50-qubit machines for tackling their unique R&D challenges. These collaborations give companies a head start in applying quantum algorithms to real-world materials and chemical problems.
Furthermore, a wave of startups – backed by venture capital and corporate funding – is focusing on quantum chemistry and materials. They range from software firms optimizing quantum chemical algorithms to specialist companies targeting drug discovery and materials design via quantum computing. The momentum is unmistakable: across government, academia, and industry, significant resources are being poured into quantum-driven materials science initiatives.
Industry-Specific Use Cases
Quantum Materials Discovery
Designing new materials often requires understanding complex electron interactions, crystal structures, and quantum phenomena that push classical computing to its limits. Quantum computers offer a way to directly simulate these quantum systems, enabling “materials by design” approaches that were previously impractical. Researchers are beginning to use quantum algorithms (like the Variational Quantum Eigensolver and Quantum Phase Estimation) to predict material properties such as electronic band structures, magnetic behavior, and superconductivity. A recent milestone came from Infleqtion (a quantum hardware company) working with university partners: they demonstrated the first use of logical qubits to boost the accuracy of materials simulations six-fold, applied to exploring new battery materials and high-temperature superconductors. By encoding error-corrected logical qubits on a neutral-atom quantum processor, the team could more reliably calculate the quantum properties relevant to designing these advanced materials. Similarly, the U.S. ARPA-E’s QC3 program is explicitly targeting “novel superconductors for more efficient electricity transmission” and other material breakthroughs using quantum computing.
One exciting application is discovering better catalysts (materials that speed up chemical reactions). Catalysts are central to processes from fertilizer production to pollution control, but finding optimal catalysts (that are efficient, cheap, and environmentally benign) involves massive combinatorial searches of material compositions. Quantum simulations can evaluate the behavior of candidate catalyst materials at the atomic level with high fidelity. In the UK, a partnership between quantum software firm Riverlane and Johnson Matthey (a large chemicals company) has developed tools for quantum computers to calculate catalyst properties that are too complex for classical methods. Their software encodes the intricate sub-atomic interactions in chemical reactions into instructions for a quantum processor, which can analyze many interaction possibilities simultaneously. The vision is that as quantum hardware scales, scientists will be able to design sustainable catalysts from scratch – for example, catalysts for hydrogen fuel cells that don’t rely on scarce platinum. By accurately computing reaction energetics on an error-corrected quantum computer, researchers could discover new catalyst materials made of abundant elements, dramatically reducing costs and environmental impact. More broadly, quantum-enabled materials discovery could yield breakthroughs in semiconductors (e.g. finding materials with ideal bandgaps for electronics or solar cells) and lightweight alloys or polymers with tailored properties. Early studies have already shown that reformulating materials problems for quantum annealers or gate-based quantum computers can tackle challenges like predicting polymer structures (another combinatorially complex task) faster than classical algorithms.
Molecular & Chemical Simulations
Chemical engineering often boils down to simulating molecular interactions and chemical reactions – tasks that become exponentially difficult as system size grows. Quantum computing is poised to revolutionize molecular simulation by modeling quantum chemistry natively. In classical simulations, chemists rely on approximate methods (like density functional theory or Monte Carlo sampling) to study molecules, because exact solutions of the Schrödinger equation for many-electron systems are usually impossible on conventional hardware. Quantum computers, however, can in principle handle the full quantum complexity of molecular systems. They use qubits to represent the quantum states of electrons directly, potentially solving for properties like reaction energies, transition states, and reaction kinetics with much higher accuracy. Already, small-scale quantum processors have been used to simulate simple molecules. IBM researchers, for example, used a 5-qubit quantum computer to calculate the ground-state energy and dipole moment of lithium hydride (LiH), a basic molecule relevant to battery chemistry. This was one of the first demonstrations of a quantum computer performing a chemistry calculation that correlates to designing better batteries. Likewise, Google’s quantum computing team reported simulating the energy surface of a modest chemical reaction, marking the largest chemical simulation on a quantum device to date as of 2020.
