D-Wave Claims Quantum Supremacy with Quantum Annealing
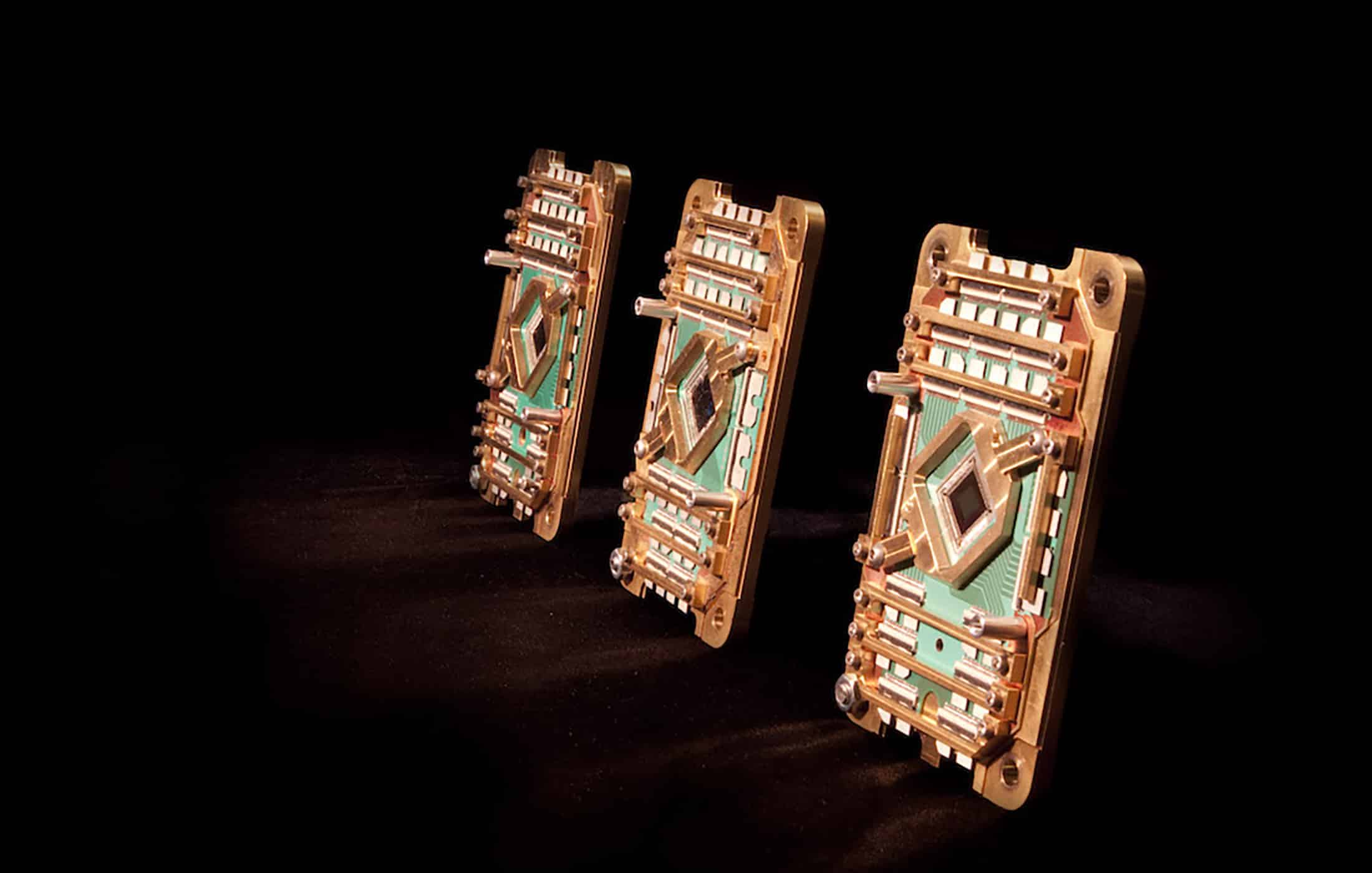
D-Wave has announced a breakthrough, claiming to achieve quantum computational advantage – even “quantum supremacy” – using its quantum annealing technology on a practical problem. In a peer-reviewed study published in Science on March 12, 2025, D-Wave’s researchers report that their 5,000+ qubit Advantage2 prototype quantum annealer outperformed one of the world’s most powerful supercomputers (Oak Ridge National Lab’s Frontier system) in simulating the quantum dynamics of a complex magnetic material. The task involved modeling programmable spin glass systems (a type of disordered magnetic material) relevant to materials science. According to D-Wave, their quantum machine found solutions in minutes that would take a classical supercomputer an estimated “nearly one million years” to match, a problem so intensive it would consume more power than the world’s annual energy supply if attempted classically. This dramatic speedup – solving in minutes what classical computing might never realistically solve – is being touted as the first-ever quantum advantage on a useful, real-world problem, distinguishing it from earlier quantum supremacy demonstrations that used abstract math problems or random circuit sampling.
