Quantum Use Cases in Pharma & Biotech
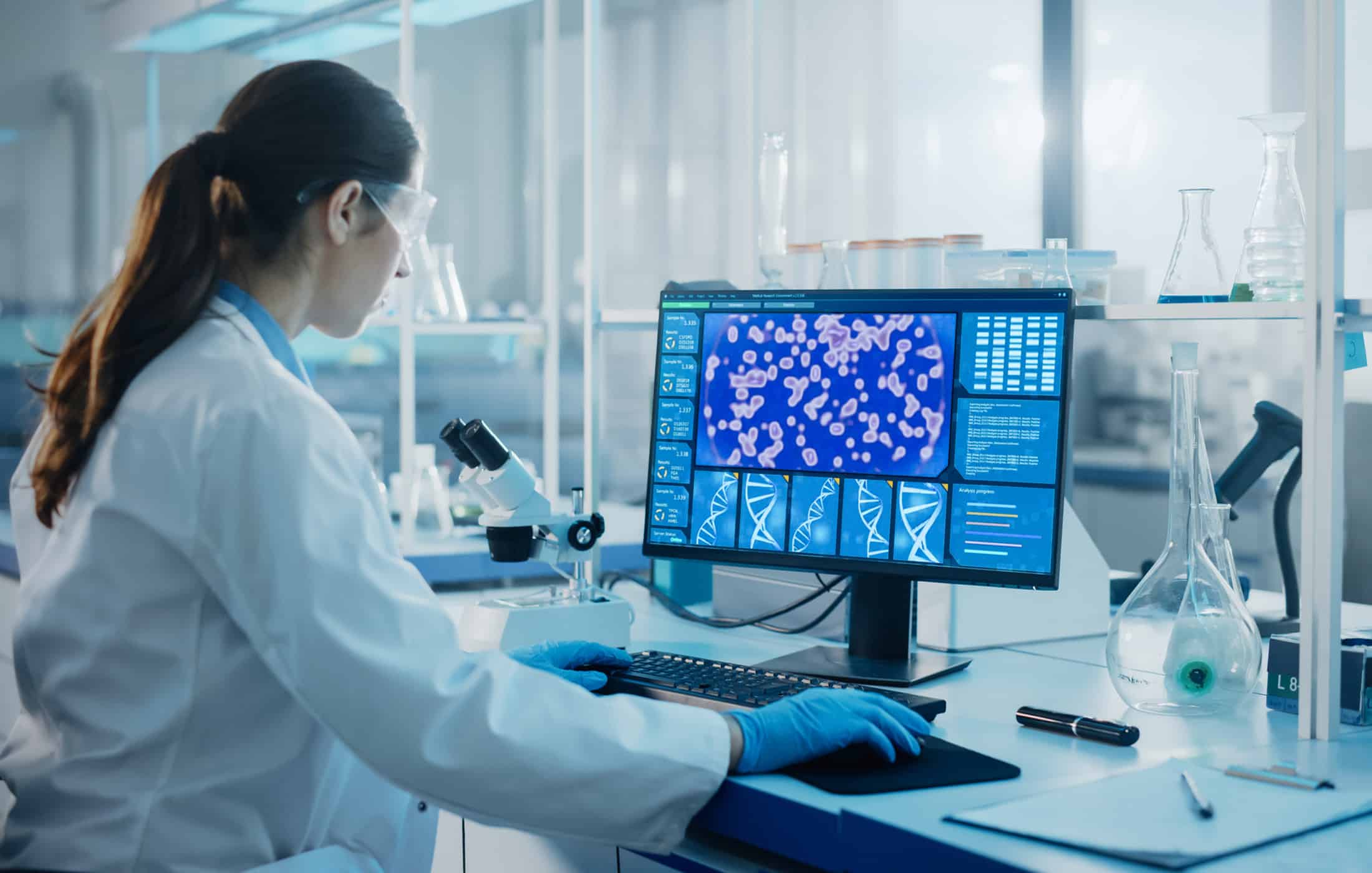
Table of Contents
Introduction
Quantum computing harnesses the counterintuitive principles of quantum mechanics to process information in ways that classical computers cannot. Unlike classical bits, quantum bits (qubits) can exist in superposition (multiple states at once) and become entangled, allowing quantum computers to evaluate many possibilities simultaneously. This capability gives quantum computers the potential to solve complex, multi-variable problems exponentially faster than conventional machines. In the pharmaceuticals and biotechnology sectors – where discovery and innovation often hinge on untangling extremely complex molecular interactions and massive biological datasets – such computing power is a game-changer. Pharma companies spend up to 15% of sales on R&D, yet many biological problems (from protein folding to drug-target interactions) are so complex that classical algorithms struggle with accuracy and speed. Quantum computing promises to push past those limits, enabling more precise simulations and data analyses that could revolutionize drug development and healthcare delivery. Industry estimates suggest the life sciences and chemistry fields alone could reap over $1.3 trillion in value by 2035 from quantum technologies, underscoring why quantum computing is capturing the imagination of pharmaceutical and biotech leaders worldwide.