Beyond proof-of-concept molecules, researchers are developing quantum algorithms to tackle reaction dynamics and molecular kinetics – for instance, simulating how a reaction proceeds over time (which classically requires solving coupled differential equations with many variables). Quantum algorithms for time evolution can, in theory, track quantum state changes during a reaction more naturally. Recent research has presented quantum approaches to calculate scattering matrix elements for chemical reactions, aiming to predict outcomes of molecular collisions and transformations more efficiently. Another area is quantum-enhanced molecular dynamics, where a quantum computer could help sample molecular configurations according to quantum Boltzmann distributions (important for understanding temperature-dependent reactions and catalysis). While current quantum hardware is still too limited (noisy and with only tens of qubits) to outperform classical supercomputers for realistic chemistry, progress is steady. Each year brings improved quantum simulation algorithms and error mitigation techniques that extend the size of molecules that can be reliably modeled. The ultimate promise is that future large-scale quantum computers could simulate complex chemical processes – like combustion mechanisms, atmospheric chemistry, or enzymatic reactions – with full accuracy, providing insights that enable engineers to design more efficient reactions and processes. As one perspective notes, finding the “sweet spot” of problems where classical methods struggle but quantum methods excel is an active area of research. Multi-metal chemical systems and highly correlated reactions (where classical approximations break down) are likely early targets for quantum advantage in molecular simulation.
Quantum-Assisted Drug & Polymer Design
In the pharmaceutical and biomaterials arena, quantum computing is emerging as a cutting-edge tool for drug discovery and the design of complex polymers. Drug molecules work by binding to biological targets (like proteins), and predicting the strength of these interactions is crucial for identifying effective new therapies. However, accurately modeling phenomena like protein-ligand binding affinities or protein folding requires accounting for many-body quantum effects (e.g. the behavior of electrons in a large, flexible molecule and the solvent effects around it), which is extremely challenging for classical computation. Quantum computers offer a new approach to this problem. For example, researchers from Pasqal and Qubit Pharmaceuticals recently demonstrated a hybrid quantum-classical workflow to analyze protein hydration – essentially mapping how water molecules occupy and influence the binding pockets of proteins. They used a neutral-atom quantum processor to place water molecules in a simulated protein cavity, leveraging quantum superposition to efficiently evaluate many possible arrangements of water simultaneously. This kind of analysis is important because water molecules often mediate the binding of drug molecules to proteins, affecting the drug’s efficacy. By capturing these effects more precisely, quantum simulations can improve our understanding of the true binding mechanism in ways classical models might miss. The same team applied quantum algorithms to model ligand-protein binding dynamics with high accuracy, feeding the results into machine learning models for drug discovery. This indicates a future where quantum computing accelerates the identification of promising drug candidates by evaluating molecular interactions at unprecedented resolution.
Polymer design is another domain ripe for quantum enhancement. Polymers – whether plastics, biological macromolecules like DNA, or advanced composites – have enormously complex configuration spaces. A single polymer chain can fold and arrange itself in astronomically many ways, and properties like strength, flexibility, or biocompatibility emerge from these configurations. Classical simulations can handle small polymers or rely on coarse-grained models, but they struggle with dense polymer mixtures or knots in long chains. Quantum approaches are showing early success in this field. In one study, scientists reformulated a polymer modeling problem for a D-Wave quantum annealer, enabling them to predict polymer chain conformations by finding the minimum-energy arrangement of a corresponding quantum system. Essentially, they encoded the rules of polymer physics (which conformations are allowed or forbidden) into a quantum Hamiltonian, so that the annealer’s lowest-energy state directly yielded valid polymer structures. The result was a quantum algorithm capable of sampling polymer configurations more efficiently than classical Monte Carlo in certain cases. Although current hardware limited the size of polymers they could test, the approach scaled favorably and points toward future quantum “design engines” for polymers. In practice, this could mean quickly identifying polymer compositions with desired traits (like a polymer electrolyte that conducts ions well for a battery, or a biodegradable plastic with high strength) by letting a quantum computer sift through configuration possibilities far faster than trial-and-error lab synthesis. As quantum hardware and algorithms advance, the pharmaceutical and materials industries anticipate integrating these tools into their R&D pipelines – potentially cutting years off the development of a new drug or material by computationally finding optimal molecular designs.