D-Wave’s press release emphasizes the practical significance of the result. The simulation of spin glass materials has direct applications in materials discovery, electronics, and medical imaging, making it more than a mere computational stunt. The company notes that understanding magnetic material behavior at the quantum level is crucial for developing new technologies, and these simulations delivered important material properties that classical methods couldn’t feasibly obtain. The achievement was enabled by D-Wave’s Advantage2 annealing quantum computer prototype, which offers enhanced performance – including a faster “annealing” schedule, higher qubit connectivity, greater coherence, and an increased energy scale. These hardware improvements allowed the team to push the annealer into a highly quantum-coherent regime (reducing the effects of noise and thermal fluctuations) and tackle larger, more complex instances than previously possible.
D-Wave’s CEO, Alan Baratz, hailed the result as an industry milestone: “Our demonstration of quantum computational supremacy on a useful problem is an industry first. All other claims of quantum systems outperforming classical computers have been disputed or involved random number generation of no practical value.” This remark alludes to past high-profile “quantum supremacy” experiments (such as Google’s and USTC’s, which involved random circuit sampling and boson sampling respectively) that, while scientifically important, did not tackle practical applications. In contrast, D-Wave asserts that their annealing quantum computer is now “solving useful problems beyond the reach of the world’s most powerful supercomputers”, and notably, this capability is available to customers via D-Wave’s cloud service today. The company is framing the achievement as a validation of its alternative approach to quantum computing, marking the first time an analog quantum annealer has definitively beaten a classical supercomputer in a real-world computational challenge.
In-Depth Analysis and Context
What Did D-Wave Announce, and How Was Quantum Advantage Achieved?
In simple terms, D-Wave announced that its quantum annealing processor solved a problem that classical computers essentially cannot solve in any reasonable timeframe. The specific problem was simulating the quantum behavior of a 3D spin glass – imagine a 3-dimensional lattice of interacting magnetic spins where the goal is to find low-energy configurations or track their dynamics. This kind of simulation is extremely hard for classical algorithms as the system size grows, because it involves quantum interactions across thousands of spins (qubits). D-Wave’s team programmed their 5,000-qubit Advantage2 prototype to act as a programmable quantum spin glass and ran it for various lattice sizes and evolution times. In essence, they turned the quantum computer into a direct analog simulator of the material. The quantum annealer naturally evolves according to the same physics the spin glass obeys, so it can mimic the material’s behavior directly in hardware. The study reports that for the largest, most complex instances they tried, the quantum annealer produced results in about 36 microseconds of runtime (plus some overhead for repetitions), whereas Frontier – a top classical supercomputer with massive GPU clusters – would have needed on the order of 1012 seconds (nearly a million years) to simulate the same quantum dynamics with comparable accuracy. In practical terms, this is a beyond-classical result: no existing classical machine, not even a supercomputer, can match the annealer for that task.
How did the annealer achieve this? D-Wave credits both hardware advances and careful problem design. The Advantage2 prototype used in the experiment includes a “fast anneal” feature and improved qubit design. Faster annealing (shortening the duration of the quantum evolution) actually helped in this context: it reduced the window for environmental noise to interfere, thereby maintaining coherence (quantum order) through the critical part of the computation. Previous D-Wave machines often operated in a slower, more thermally-dominated regime, which blurred the quantum effects; by contrast, this experiment pushed the system into a more coherent, quantum-dominated regime with negligible thermal excitations. Additionally, the connectivity between qubits in the new processor is higher (Advantage2 uses D-Wave’s Pegasus topology where each qubit can connect to 15 others, and a next-gen Zephyr topology with up to 20 connections is planned). Higher connectivity meant the 3D lattice of the spin glass (where each spin interacts with several neighbors in the 3D grid) could be embedded more naturally onto the hardware graph, with fewer extra qubits needed to mediate long-range connections. In short, the annealer’s improvements in speed, coherence, and connectivity enabled it to handle a large, entangled quantum simulation that classical methods could not keep up with.