Quantum computing matters for pharma and biotech because at its core, medicine is an information science dealing with inherently quantum systems. Molecules, proteins, and biochemical reactions operate under quantum physics – and thus a quantum computer can, in principle, model these with far greater fidelity. This means researchers might simulate drug molecules and their behavior in the body with unprecedented accuracy, potentially predicting efficacy and side effects before a lab experiment is ever done. Beyond drug chemistry, quantum computers’ ability to rapidly analyze enormous datasets could transform fields like genomics and personalized medicine. It could enable finding subtle patterns in patient data or genetic profiles that classical computing would miss, leading to highly targeted therapies. In short, quantum computing offers a path to faster discovery, more personalized treatments, and solutions to biomedical problems that were previously intractable – making it a seminal technology on the horizon of healthcare.
Current Developments
Though universal quantum computers are still in their infancy, we are already seeing significant activity at the intersection of quantum tech and the life sciences. Early proof-of-concept results have demonstrated that even today’s prototype quantum devices can tackle certain pharmaceutical problems. For example, D-Wave (a quantum annealing company) has shown faster molecular simulations for drug research, and IBM’s quantum systems have been used to model chemical reactions in the hunt for new drugs. These initial applications, while limited to relatively small molecules or simplified models, mark the first steps toward integrating quantum computing into drug R&D. Academic and corporate labs worldwide are actively exploring “quantum chemistry” techniques to validate that current quantum hardware can indeed reproduce molecular properties and reaction dynamics that are challenging for classical supercomputers. The encouraging results to date – such as Google’s quantum processor successfully simulating a simple chemical reaction – have spurred a wave of investment and experimentation in pharma-focused quantum computing.
Public-private collaborations and consortiums are driving many of the advances in quantum biotechnology. In the United States, the Cleveland Clinic partnered with IBM to launch the Discovery Accelerator, a 10-year initiative to apply quantum computing (alongside AI) to biomedical research and drug discovery. Likewise, pharmaceutical giant Merck has been actively engaging in quantum research: Merck KGaA in Germany joined a UK-led consortium with Oxford University and startup SEEQC to build a full-stack quantum computer specifically for drug development, backed by a £6.8 million government grant. Another example is a partnership between Boehringer Ingelheim and Google Quantum AI, focusing on quantum use cases in pharmaceutical R&D. Major tech firms are offering their quantum platforms to biotech researchers via the cloud – IBM’s Quantum Network, Microsoft’s Azure Quantum, and Amazon’s Braket all have pharma companies as collaborators. These collaborations give drug researchers early access to cutting-edge quantum hardware and expertise, while tech companies learn which real-world biomedical problems are most tractable. On the startup front, dozens of quantum computing companies and biotech firms are teaming up: DNA analysis firm Strangeworks and protein simulation startup ProteinQure, for instance, or quantum software companies like Zapata Computing working with drug makers to test hybrid quantum-classical algorithms for clinical trial design. Notably, about 80% of biotech startups exploring quantum computing are targeting drug discovery applications, reflecting intense focus on this use case.
Industry interest is further evidenced by patent and investment trends. Pharma leaders such as Merck, Johnson & Johnson, Roche, and Amgen filed multiple patents related to quantum computing in 2022-2023, indicating active internal research. Vaccine maker Moderna forged a partnership with IBM to investigate how quantum computing and generative AI could improve mRNA medicine design. Similarly, AstraZeneca and Sanofi teamed up with Alphabet’s quantum spin-off SandboxAQ to explore quantum applications in drug development. Meanwhile, alliances like QuPharm have formed – a consortium of major biopharma companies collaborating to develop pre-competitive quantum computing solutions for pharma. QuPharm, working with groups like the Pistoia Alliance (a pharma IT consortium) and the U.S. Quantum Economic Development Consortium, has already brainstormed dozens of quantum use cases from target identification to clinical trial optimization. Governments are also supporting these efforts: the EU’s Quantum Technologies Flagship and EuroHPC initiatives include projects aimed at pharma applications, and national R&D programs in the US, UK, and China are funding research into quantum techniques for biomedical imaging, genomics, and more. In short, the quantum revolution in pharma is well underway – not in full bloom, but with significant “seed” projects and partnerships indicating what’s to come.