Battery & Energy Storage Innovation
Energy storage is a critical area where materials science and chemistry intersect – and where quantum computing is expected to make a major impact. Developing better batteries, fuel cells, and other storage technologies largely hinges on discovering new materials for electrodes, electrolytes, and catalysts that enable higher capacity, faster charging, and longer lifetimes. Quantum computing can contribute by deeply simulating electrochemical processes and material properties that govern performance in these systems. A prominent example is lithium-ion batteries. Improving battery chemistry involves understanding phenomena like ion diffusion, electron transfer reactions, and the formation of solid-electrolyte interfaces at the atomic level. Classical simulations can only go so far in modeling these multi-scale, quantum-mechanical processes. Quantum computers, however, can directly simulate the relevant quantum states of battery materials. In 2020, a collaboration between IBM and Daimler (Mercedes-Benz) used a quantum computer to model key lithium-containing molecules involved in lithium-sulfur batteries, an emerging high-density battery technology. They calculated properties like the dipole moment of lithium hydride and other lithium-sulfur compounds on a 4-qubit device, demonstrating the potential to eventually compute reaction mechanisms inside a battery cell with high precision. The goal of such studies is to help battery scientists pinpoint which chemical pathways lead to degradation and which could yield more stable, high-capacity batteries. Volkswagen’s work with Xanadu likewise highlights this direction: by focusing on quantum simulation of battery cathode materials, they aim to accelerate the search for safer and lighter battery materials within the decade. Quantum simulation could, for example, reveal new composite materials or dopants for battery electrodes that allow faster ion flow or prevent unwanted side reactions, thus improving charging speed and battery longevity.
Beyond batteries, fuel cells and hydrogen storage materials also stand to benefit. Fuel cells rely on catalysts (often platinum-based) for reactions like splitting hydrogen gas into protons and electrons. Quantum computing can help design non-platinum catalysts by accurately calculating the activation energies of alternative catalytic materials or complexes. In one vision, a large fault-tolerant quantum computer would let engineers virtually test a huge range of candidate compounds for, say, the hydrogen oxidation reaction, identifying efficient catalysts made from inexpensive elements. Similarly, materials for safe hydrogen storage or novel battery types (such as solid-state batteries or flow batteries) involve complex solid-solid and solid-liquid interactions that quantum simulations could unravel. Even supercapacitors and emerging electrochemical storage methods involve quantum phenomena (like quantum capacitance at nanoscale interfaces) that could be better understood with quantum models. The overarching promise is that quantum computing will significantly shorten the material discovery cycle for energy storage: instead of years of lab experimentation guided by trial-and-error and simplified models, researchers could run highly accurate simulations of candidate materials and electrochemical reactions on quantum hardware, narrowing down the most promising options in days. Major automakers, oil & gas companies, and battery manufacturers are already monitoring these developments closely, with partnerships (like Total with quantum startups) aiming to be early adopters of quantum-assisted battery research. As electric vehicles and renewable energy storage needs grow, the pressure to deliver leaps in battery performance makes this one of the most eagerly anticipated use cases for quantum computing.
Corrosion & Surface Science
Every year, industries worldwide lose billions of dollars to corrosion – the gradual degradation of materials (especially metals) due to chemical reactions with their environment. From pipelines and aircraft to chemical plants, corrosion is a pervasive challenge in materials engineering. Yet, the chemistry of corrosion (often electrochemical reactions at metal surfaces) is complex and not fully understood in many cases. Quantum computing is poised to offer new insights by simulating corrosion processes at the quantum level, helping scientists devise better corrosion-resistant materials and coatings. A notable effort in this arena is the IBM–Boeing collaboration mentioned earlier, which focuses on using quantum algorithms to study the fundamental reactions that cause rust and material fatigue. They specifically looked at the water reduction reaction – a key step in electrochemical corrosion where water and oxygen lead to oxide formation on metals. Using quantum simulations, the researchers computed the reaction’s energy landscape more accurately than conventional methods, which can improve predictions of how and when corrosion will initiate. Importantly, they also developed quantum circuit optimization techniques to run these simulations efficiently on current hardware. The long-term vision is to simulate entire corrosion mechanisms (in, say, an aluminum alloy or a steel under stress) to identify points of vulnerability and test how various inhibitors or protective coatings might interrupt the process.
On the experimental side, quantum sensors (discussed in the next section) are enhancing surface science by detecting early signs of corrosion that were previously undetectable. For example, quantum magnetometer sensors can pick up tiny magnetic field changes caused by micro-cracks or corrosion byproducts in ferromagnetic materials. Combining this with quantum-computed models provides a powerful one-two punch: quantum computing predicts where and how corrosion is likely to occur at the atomic scale, and quantum sensors validate those predictions in real materials by observing initial damage at the nanoscale. Another application of quantum computing in surface science is studying catalyst surfaces and adsorption. Many industrial processes involve reactants adsorbing onto metal surfaces (catalysts) – a quantum process that determines reaction rates and pathways. Quantum simulations can shed light on these surface interactions (for instance, how a molecule dissociates on a platinum surface during catalysis) more accurately than classical simulations, which helps in designing surfaces that resist deactivation (a form of “surface corrosion” in catalysts). In sum, by illuminating the quantum mechanics of material degradation, quantum technologies will help engineers develop smarter corrosion prevention strategies – from advanced alloys that naturally form stable protective films, to optimized coatings whose chemistry (tailored with quantum precision) halts corrosion before it starts.