It’s worth noting that D-Wave’s approach here is somewhat different from running a conventional algorithm on a quantum computer. This was analog quantum simulation – essentially letting the quantum device behave like the system of interest – rather than a step-by-step algorithm with logic gates. The team did benchmark the results to ensure they were correct (for smaller instances they could compare against exact classical solutions or known physics), and then extrapolated to larger instances beyond classical solvability. They also analyzed scaling behavior: by studying how the solution time scales as the problem size grows, they found that the quantum annealing time scaled more favorably (faster growth exponent) than various classical algorithms (such as simulated annealing or path-integral quantum Monte Carlo). This indicates an asymptotic quantum speedup – a hallmark of quantum advantage – at least for this class of problem. Importantly, the D-Wave experiment delivered a useful outcome (properties of a magnetic material) while demonstrating the speedup, unlike earlier supremacy experiments which were essentially checking random outputs.
What is Quantum Annealing, and How Does It Differ from Gate-Based Quantum Computing?
Quantum annealing is a specialized approach to quantum computing that focuses on solving optimization and sampling problems by exploiting the natural tendencies of quantum systems. In quantum annealing, the computer’s qubits are all analogously evolving under a physical process: the system is initialized in an easy, low-energy state, then a Hamiltonian (energy landscape) representing the problem is gradually turned on. By slowly “annealing” (adjusting the quantum parameters), the system is supposed to remain in (or end up in) the lowest energy state of that problem Hamiltonian, which encodes the solution to the optimization problem. In practice, quantum tunneling and other quantum effects help the system escape local minima in the energy landscape and hopefully find a better solution than classical thermal fluctuations would find in a similar classical annealing process. One way to think of it: quantum annealing is like an analog computer that physically imitates the problem you want to solve – for example, finding the ground state of a spin glass is solved by literally having qubits behave as spins and letting the quantum physics do the work.
This is fundamentally different from gate-based (circuit-based) quantum computing, which is the model companies like Google, IBM, and others mostly pursue. In gate-based quantum computing, qubits are manipulated with discrete logic gates (analogous to classical logic gates but quantum). This model is universal – in theory a sequence of quantum gates can perform any computation (including those a quantum annealer does), and algorithms like Shor’s for factoring or Grover’s for search run on gate-based machines. Gate-based quantum computers are more flexible; they can create highly entangled states and don’t have to always find ground states – they can implement error correction, run arbitrary circuits, etc. However, current gate-model machines have far fewer qubits (tens or hundreds, versus D-Wave’s thousands) and each gate operation is prone to error unless corrected. They require a lot of overhead for error correction which is still being developed. By contrast, annealers trade generality for scale and stability – D-Wave can have thousands of qubits because each qubit just needs to follow the analog annealing process, which is easier to scale, and errors manifest differently (often as ending in an excited state rather than a wrong logical bit flip). Annealing is best suited for certain problems (like combinatorial optimization or sampling from complicated distributions) and not able to run algorithms like Shor’s algorithm for breaking encryption. In summary:
- Quantum Annealing (Analog): Uses a gradual evolution of a quantum system to find minimum-energy solutions. Large number of qubits but limited to solving optimization/sampling problems formulated as Ising models or QUBOs (quadratic unconstrained binary optimization). Less flexible, but currently more qubits and easier to scale hardware.
- Gate-Based Quantum Computing (Digital): Uses sequences of quantum gate operations on qubits to perform arbitrary algorithms. In principle can solve a wider range of problems (including all that annealers can) with enough qubits and low error rates. However, current devices have fewer qubits and require error correction for scaling. More flexible but more challenging to scale in the near term.
Notably, the gap between these approaches has started to blur a bit: some gate-model quantum computers have demonstrated small optimization algorithms, and conversely D-Wave’s annealers do perform some gate-like calibrations and could potentially be used in hybrid algorithms. But by and large, D-Wave’s annealer achieving quantum advantage is a milestone for the analog/annealing paradigm, whereas previous claims of quantum advantage were achieved on gate-model or photonic systems doing very different tasks.