Industry-Specific Use Cases
Quantum technologies stand to impact nearly every stage of the pharmaceutical and biotechnology pipeline. Below we break down key application areas where quantum computing (and quantum sensing) are being applied or studied today:
Drug Discovery and Molecular Simulations
Perhaps the most talked-about application is using quantum computers to improve drug discovery, especially in simulating molecules and chemical reactions. Classical computer models struggle with the quantum nature of chemistry – the behavior of electrons in atoms and molecules. As a molecule grows in size, the number of interacting electrons explodes in complexity, quickly exceeding what conventional algorithms can handle exactly. Today’s drug designers rely on approximations (like molecular dynamics or density functional theory) that can be slow and sometimes inaccurate for complex targets. Quantum computing offers a fundamentally new approach: since molecules themselves are quantum systems, a quantum computer can natively represent and analyze their quantum states. In theory, a sufficiently powerful quantum computer could simulate a molecule’s full electronic structure and reactivity exactly, something impossible for a classical supercomputer. While that ultimate goal is still years away, even current quantum processors have demonstrated the ability to calculate molecular energies and optimize small drug-like molecules more efficiently than classical methods in certain cases. By evaluating many molecular configurations simultaneously via superposition, quantum algorithms can scan the potential “chemical space” of new compounds much faster.
In practical terms, quantum computing could dramatically accelerate the early phases of drug R&D. Screening libraries of candidate molecules for a desired biological activity – which normally involves testing tens of thousands of compounds – might be expedited by quantum algorithms that identify promising hits with far fewer experiments. For instance, researchers have shown that a quantum annealer can generate novel drug-like molecules via fragment-based design, exploring billions of possibilities in weeks rather than years. Quantum computers are also being used to refine lead compounds: in one case, quantum algorithms optimized the molecular structure of drug candidates to improve efficacy and reduce side effects. Such approaches not only speed up the process but can produce better-quality drug leads.
Even the nuanced process of protein-ligand binding – how a drug molecule fits into its target protein – is getting a quantum boost. A recent collaboration between Pasqal (a quantum computing firm) and Qubit Pharmaceuticals used a neutral-atom quantum computer to model the role of water molecules inside protein binding pockets, something classical simulations find very challenging. They successfully placed water molecules in a protein binding site using a quantum algorithm on real hardware – reportedly the first time a quantum computer tackled a molecular biology task of this complexity. IBM researchers likewise are using quantum computers to calculate drug-protein binding affinities and toxicities more efficiently than classical simulations.
By improving the accuracy of these in silico predictions, quantum tools could help chemists “fail fast” – ruling out poor drug candidates early – and focus resources on the most promising leads. Ultimately, experts predict that as quantum hardware scales, it could cut years off the drug discovery timeline and save hundreds of millions in R&D costs. Indeed, theoretical models suggest quantum computing might reduce drug development times by up to 50–70% in the coming decades, an impact that would be profoundly disruptive (in a good way) for pharmaceutical innovation.
Personalized Medicine and Genomic Analysis
Another transformative use case is applying quantum computing to personalized medicine – tailoring treatments to an individual’s genetic and molecular profile. Modern healthcare is awash in genomic data: sequencing a single person’s genome produces billions of data points. Add to that other “omics” (transcriptomics, proteomics, metabolomics) and health records, and the datasets become staggeringly complex. Quantum computing’s ability to handle large, complex datasets offers a way to make sense of this information deluge. For example, quantum algorithms could sift through massive genomic databases to discover subtle genetic markers associated with a disease or a patient’s likely response to a drug. These are patterns that might be indiscernible to classical data-mining techniques but pop out when analyzed with quantum-assisted optimization or quantum-enhanced machine learning. Researchers have noted that quantum computers, with their parallelism, could identify correlations in genomic data orders of magnitude faster than conventional systems, vastly speeding up biomarker discovery.
The end-goal is truly personalized treatment plans. In cancer therapy, for instance, doctors could use a patient’s tumor genome and other health data to determine which drug or combination will work best – a complex decision requiring evaluation of many variables. Quantum computing can help by rapidly evaluating how different genetic mutations might influence the efficacy of various treatments. Early studies have shown quantum models improving the precision of cancer therapy selection: one quantum-driven model predicted how cancer cells with specific genetic mutations would respond to a panel of drugs, enabling clinicians to pick the most effective therapy for that patient. Beyond cancer, similar approaches could optimize treatment for anything from rare genetic disorders (by analyzing how a particular mutation alters protein function and which drug can counteract it) to common conditions like diabetes (by stratifying patients into subgroups based on genomic and lifestyle factors). Quantum computing might also streamline the search for optimal drug dosages or multi-drug regimens personalized to an individual’s metabolism. In essence, it brings us closer to the vision of computationally testing treatments on a virtual twin of the patient – encompassing their genetic makeup and even simulating their biochemistry – before administering the therapy in real life.