Quantum Sensors for Material Characterization
While quantum computing grabs a lot of headlines, quantum sensing technologies are also transforming materials science and chemical engineering. Quantum sensors exploit quantum effects (such as spin precession, entanglement, or quantum state interference) to achieve measurement precision far beyond classical sensors. In material characterization, this means we can probe structures and phenomena at extremely small scales, or detect minute signals that were previously drowned out by noise. One striking example is the use of NV-center magnetometers – tiny quantum sensors based on nitrogen-vacancy defects in diamond – to detect defects and stress in metals. Researchers have shown that quantum magnetometers can “detect and visualize the tiniest damage in ferromagnetic materials”, like early-stage micro-cracks or fatigue, well before they grow into visible flaws. These sensors work by measuring subtle changes in magnetic fields caused by stress-induced changes in a material’s microstructure. In aerospace and automotive engineering, such a capability is a game-changer for safety and maintenance: components can be tested non-destructively with sensitivities far exceeding conventional techniques. For example, a quantum magnetometer array can scan an aircraft wing or a pipeline and pinpoint nanoscale corrosion sites or stress points that standard ultrasonic or visual inspections would miss. The Fraunhofer IAF’s QMag project in Germany recently demonstrated that NV-diamond magnetometers detect fatigue damage in steel samples much earlier than traditional sensors, and even reduced the time needed for testing. They also found these quantum sensors useful for quality control in microelectronics – detecting faulty transistors by mapping tiny magnetic signatures in circuits.
Quantum sensors are also boosting spectroscopic and microscopic techniques. In spectroscopy, researchers are developing quantum-enhanced spectroscopy where entangled photons or squeezed light states improve sensitivity. For instance, entangled photon IR spectroscopy can surpass classical limits of detection, allowing chemists to identify trace impurities or characterize reactions with fewer photons and lower noise. This could be useful in chemical engineering for monitoring processes in real-time with high precision (imagine being able to see intermediate species in a reactor in ultra-low concentrations). In microscopy, quantum illumination techniques use entangled light or single-photon probes to achieve better contrast when imaging materials, even in noisy environments. There is research into quantum-enhanced MRI and NMR (nuclear magnetic resonance) for materials as well – for example, hyperpolarizing nuclei via quantum means to get stronger signals for NMR spectroscopy. This would let scientists study surfaces and interfaces with NMR that are currently too insensitive to analyze. Additionally, atomic-scale sensors like scanning tunneling microscopes (STM) are being augmented with quantum bits to sense forces or fields at individual atom resolution. All these sensor advances complement quantum computing: while quantum computers simulate material behavior, quantum sensors directly measure it, and the two together give a powerful feedback loop. In practical terms, we’ll have manufacturing tools that can detect a single-atom defect in a semiconductor wafer or a single molecule of a contaminant in a chemical product. Such capabilities will dramatically improve quality control and R&D feedback in material fabrication. Quantum sensing is thus an integral part of the quantum revolution in materials science – quietly providing the eyes and ears at the nanoscale, while quantum computing provides the brain to interpret and predict what those eyes see.
Sustainable & Green Chemistry
Sustainability is a growing priority across the chemical engineering and materials sectors. Here, quantum computing could play a pivotal role by enabling cleaner, more efficient chemical processes and helping to design environmentally friendly materials. One angle is process optimization: many industrial chemical processes (like ammonia synthesis for fertilizers, petrochemical refining, or polymer production) operate under suboptimal conditions and produce unwanted byproducts because we lack perfect understanding of the chemistry. Quantum simulations can help identify reaction pathways that yield higher selectivity and lower waste. For example, the ARPA-E QC3 program specifically calls out the goal of discovering catalysts that could significantly reduce greenhouse emissions. By simulating catalyst behavior with quantum precision, researchers aim to develop catalysts that, say, allow ammonia production at lower temperatures (cutting energy use and CO₂ emissions) or that convert CO₂ into useful chemicals efficiently. Early quantum studies have targeted carbon capture as well – looking at materials that can absorb CO₂ or catalyze its conversion to fuels. Quantum computing can evaluate how CO₂ molecules interact with novel absorbent materials or metal-organic frameworks, guiding chemists towards designs that capture more CO₂ with less energy. In fact, engineers have noted that current fertilizer catalysts (for ammonia synthesis) account for about 2% of global CO₂ emissions, and quantum computing could help “develop more sustainable catalysts with a lower environmental impact,” as well as improved carbon capture materials. A concrete outcome would be a catalyst that enables ammonia production using less fossil fuel, or a sorbent that scrubs CO₂ from industrial flue gas more effectively – breakthroughs that directly curb emissions.