The Controversy: Skepticism and Academic Critiques of D-Wave’s Claim
D-Wave’s bold claim has naturally stirred debate in the quantum computing community. While the result is peer-reviewed and significant, some researchers question the interpretation and scope of this “quantum advantage.” One line of critique is that the D-Wave demonstration, impressive as it is, addresses a very narrow problem – essentially, a physics simulation of a spin glass – which may or may not translate to broader real-world applications. Indeed, the D-Wave researchers themselves acknowledge in the Science paper that “extending this…to industry-relevant optimization problems…would mark an important next step in practical quantum computing.” In other words, even D-Wave admits that solving practical business problems (e.g. in logistics, finance, etc.) on an annealer remains work for the future; so far, they have shown a quantum speedup on a physics problem that, while relevant to materials science, is still somewhat esoteric from an industry perspective.
Critics point out that D-Wave has a history of demonstrating speedups on Ising spin glass problems – an academic benchmark – without yet showing an advantage on a more directly useful problem that everyday industries care about. Over the past decade, D-Wave teams have published multiple papers in Nature, Science, etc., simulating spin glasses or related problems. These studies often leave the question, “okay, but when will we see a quantum speedup on a practical optimization like portfolio optimization or scheduling?” The latest work is a step closer (because materials simulation can be useful), but skeptics remain cautious.
Another critique centers on whether the playing field was truly fair in the comparison to classical computing. D-Wave compared their annealer against a brute-force simulation of quantum dynamics on a classical supercomputer, which indeed is hopelessly slow for large quantum systems. However, one could ask: is this an entirely surprising result, given that the quantum device is essentially designed to emulate the quantum system directly? In a sense, D-Wave built a special-purpose machine for this class of problem (just as an analog solver), so surpassing a general-purpose classical computer that’s trying to do the same physics in software is expected. Some researchers are investigating whether improved classical algorithms could narrow the gap. For instance, shortly after D-Wave’s announcement, a group of scientists proposed using time-dependent variational Monte Carlo algorithms to more efficiently simulate quantum annealing processes on classical machines. It’s an open question whether classical approximation methods might handle slightly smaller or simplified versions of the spin glass simulation, which could challenge the practical supremacy claim. So far, D-Wave’s million-year vs minutes advantage stands unchallenged, but history tells us that classical algorithms often catch up when a new quantum benchmark is set (as we saw with Google’s and USTC’s claims – more on that soon).
There’s also a philosophical dispute: what counts as a “useful problem”? D-Wave is heavily promoting that this is the first quantum supremacy demonstration for a useful problem, implicitly dismissing earlier experiments as useless. Some critics respond that D-Wave’s problem, while grounded in real physics, is still very specialized. It’s “useful” in a niche sense (materials scientists studying spin systems care, as might certain niche applications in magnetism), but it’s not a broad commercial problem like optimizing supply chains or machine learning. Moreover, mapping many real-world problems onto the form solvable by D-Wave’s annealer is non-trivial. Quantum annealers can only natively handle problems expressible as quadratic interactions (pairwise couplings) between bits. If a problem has more complex relationships, one must introduce auxiliary qubits – a process called quadratization – which can blow up the problem size dramatically. For example, one analysis noted that factoring a large number (RSA-230) could be encoded as an Ising spin problem with about 5,893 spins if higher-order interactions are allowed, but if you restrict to only pairwise interactions (as D-Wave’s hardware does), it would require on the order of 148,000 qubits after adding auxiliary variables. This kind of overhead can erase any speed advantage. D-Wave’s current machines have at most ~5,000 qubits, so they cannot tackle such embedded problems yet. Additionally, even if the problem is quadratic, the connectivity constraints of the hardware mean one often has to embed a logical fully-connected graph onto the sparse hardware graph, using chains of physical qubits. This overhead also reduces the effective size of problem you can solve. The D-Wave Advantage2 (Pegasus topology) allows each qubit to connect to at most 15 others, which is still a far cry from an “all-to-all” connectivity many algorithms assume. These limitations lead some researchers to argue that quantum annealers face scaling challenges for truly complex, real-world instances, even if they show promise on physics simulations.
In short, while no one denies the D-Wave experiment shows a quantum machine doing something remarkable, skeptics question how much it will impact real-world applications outside of physics simulations. They call for cautious interpretation: the result is a milestone, but we should be careful about the “first useful quantum supremacy” narrative until we see quantum annealers tackling a wider range of industry problems.