Another facet of personalized medicine is preventative: predicting disease risk and catching illnesses early. Quantum-enhanced data analysis could integrate genomic data with environmental and lifestyle data to improve risk models for diseases. For example, a quantum algorithm might analyze a person’s entire genome along with biomarkers (cholesterol, blood sugar, etc.) and medical history to detect patterns indicating a high risk of Alzheimer’s or heart disease long before symptoms appear. Quantum computers are well-suited for such high-dimensional analysis, where many factors interplay.
Additionally, quantum computing can aid in pharmacogenomics – understanding how genetic differences affect individual responses to drugs. By simulating drug metabolism pathways for different genetic variants, quantum computations could flag if a particular patient is likely to have an adverse reaction or need a higher/lower dose, enabling truly personalized prescriptions.
Real-world implementations are starting to form: the Cleveland Clinic-IBM partnership, for example, is working on a quantum computing platform to integrate genomic analysis into clinical decision-making, aiming to make these quantum-derived insights seamlessly usable by doctors.
Although today’s quantum hardware is not yet powerful enough to crunch all the data in a human genome at once (quantum-inspired algorithms are being used as a stopgap on classical machines for now), the trajectory suggests that in the near future, quantum computing will be a key enabler of personalized medicine – delivering the right treatment to the right patient at the right time, based on deep computational insight into their unique biology.
Quantum-Enhanced AI and Machine Learning for Healthcare
Quantum computing and artificial intelligence are complementary technologies, and their intersection – quantum machine learning (QML) – holds intriguing possibilities for healthcare. AI and machine learning are already ubiquitous in biotech (e.g. AI models for drug design, diagnostic image analysis, predicting protein structures), but they are limited by classical computing constraints. Quantum computers could supercharge certain AI algorithms, allowing them to explore more complex models or train on larger datasets in feasible time. One promising area is in medical imaging diagnostics: quantum algorithms are particularly suited for pattern recognition problems, which are at the heart of interpreting MRI scans, CT images, or even microscope images of cells. Researchers are developing QML techniques to improve the accuracy and speed of image-based diagnoses. For example, a quantum machine learning model might be able to detect extremely subtle anomalies in radiology images – early signs of cancer or neurological disease – that might elude even the best classical image-analysis AI. By processing the vast configuration space of pixel data in parallel, quantum-enhanced AI could reduce false negatives and catch diseases at earlier stages, when they’re more treatable.
Quantum computing can also enhance AI-driven drug discovery. Generative models (like GANs or variational autoencoders) are used to invent new molecular structures with desired properties – essentially algorithmic molecular design. Incorporating quantum computing into this process can improve the fidelity of molecular generation. A recent international collaboration demonstrated a prototype quantum-powered generative adversarial network for drug discovery, combining quantum computing with AI to propose novel chemical structures. The quantum component can handle the combinatorial complexity of molecule generation more efficiently, offering a richer variety of candidate compounds for medicinal chemists to explore. InSilico Medicine, for instance, has been experimenting with quantum GANs in generative chemistry, aiming to leverage quantum sampling to navigate chemical space more thoroughly than classical AI alone. The upshot is quantum-enhanced AI could design better drug leads or biological molecules (like optimized antibodies or peptides) in a fraction of the time, by evaluating many possibilities simultaneously and understanding molecular patterns on a quantum level.
Additionally, quantum machine learning could find complex patterns in biomedical data that classical ML struggles with. Healthcare datasets – think genomic sequences, proteomic data, electronic health records – are not only enormous, but have intricate, non-linear relationships. QML algorithms (for example, quantum support vector machines or quantum neural networks) might detect patient clusters or treatment outcome predictors with higher accuracy. There’s also interest in quantum-enhanced natural language processing to sift through vast biomedical literature or patient records to find relevant insights faster. Companies like Google have hinted at using their quantum processors to assist in analyzing genetic variations linked to disease and in modeling biological systems for research. Even DeepMind’s AlphaFold, which revolutionized protein folding via AI, is now conceptually being linked with quantum computing research – exploring whether quantum computers can further improve protein structure predictions and dynamics simulations. While much of quantum-enhanced AI for healthcare is still in the research stage, the convergence of these fields is accelerating. The goal is that in coming years, many AI-powered biotech tools – from diagnostic algorithms to drug discovery platforms – will have quantum accelerators under the hood, enabling them to solve biomedical problems faster and more comprehensively than ever before.