Another aspect of green chemistry is developing sustainable materials to replace hazardous or scarce substances. Quantum computing can assist in designing alternatives – for instance, finding a quantum-derived recipe for a polymer that is biodegradable yet has the performance of a conventional plastic, or designing corrosion inhibitors that are non-toxic. Researchers have started investigating corrosion inhibitors using quantum chemistry methods, with the aim of formulating coatings that prevent rust without the harmful pigments and additives used today. Likewise, quantum-driven discovery of solar cell materials could lead to more efficient photovoltaics made from Earth-abundant elements, accelerating the transition to renewable energy. On the process side, quantum algorithms can optimize reaction networks for biomass conversion or recycling processes, potentially revealing pathways that yield less hazardous waste. For example, a quantum computer might find a catalyst or a sequence of reactions that converts a bio-based feedstock to a valuable product in fewer steps (reducing solvents and waste). What makes quantum computing especially relevant to sustainability is its ability to handle the complexity of real-world chemistry – multi-step reactions, solvent effects, many interacting molecules – which is where many inefficiencies and pollution issues arise. Classical models often simplify these complexities and thus miss opportunities for improvement. Quantum models, by capturing more detail, could unveil “green” operating regimes (temperatures, pressures, catalysts) that minimize byproducts or energy usage. In summary, the advent of quantum computing aligns perfectly with the needs of green chemistry: both seek smarter, more precise control of chemical processes. As fault-tolerant quantum machines become available, we can expect a flurry of activity in redesigning industrial processes for sustainability – from cleaner production of chemicals and fuels to the creation of entirely new eco-friendly materials – effectively using quantum computing as a tool for environmental innovation.
The Arrival of Universal Quantum Computing
Many of the advances discussed are happening on today’s “noisy” quantum hardware. But the true transformation will unfold when universal, fault-tolerant quantum computers – machines with thousands of stable, error-corrected qubits – come online. These future quantum computers will be able to perform sustained, complex calculations that are impossible now, unleashing a new era for materials science and chemical engineering. With fault-tolerant quantum computing, scientists could accurately model “the most complex molecules – a task the largest imaginable classical computers could not solve,” as a Microsoft Azure Quantum project put it. In that envisioned future, a researcher could run a simulation of a protein with hundreds of atoms in full quantum detail, or determine the exact ground-state energy of a high-temperature superconductor compound – computations so vast that classical supercomputers (even with centuries of time) would fail to brute-force them. The impact will be both disruptive and profoundly beneficial. On the disruptive side, quantum computing may render many classical modeling techniques obsolete. Entire subfields like approximate quantum chemistry or molecular dynamics might be upended as quantum computers can give essentially exact answers for large systems. This could challenge companies that are built around classical simulation software to reinvent themselves for the quantum era. Companies that leverage universal quantum computers early for R&D may leap ahead, creating a competitive gap. For example, a materials company that discovers a room-temperature superconductor or an ultra-efficient battery via quantum computation will hold a massive advantage in the market. Traditional R&D timelines (often a decade or more for a new material or drug) could shrink dramatically – as Satya Nadella quipped, the goal is to “compress the next 250 years of chemistry and materials science progress into the next 25.” That kind of acceleration in innovation would be disruptive in a positive sense, acting as a catalyst for technological leaps (and also possibly economic shifts, as new quantum-designed products replace old ones).
The benefits of universal quantum computing for these sectors are difficult to overstate. Consider some scenarios: A fault-tolerant quantum computer could solve the electronic structure of a complex enzyme’s active site (like the famous FeMoco cluster in nitrogenase that catalyzes natural fertilizer production), providing insight to finally synthesize an artificial catalyst for ammonia at mild conditions – a holy grail of green chemistry. It could unravel the mechanism of high-temperature superconductivity by simulating the Hubbard model or other quantum materials with high accuracy, potentially guiding the intentional design of a superconductor that works at room temperature and revolutionizes energy transmission. It might optimize a multi-step chemical plant process end-to-end: feedstocks, intermediates, catalysts, and conditions, all tuned to maximize yield and minimize waste, something currently approached via heuristics and lots of testing. In drug discovery, a universal quantum computer could virtually screen and predict the efficacy of tens of thousands of drug candidates by computing binding free energies precisely, delivering drug leads in weeks where it used to take years. These machines will also be able to tackle “multi-metal systems” and other hard cases where classical methods hit a wall. Notably, they can explore phenomena like quantum tunneling in reactions or entangled states in materials that classical simulations often have to ignore or approximate. The arrival of such powerful computers will also synergize with AI and machine learning – quantum computers can feed highly accurate data into machine learning models (e.g. for material property prediction), making those models far more reliable.