Comparison to Past Quantum Advantage/Supremacy Claims (Google, USTC, etc.)
D-Wave’s claim arrives in the context of several prior “quantum supremacy” or “quantum advantage” announcements in recent years. It’s helpful to compare them:
- Google’s “Sycamore” (2019) – Google’s 53-qubit superconducting gate-based processor performed a random circuit sampling task (basically checking the output of a random quantum circuit) in about 200 seconds. In their Nature paper, Google estimated that the same task would take a state-of-the-art classical supercomputer approximately 10,000 years to simulate. This was heralded as the first experimental quantum supremacy. However, IBM immediately challenged the claim by suggesting a better simulation method: IBM argued their Summit supercomputer could do it in roughly 2.5 days by using more clever techniques and disk storage to extend memor. Even if 2.5 days is accepted, that’s still a quantum speedup (200 seconds vs ~60 hours), but the episode underscored that supremacy claims can be fragile, depending on what you assume about classical algorithms. Importantly, Google’s task had no practical application beyond being a proof-of-concept; it was essentially random number generation. The outcome didn’t directly solve any useful problem – it just proved the quantum hardware could do something very hard to simulate.
- USTC’s “Jiuzhang” (2020, 2021) – A team at University of Science and Technology of China (USTC) led by Pan Jianwei achieved quantum advantage using photonic quantum computers that implement boson sampling (specifically Gaussian boson sampling). In late 2020, their Jiuzhang 1.0 system with 76 detected photons was reported to generate samples from a photonic circuit in a few minutes, a task they estimated would take a classical supercomputer on the order of 2.5 billion years to replicate. This was another astonishing demonstration of quantum speedup, and unlike Google’s qubits, photonic systems have very different error profiles. USTC followed up with Jiuzhang 2.0 (113 photons) pushing the boundary further in 2021. Again, boson sampling is a specialized problem (essentially a random matrix permanent calculation) with no known practical output – it’s mainly a way to showcase that the quantum device can explore Hilbert space much faster than classical simulations. Some scientists later debated whether the output of Jiuzhang was truly beyond classical simulation or if approximate algorithms could spoof the results; there were even papers suggesting alternative classical explanations for some aspects of the experiment Nonetheless, Jiuzhang’s achievement, like Google’s, remains a milestone in showing quantum machines outpacing classical ones on at least some task.
- Other notable claims – Since those landmarks, there have been a few additional quantum advantage claims. For instance, in 2022 Xanadu (Canada) used a photonic processor called Borealis to perform another boson sampling experiment, claiming a significant speedup over classical methods for that task. IBM has not explicitly claimed “quantum supremacy,” but they have demonstrated quantum advantage in specific scenarios such as certain small chemistry simulations via hybrid algorithms, and they continue to push quantum volume and error-corrected logical qubits rather than making a supremacy declaration. There was also a 2023 report by IonQ claiming its trapped-ion system beat classical on a magnetism simulation, though on a very small scale – these are early steps and often debated. None of these had the dramatic gap that Google or USTC did, and crucially none were both beyond classical and immediately useful in application.
In comparison, D-Wave’s new claim attempts to combine the strengths of these earlier milestones while addressing their shortcomings: like Google and USTC, D-Wave shows an astronomical speedup (millions of years vs minutes) but it also stresses that the problem has practical relevance. The spin glass simulation yields insights into material properties, arguably more directly useful than random circuit samples or boson samples. D-Wave’s experiment also used far more qubits (5000 vs 50-100 in the prior cases), though being analog qubits of a different type. It’s also notable that D-Wave’s result was achieved on a commercially oriented machine available (in prototype form) via cloud, whereas Google’s and USTC’s were lab experiments not accessible to end-users.
However, it’s worth tempering the comparison: Google’s and USTC’s experiments tested the universality and control of gate-model and photonic systems – they showed those qubit systems can be pushed to regimes of complexity that are hard to simulate, which is a huge validation for those technologies. D-Wave’s annealer is a more narrow device in terms of what it can do; it’s extremely powerful for problems that map onto it (like the spin glass here), but it cannot run arbitrary algorithms. So each of these achievements is significant in its own domain. The quantum advantage demonstrations so far have each been somewhat isolated to particular tasks: random circuit sampling, boson sampling, and now annealing-based spin glass simulation. The long-term quest is to extend quantum advantage to broader, useful tasks that directly impact industry or society. D-Wave’s claim is a step in that direction, though it will likely be dissected and tested by the community just as Google’s and USTC’s were.