Optimization of Clinical Trials and Supply Chain Logistics
Beyond discovery and diagnosis, quantum computing can also tackle operational challenges in pharma and biotech, such as clinical trial optimization and supply chain management. Designing a clinical trial involves a daunting optimization problem: selecting patient cohorts across sites, determining dosage schedules, minimizing biases, and ensuring statistical power, all under various constraints. Classical methods rely on heuristics or simplified models, meaning trial designs may not be truly optimal. Quantum computers excel at certain optimization tasks (thanks to algorithms like QAOA or quantum annealing), and this is being applied to improve clinical trial efficiency. In fact, some partnerships have already piloted this – for example, Zapata Computing worked with pharmaceutical firms to use quantum-inspired algorithms for optimizing clinical trial design. By encoding trial parameters into a quantum algorithm, they aimed to find the best configuration out of an astronomically large solution space (something a brute-force classical approach could never attempt). A quantum-enhanced approach could balance numerous factors (patient diversity, drop-out rates, treatment vs. placebo ratios, etc.) to suggest trial designs that yield conclusive results faster or with fewer patients. This could reduce the costly failures or mid-trial adjustments that sometimes plague drug development. While these methods are still experimental, early results hint that even noisy quantum devices can provide useful guidance for complex trial planning. In the future, a pharmaceutical company might routinely use a quantum computer to simulate thousands of trial variations and pick the optimal one, potentially shaving months off the clinical development process.
On the manufacturing and supply chain side, pharma companies face highly complex logistics – from sourcing raw materials and managing production lines (for small-molecule drugs or biologics) to distributing finished products globally under tight regulations (and often cold-chain requirements). These processes involve many variables and constraints, forming massive optimization puzzles that quantum computing is well-suited to address. Microsoft, for instance, has highlighted how its Azure Quantum platform helps biotech firms streamline manufacturing and supply chains: using quantum algorithms to optimize everything from raw material sourcing to scheduling production runs. By crunching countless possible production sequences or shipping routes in parallel, a quantum solver can identify solutions that cut costs and time – for example, optimal allocation of limited manufacturing capacity to different drugs, or the fastest way to deliver a therapy to dozens of clinical sites around the world. Early adopters have used quantum-inspired solutions to improve the scale-up of biologics (like monoclonal antibody production), finding more efficient processes that would be hard to discover manually. Logistics giants are similarly exploring quantum algorithms to optimize delivery routing and supply distribution, which is directly applicable to distributing vaccines or medicines to clinics. As an illustration, consider distributing a new vaccine during a pandemic: there are myriad possible ways to route shipments to thousands of clinics under refrigeration constraints. A quantum computer could evaluate far more of these routing combinations than a classical computer, potentially identifying a distribution plan that reaches people faster or uses fewer resources.
In current practice, companies like IBM and Amazon (with AWS Braket) are enabling pharma supply chain experiments on their quantum cloud platforms. And industry surveys already list manufacturing & supply optimization among the expected use cases for quantum in life sciences. The impact of this optimization could be significant: more robust supply chains mean fewer drug shortages, and more efficient manufacturing could lower drug costs. When paired with AI (for predictive maintenance of equipment or demand forecasting), quantum optimization can further enhance reliability. It’s worth noting that many of these optimization problems can often be tackled with quantum-inspired algorithms on classical hardware as an intermediate step – and indeed, some solutions being deployed are quantum-inspired rather than strictly running on a quantum computer. Even so, they draw on quantum principles to find better solutions. As quantum hardware matures, direct quantum optimization of pharma logistics will become more practical. The net result will be an industry that not only discovers drugs faster with quantum computing, but also gets those drugs to patients more swiftly and efficiently by optimizing the “last mile” of development and delivery.
Quantum Sensing for Bioimaging and Diagnostics
Not all quantum breakthroughs in healthcare come from computing; quantum sensing is another pillar of quantum technology with immediate biomedical applications. Quantum sensors exploit quantum phenomena to measure physical quantities with extreme sensitivity and precision. In medicine, this translates to imaging and diagnostic techniques capable of detecting subtle signals that conventional sensors miss. In fact, the most mature quantum technologies today are sensors – MRI machines and PET scanners, for example, rely on quantum physics principles (nuclear spin resonance and positron annihilation, respectively) and have been staples of diagnostics for decades. The next generation of quantum sensors promises to push the boundaries of what we can see and measure in biological systems. For instance, optically pumped magnetometers (OPMs) and diamond NV-center sensors can detect extremely faint magnetic fields, such as those produced by neuronal electrical activity in the brain or by the heart’s electrophysiology. This could enable magnetoencephalography (MEG) brain imaging without the bulky superconducting apparatus, or fetal heart monitoring from outside the womb with much higher sensitivity than current technology. Quantum sensors’ increased sensitivity and potential for non-invasive, high-resolution measurement open up a range of biomedical uses: from sub-cellular imaging (e.g. detecting the magnetic signatures of individual bacteria or molecules), to real-time metabolic monitoring (sensing minute changes in blood chemistry), to early disease detection through ultra-precise biomarker measurements.