Of course, realizing this future requires overcoming significant technological challenges (quantum error correction, scaling qubit counts, etc.), but steady progress is being made. Researchers anticipate that in the next 5–10 years we may see prototype fault-tolerant systems coming online. As they do, the materials and chemical sectors are likely to be among the first beneficiaries. In practical terms, the R&D workflow in these industries could fundamentally change: instead of starting with experimental lab work and later validating with simulations, future scientists might first perform exhaustive quantum simulations of a new material or reaction on a quantum supercomputer, then only do minimal confirmatory experiments in the lab. This inversion of the discovery process – compute first, experiment second – will save time, money, and reduce trial-and-error. It also democratizes discovery; for instance, a small startup with access to a cloud-based quantum computer could design a breakthrough polymer without needing a giant lab. However, this also means industries must adapt: the workforce will need new skills (quantum algorithms, interpreting quantum simulation output), and companies will have to invest in quantum infrastructure or cloud services. Ultimately, universal quantum computing will be both a disruptive force and a huge boon – disruptive in that it will challenge the status quo and existing tools, but overwhelmingly beneficial by opening doors to innovations that were out of reach with classical technology.
Sector Preparation & Responses
Research institutions, companies, and governments worldwide are actively preparing for these coming quantum breakthroughs in materials and chemical engineering. A key focus is building a “quantum-ready” workforce and knowledge base. Universities are launching interdisciplinary quantum programs that blend physics, chemistry, materials science, and engineering. For example, the University of Texas at Austin recently announced a new Texas Quantum Institute to bridge basic research and applied science, explicitly aiming to foster quantum-based innovation in energy, electronics, materials and more. The institute brings together physicists, chemists, and engineers under one roof, reflecting the recognition that breakthroughs often happen at the intersection of quantum computing and materials expertise. Similarly, national research centers have been established: in the U.S., five DOE Quantum Information Science Research Centers were founded in 2020, each involving multiple labs and universities, to advance quantum computing and sensing for various domains. In their first four years, these centers trained over a thousand students and early-career researchers through workshops and summer schools, seeding industry and academia with young scientists fluent in quantum algorithms and their applications to chemistry and materials. They even launched an annual Quantum Summer School to connect academia, national labs, and industry in building a quantum-savvy community. Governments outside the U.S. are no less active – the EU’s Quantum Flagship program includes projects on quantum simulation for chemistry, and countries like China, Japan, and Australia have national quantum initiatives that incorporate materials science use cases. This concerted global effort is ensuring that when quantum hardware is ready, there won’t be a lack of human talent or foundational research to apply it effectively.
Industry leaders in chemicals, pharma, and materials are also taking proactive steps. Many have formed dedicated quantum research teams or partnerships to explore near-term benefits. We mentioned how Mitsubishi Chemical and JSR joined IBM’s quantum network – this gives them hands-on experience with quantum computers and a chance to develop custom quantum solutions for their product challenges. Likewise, Dow Chemical has collaborated with quantum software startups to study quantum algorithms for material simulations (like modeling chemical reactions on catalytic surfaces). Big pharmaceutical companies such as Roche and GlaxoSmithKline have research agreements with quantum computing firms to start integrating quantum methods into drug discovery pipelines. Even in aerospace and energy, corporations like Airbus, Total, and ExxonMobil have publicized quantum computing programs focused on their material and chemical problems (from optimizing fuel mixtures to designing new alloys). These early investments serve a dual purpose: first, to make sure the company is not left behind when quantum advantage arrives, and second, to potentially reap some early benefits on NISQ (noisy intermediate-scale quantum) devices. For instance, ExxonMobil has been using quantum algorithms for more predictive environmental modeling and searching for new materials for carbon capture, aligning with their long-term business in cleaner energy. Volkswagen’s multi-year quantum program we discussed is aimed squarely at gaining a competitive edge in EV battery technology by leveraging quantum computing ahead of its rivals. In essence, forward-looking companies are treating quantum readiness as part of their innovation strategy, much like the adoption of AI or high-performance computing in earlier decades.