Broader Implications for Quantum Computing Credibility (Post-Microsoft Majorana Controversy)
The fanfare around D-Wave’s announcement comes at a time when the credibility of quantum computing research is under the microscope, in part due to some high-profile controversies. One such episode is the recent “Microsoft Majorana-1” controversy. Microsoft has been pursuing a very different approach to quantum computing – trying to create topological qubits based on Majorana fermions (exotic quasiparticles that could enable more stable qubits). In 2018, a Microsoft-affiliated academic team published a paper claiming they had observed Majorana particles – a breakthrough for the field – only for that paper to be retracted in 2021 after other scientists found problems with the data. Fast forward to early 2025, Microsoft announced a new breakthrough: a device called “Majorana 1” with supposedly the first ever topological qubits. This time too, experts quickly voiced skepticism, noting that the fundamental physics is still not conclusively proven and that Microsoft’s claims lack some crucial details. In the words of one physicist, Microsoft was making “a piece of alleged technology based on basic physics that has not been established.” Such incidents cast a shadow – they remind everyone that extraordinary claims require extraordinary evidence, and the quantum field is not immune to hype or even errors that can mislead if not checked.
In this climate, D-Wave’s claim of quantum advantage with annealing will be met with both excitement and healthy skepticism. The broader quantum community will look for independent validation of D-Wave’s results and methods. The good news for D-Wave is that their work was published in a major peer-reviewed journal (Science), suggesting it passed scrutiny of reviewers. Additionally, because their machine is accessible (via cloud) to some degree, other researchers might attempt to reproduce or at least validate aspects of the experiment (within the limits of access). This transparency is important – one reason the Google and USTC claims gained acceptance is that other groups could verify parts of them (or at least verify the classical difficulty of reproducing them). D-Wave has an opportunity to build credibility if the result holds up and if they engage openly with the scientific community’s questions (for example, publishing detailed data, inviting challenges to their classical “one million year” estimate, etc.).
The D-Wave achievement, if borne out, bolsters the argument that quantum computing is indeed making progress toward practical impact. Each time a quantum machine beats the best classical efforts, it adds confidence that quantum computers aren’t just a theorist’s dream but a real, emerging technology. It’s also a validation for the often underrated annealing approach – D-Wave’s long-game with annealing is paying dividends just as some in the field were questioning if gate-model quantum computers would leapfrog it entirely.
On the other hand, if any aspect of D-Wave’s claims were found to be overstated or if later research showed a classical workaround that cuts that “million-year” gap down dramatically, it would be a setback for quantum advantage claims. The field has to be careful about messaging. Overhyping results can backfire – as seen in the Microsoft case, where a retraced claim damaged trust and gave fuel to skeptics of quantum computing. D-Wave’s explicit branding of this result as the “first and only quantum supremacy on a useful problem” is bold and will invite scrutiny from competitors and academics. Already, some observers note that calling it “supremacy” (a loaded term) might be premature until the result is widely confirmed or until it’s clear no new classical algorithm can challenge it. Many prefer the term “quantum advantage” for now, reserving “supremacy” for an unassailable lead.
In summary, the implications of D-Wave’s quantum advantage claim are significant: it suggests we are entering an era where quantum computers tackle meaningful problems better than classical computers. If the claim holds, it will energize investment and research in non-gate-model quantum computing and could lead to more attention on analog quantum simulators for practical tasks. It also raises interesting questions about the value of specialized vs. general quantum computing approaches. D-Wave’s success doesn’t immediately translate to gate-based quantum computers solving broader problems – it might be that different quantum hardware will excel at different tasks (annealers for optimization, photonic systems for sampling, gate-based for factoring and general algorithms, etc.) This could shape a landscape where quantum computing isn’t one-size-fits-all, but rather a suite of quantum technologies each with its niche.
Finally, the community will be weighing this result alongside claims from big players. Trust and verification are crucial. In the wake of episodes like the Majorana controversy, researchers are likely to dig into D-Wave’s data and methods to ensure everything is sound. A key takeaway is that quantum computing has matured to a point where bold claims are being made and tested regularly – a healthy sign of progress, as long as we remain cautious and scientific rigor prevails over hype.