One exciting application is in diagnostics and imaging for cancer and other diseases. Quantum sensors could improve the detection of tumors or diseased tissue by picking up tiny differences in magnetic or electric properties. For example, certain quantum-enhanced imaging techniques might distinguish cancerous cells by their slight magnetic field distortions or by using entangled photons to get super-resolution images of tissues. According to a recent industry consortium report, quantum sensors could enable significantly more efficient and accurate medical diagnoses, thanks to their heightened sensitivity. They could collect vast amounts of patient data (like scanning the body for trace molecular signals of disease) and catch disease at an earlier stage than conventional diagnostics allow. Areas like brain imaging stand to benefit: with quantum sensors, one could conceivably image neural activity or neurotransmitter levels with resolution and depth that today’s fMRI or EEG can’t achieve. Even outside the hospital, quantum sensing might enhance wearable health monitors – for instance, wearable quantum devices measuring blood oxygen or sugar levels with lab precision continuously. Researchers are already working on some of these fronts: the NIH has funded projects to use quantum sensors for imaging inside living cells and for noninvasive monitoring of physiological parameters.
Another domain is bioimaging at the microscopic scale. Quantum fluorescence microscopes and nanodiamond sensors can potentially observe biochemical reactions in real time at the single-molecule level, which would be revolutionary for cell biology and drug development. Imagine watching how a drug molecule binds to a target protein inside a living cell via a quantum sensor tag – providing instant feedback on drug mechanism. Quantum sensing might also improve diagnostic scans like MRI: for example, using entangled particles to enhance image clarity or quantum algorithms to reconstruct images with less data (which could reduce scan times or radiation doses). The UK’s Quantum Technology Hub in Sensors and Timing and similar programs are actively looking at medical imaging use cases. In one international effort, the U.S. Quantum Economic Development Consortium has a project to develop ultra-sensitive quantum sensors for early disease detection, explicitly aiming to advance medical imaging capabilities. The bottom line is that quantum sensing could provide doctors with new eyes and ears – devices that see the otherwise invisible and diagnose the otherwise undetectable. While some quantum sensor technologies are still experimental, others (like improved MRI magnetometers) are likely to reach clinics in the coming years. They will complement quantum computing’s impact by improving how we gather data from patients, which in turn feeds into better analyses and treatments.
The Arrival of Universal Quantum Computing
So far, much of the quantum computing work in pharma/biotech has used NISQ (noisy intermediate-scale quantum) devices – early machines that have dozens or a few hundred qubits but are not error-corrected and still prone to noise. These devices can perform certain tasks but with limited fidelity. The true paradigm shift is expected with the arrival of universal, fault-tolerant quantum computers – machines with error-corrected qubits that can run long and complex computations reliably. Industry roadmaps suggest that fully error-corrected quantum computers could be realized beyond 2030, ushering in an era where quantum computing’s full power is available for practical use. When that happens, the impact on pharma and biotech will be immense. A universal quantum computer could simulate larger, more complex molecules and biological systems in exact detail, something currently impossible. This means drugs involving macromolecules (like proteins, antibodies, RNA therapeutics) – which today we largely have to discover via lab experiments – might be rationally designed entirely via computation. We could virtually screen millions or billions of compounds against not just one target, but networks of targets and pathways, to find optimal therapeutic interventions for diseases like Alzheimer’s or cancer, which involve many proteins and genes. In fact, researchers have suggested that fault-tolerant quantum computers could uncover novel therapeutic targets and strategies for complex diseases by simulating biochemical interactions at a scale and accuracy that is completely out of reach today. The result could be breakthrough treatments for conditions that have eluded cures so far.
The beneficial effects of large-scale quantum computing on the pharma/biotech sector will span R&D and beyond. For drug discovery, it would drastically cut the cost and time needed to bring new drugs to market – some estimates say cost reductions up to 40% for early research and trimming several years off development. This could make developing treatments for rare diseases or globally neglected diseases economically feasible, since quantum computing would lower R&D expenditures. Patients would see novel drugs (and maybe even personalized drug formulas) arriving much faster. In clinical medicine, universal quantum computers might enable real-time analysis of patient data and simulations of disease progression to guide treatment decisions on the fly. Healthcare could become more proactive and predictive, with quantum computers continuously analyzing health data streams to flag risks and suggest interventions specific to each individual. On the biotech side, areas like synthetic biology and bioengineering would flourish – one could design complex biomolecules or even cells with desired functions using quantum-precise modeling, advancing fields like gene therapy, vaccine development, or enzyme engineering. The upshot is a potential golden age of pharmaceutical innovation: with quantum computing, more shots on goal in research and far fewer expensive failures, resulting in a broader range of therapies for patients worldwide.