Governments are facilitating these industry preparations through funding and policy. Public-private partnerships are common: for example, the UK’s Quantum Technologies Challenge has funded collaborations between quantum startups and manufacturing companies to explore quantum solutions in materials development. Governments are also investing in quantum infrastructure (like national quantum computing hubs where companies can experiment on quantum hardware) and creating frameworks for quantum IP and standards. Another important aspect of preparation is community-building and knowledge sharing. Industry consortiums are emerging – one example is the Quantum Economic Development Consortium (QED-C) in the US, which includes many materials and chemical companies working together to identify quantum use cases and requirements. Such groups help define benchmarks (e.g. what size quantum computer is needed to surpass classical methods for a catalyst simulation?) and share non-competitive knowledge so everyone can progress faster. Competitors in the chemical industry, for instance, might collaborate on foundational quantum algorithms for chemistry, recognizing that the real differentiation will come in proprietary data and specific implementations. Meanwhile, scientific conferences and journals are increasingly featuring quantum computing in chemical engineering sessions, indicating a cross-pollination of ideas.
Finally, education and retraining efforts are noteworthy. Some companies are upskilling their current workforce – training computational chemists or materials engineers in quantum computing basics. Universities are adapting curricula; an AIChE article pointed out that quantum computing will need to be incorporated into chemical engineering education to prepare the next generation of engineers. We see early signs of this: new textbook materials on quantum computing for chemists, online courses, and even specialized master’s programs focusing on quantum applications in chemistry/materials. All these measures reflect a broad consensus: quantum technology is coming to these industries, and those who prepare now will capitalize on its benefits, whereas those who ignore it risk falling behind when the technology matures. The learning curve is significant – quantum computing requires new ways of thinking – so preparation and practice now are viewed as essential. In summary, the sector’s response has been proactive and collaborative, spanning workforce development, partnerships, infrastructure, and education, to ensure that the revolutionary potential of quantum computing in materials science and chemical engineering can be fully realized when the hardware catches up with the vision.
Challenges and Risks
Despite the optimism, it’s important to acknowledge the significant challenges and risks on the road to integrating quantum computing into materials science and chemical engineering. Technical challenges remain the foremost concern. Current quantum computers (tens of qubits, error-prone operations) are still far from the scale needed to solve industrial-scale chemistry problems. As one chemical engineering perspective noted, today’s best quantum machines have on the order of 50–100 qubits, whereas a few hundred stable qubits would be required just to outperform classical computers on certain tasks. Quantum bits are extremely fragile – slightest noise can cause calculation errors – which means most algorithms must be short or use error mitigation, limiting the complexity of simulations we can run right now. Truly simulating a complex molecule or a bulk material might need thousands of logical (error-corrected) qubits, which could be a decade or more away. Until then, there’s a risk of overhyping results. If expectations aren’t managed, some in the industry might grow skeptical due to the so-called “quantum hype cycle.” It’s possible that near-term devices will not show clear advantage for useful material science problems, given how powerful classical chemistry software has become with clever approximations. Indeed, classical computational chemists have continuously raised the bar by finding new algorithms and tricks, so quantum needs to reach a high threshold of quality and scale to truly surpass classical methods in practice. This means there’s a risk that practical quantum advantage arrives later than hoped, potentially leading to disillusionment or reduced funding in the interim.
Another challenge is computational integration. Marrying quantum outputs with existing simulation workflows is non-trivial. Early quantum results (like an energy of a molecule from a small quantum processor) often need hybrid quantum-classical workflows to be useful. Engineers and researchers will need new software tools that integrate quantum algorithms with classical HPC, and developing user-friendly platforms is still underway. If these tools remain too hard to use or understand, adoption in industry could lag. There’s also the matter of validating quantum results – ensuring a quantum simulation is giving physically meaningful answers and not an artifact of noise or algorithmic error. Industries that are conservative (for good reason, when safety or millions of dollars are on the line) may be slow to trust quantum-derived insights until they are thoroughly validated by experiments or long experience. Workforce and knowledge gaps pose another risk: quantum computing is a specialized field, and currently there are relatively few experts who both understand quantum algorithms and have domain expertise in chemistry/materials. Bridging this gap through training will take time, and in the interim, companies might not fully grasp how to apply quantum solutions effectively. This is why education efforts are crucial – without enough skilled practitioners, even available quantum tools could be underutilized.