However, the arrival of powerful quantum computers will also have disruptive effects that the industry must brace for. One oft-cited concern is data security: fault-tolerant quantum machines will eventually be able to crack current encryption schemes (like RSA) by brute-forcing large integer factorizations via Shor’s algorithm. This means that sensitive pharmaceutical data – from proprietary drug formulas to patient records – could be vulnerable if companies don’t transition to quantum-safe encryption in time. The pharma industry handles huge amounts of confidential information and trade secrets; a breach enabled by quantum decryption would be devastating. Thus, even as they chase the benefits of quantum computing, organizations must invest in post-quantum cryptography to secure their data (we discuss this further in Challenges below). Another disruptive aspect is competitive balance. Quantum computing capabilities might not arrive everywhere equally: there’s a risk of a quantum divide where companies or countries with early access to universal quantum computers leap ahead in drug discovery, while others fall behind. A pharmaceutical firm that’s an early adopter could potentially design superior drugs at breakneck speed, squeezing out competitors who rely on slower classical R&D. This raises strategic questions – e.g., should smaller biotechs partner with tech providers or consortia to gain quantum access so they aren’t left out? We might also see non-traditional players (big tech, AI-driven startups) enter the pharma space leveraging quantum advantage, disrupting the traditional pharma model.
Furthermore, once fully capable quantum computers are online, some existing processes will be upended. The role of high-performance classical computing in pharma may diminish as quantum takes over certain tasks. Skill sets will shift (quantum algorithm specialists could become as important as medicinal chemists in some R&D teams). And regulatory frameworks will need to adapt quickly – for instance, how will agencies like the FDA evaluate a drug candidate that was discovered and largely validated by quantum simulations? They may need new guidelines to trust computational evidence of safety/efficacy produced by methods unlike those used historically. On the positive side, by the time we have universal quantum computers, we will also likely have quantum-informed AI that can make sense of the deluge of biological data, leading to a more integrated approach to healthcare (imagine AI that can reason about biology with the aid of quantum-computed insights). All told, the fault-tolerant quantum era, expected in the next decade or two, is poised to both greatly benefit and sharply disrupt the pharmaceutical and biotech sector. Companies that prepare now for the quantum leap will be positioned to capture its value – those that remain complacent could find themselves overtaken in the race to discover and deliver the medicines of the future.
Sector Preparation & Responses
Recognizing both the promise and the challenges of quantum computing, stakeholders across the pharmaceutical and biotechnology industries are actively preparing for this technological shift. Leading pharma companies have been among the first to invest in quantum research and partnerships. As mentioned, firms like Merck, Roche, Pfizer, Johnson & Johnson, and others have not only filed patents in the quantum space but also formed collaborations with quantum technology companies. For example, Merck is involved in multiple initiatives (from its work with QC Ware on quantum chemistry algorithms to the SEEQC consortium building a quantum computer for pharma use). Boehringer Ingelheim’s partnership with Google Quantum AI, launched in 2021, explicitly focuses on developing quantum use cases for drug discovery and design. Similarly, Biogen and Bayer have engaged quantum computing startups to explore drug modeling problems. These partnerships allow pharma scientists to start getting hands-on experience with quantum algorithms and help quantum experts understand real pharma needs – a win-win that builds readiness on both sides.
Many companies are also creating internal quantum R&D teams or centers. For instance, biotech company Amgen reportedly established a small quantum computing task force to evaluate how the tech could integrate into its computational chemistry workflows. Some firms have a dedicated “Head of Quantum Computing” role now, indicating a strategic commitment. Johnson & Johnson, AstraZeneca, and GlaxoSmithKline have had scientists publish research on quantum applications, showing internal support. Furthermore, consortia like QuPharm (which counts numerous big pharma members) provide a forum to share knowledge and even pool resources for pre-competitive quantum tool development. By collaborating through such alliances, companies can avoid duplication of effort on foundational quantum techniques (like quantum algorithms for molecular energy calculations) and collectively push the field forward. The Pistoia Alliance’s future-casting of quantum computing and the resulting identification of 20+ high-value pharma use cases by 2020 was one example of industry-wide strategic planning. This has given companies a roadmap of where quantum could impact their business, so they can plan pilot projects accordingly.
Investment in workforce development is another critical response. Pharma companies acknowledge that quantum computing expertise is in short supply and highly specialized. To address this, some are upskilling their existing computational scientists and chemists with quantum training. We’re seeing early steps like sponsoring employees to take quantum computing courses or workshops, and inviting quantum computing vendors to run training sessions. Specialized quantum-computing-for-chemistry courses are popping up in academia, often supported by industry. Notably, startups like Zapata Computing have started offering training programs to bridge the knowledge gap between quantum physics and drug discovery for pharmaceutical companies. This kind of capacity-building ensures that when the technology is ready, there will be people able to implement it. Additionally, recruiting is ramping up: companies are hiring quantum chemists, physicists, or software developers straight from grad schools or tech firms. Boehringer Ingelheim, for example, brought on quantum computing scientists to join their research staff in recent years. Joint appointments or fellowships between pharma companies and academic quantum labs are also emerging, which help transfer knowledge in both directions.