From an industrial adoption standpoint, there’s the risk of investment timing. If companies invest too early, they might spend on quantum efforts that don’t pay off quickly, potentially causing internal backlash or “quantum fatigue.” If they invest too late, they could scramble to catch up. Navigating this requires clear-eyed understanding of quantum tech roadmaps. Some firms might hedge by focusing on quantum-inspired algorithms (classical algorithms that mimic quantum ones) as a stopgap – but there’s a risk in conflating those with true quantum solutions. On the technical risk side, one cannot ignore that quantum computing is advancing on multiple hardware fronts (superconducting qubits, trapped ions, photonic, etc.), and it’s uncertain which will win out or if there will be accessible cloud quantum computers that meet industry needs. Companies may worry about betting on the wrong horse – investing in a particular quantum platform that doesn’t become the standard. Standards and interoperability issues may arise if different quantum systems have different programming models.
Security is a minor but sometimes noted concern: quantum computers in the long run threaten encryption protocols, but that’s more relevant to IT than to materials R&D. In our context, a more fitting angle is intellectual property – if quantum computing allows rapid discovery of materials, companies will need to rethink IP strategies (e.g., a competitor might quickly “invent around” a patented material if they have quantum capabilities). This could lead to a more fast-paced, competitive landscape in innovation.
Lastly, a socio-technical challenge is ensuring broad access. If only big corporations can afford quantum computing initially, there’s a risk that breakthroughs concentrate in a few hands. Governments and consortia are trying to mitigate this by providing cloud access or national facilities, but equitable access to quantum resources will be important so that a wide range of players (including academia and startups) can contribute solutions to materials and chemistry problems – especially those relating to global challenges like climate change.
In summary, while the trajectory is exciting, it’s not without obstacles: hardware needs to mature, classical-quantum integration must improve, the workforce must grow, and early adopters need to be cautious yet persistent. The next few years will likely involve a careful balancing act – pushing the frontiers of what’s possible with quantum in materials/chemistry, while pragmatically using classical methods for what remains out of reach, and steadily chipping away at the limitations. Those in the field are well aware of these challenges, and a lot of current research is devoted to overcoming them one by one, from better error-correcting codes to hybrid algorithms and educational initiatives.
Conclusion
Quantum computing and associated quantum technologies are on the cusp of ushering in a new era for materials science and chemical engineering. We began with Richard Feynman’s early insight that “nature isn’t classical… and if you want to make a simulation of nature, you’d better make it quantum mechanical.” After decades of development, that vision is becoming reality. Quantum computers – though still nascent – have already shown they can emulate the quantum behavior of molecules and materials in ways that classical computers never could, hinting at their tremendous potential. We explored how current developments, from government-funded programs to private collaborations, are laying the groundwork by tackling pilot problems: simulating small molecules, optimizing battery materials, analyzing corrosion reactions, and more. These are important stepping stones, demonstrating feasibility and teaching us how to formulate real-world problems for quantum machines. In various industry-specific use cases, we saw a common theme: whether it’s discovering novel materials (superconductors, catalysts, polymers), simulating chemical reactions and biological molecules, improving energy storage, preventing corrosion, or enhancing sensing and characterization – quantum technologies offer qualitatively new capabilities. They allow us to model complexity and precision that were previously unattainable, which in turn can drive innovations (like a new catalyst or drug molecule) that had remained out of reach.
The full impact will emerge with the advent of large-scale, error-corrected quantum computers. When those arrive, the effect on materials and chemical industries could be nothing short of revolutionary. Imagine solving in minutes a materials problem that would have taken a classical supercomputer longer than the age of the universe – that is the kind of speedup quantum computing promises for certain hard problems. With such power, R&D becomes faster and more predictive. Entire development cycles could be shortened from years to months, as quantum simulation and optimization quickly zero in on the best designs for experiments. This will enable a more intentional and targeted approach to innovation: instead of Edisonian trial-and-error, engineers can use quantum computers almost like oracles to guide them to the solutions that physics allows. The benefits to society could be immense: cleaner energy through better catalysts and batteries, new materials that make transportation or computing more efficient, breakthroughs in medicine through drugs discovered in silico, and more. It’s telling that experts foresee quantum computing helping solve urgent global challenges – for example, by finding efficient routes for carbon capture, water splitting for hydrogen fuel, or designing high-capacity batteries to store renewable energy. In that sense, quantum computing is more than a technological end in itself; it’s a means to amplify human ingenuity in addressing big problems.