On the infrastructure and strategy side, many pharma/biotech firms are making modest but meaningful investments in quantum hardware and cloud access. Rather than building quantum computers (which is outside their core business), they are securing access to quantum computing resources. This might be through cloud services (IBM’s Quantum Network has dozens of biotech and research members) or through consortia that provide shared hardware access. For example, multiple pharma companies have joined IBM’s Quantum Accelerator program, which grants prioritized access to IBM’s latest quantum processors and technical support to experiment with real-life problems. Such early access programs are invaluable for testing algorithms on actual quantum machines and learning their quirks. Companies are also budgeting for proof-of-concept projects – essentially R&D prototypes where a small quantum algorithm is applied to a problem like molecular energy calculation, to assess performance relative to classical methods. “Start small and test” is the prevailing strategy: identify a pain point in the R&D pipeline that might benefit from quantum acceleration, partner with quantum experts to attempt a solution, and measure the results. These experiments, even if they don’t yet outperform classical computing, teach organizations how to work with quantum tech and what bottlenecks need addressing.
From a regulatory and policy perspective, the sector is also proactively engaging. Pharma and biotech companies operate in a heavily regulated environment, so they are beginning to dialog with regulators about quantum computing. In the EU, for instance, industry groups are informing the European Medicines Agency and other bodies about the coming changes – the recent EU declaration on quantum technologies emphasizes making Europe a “quantum valley” and highlights life sciences as a key area. Regulatory considerations include how to validate quantum-derived results (ensuring that drug candidates predicted by quantum models are reliable) and how to manage patient data security in a quantum era. Companies are working on quantum-safe encryption now to protect clinical trial data and health records, often following government guidelines. Some are taking cues from other sectors: for example, seeing banks like JPMorgan test quantum key distribution for secure communication, a few healthcare enterprises have begun similar trials for health data encryption. Additionally, pharma companies part of standards bodies or advisory boards are pushing for guidelines on quantum computing use – to ensure, for example, that if quantum algorithms are used in medical devices or diagnostic software, they meet safety and efficacy standards just like any other medical tech.
Finally, we see significant public sector support and academia-industry collaboration gearing up the ecosystem. The U.S. National Quantum Initiative Act is funneling federal funding into quantum research centers, some of which focus on health and bio-applications. The NIH and NSF have issued grants for quantum sensing in biomedicine and quantum computing for health data, reflecting government interest in keeping the country at the forefront. In the UK and Germany, government-backed programs (Innovate UK grants for quantum pharma, the German QUTAC consortium uniting pharma and tech companies) are ensuring companies have a sandbox to develop quantum solutions with less financial risk. Universities, often with pharma funding, are creating interdisciplinary labs – e.g. a university might have a quantum computing unit embedded within a medical research institute. Oxford’s participation in the Merck/SEEQC quantum computer project exemplifies academia stepping in to drive quantum drug discovery innovations alongside industry and government. All these moves point to a sector that is not sitting idle. The overwhelming sentiment is that quantum computing is coming, and preparation today is essential. A McKinsey analysis urged pharma executives to start developing a strategic quantum roadmap now, assessing their R&D portfolios for quantum opportunities and building internal know-how ahead of the technology’s full maturation. By investing early – in collaborations, talent, and pilot projects – pharmaceutical and biotech companies aim to be “quantum-ready” and turn this disruptive technology into a competitive advantage rather than a threat.
Challenges and Risks
For all its potential, quantum computing in pharma/biotech faces significant challenges and risks that must be navigated:
Technical Hurdles: Quantum hardware today is extremely delicate. Qubits suffer from decoherence (losing their quantum state) within fractions of a second, and quantum computations are riddled with errors unless corrected. The current limitation in qubit coherence time restricts the size and complexity of molecular simulations one can run reliably. Scaling up to millions of qubits with full error correction is a major engineering challenge still likely a decade away. Until then, most quantum computations will be “noisy,” requiring hybrid algorithms to get useful results (e.g. the Variational Quantum Eigensolver, which pairs quantum circuits with classical optimization to mitigate noise). Additionally, many quantum algorithms need deeper circuits than hardware currently allows, making some theoretical advantages unreachable in the near term. There’s also a scarcity of key components (like certain superconducting materials or high-quality laser systems for ion trap qubits) and supply chain limitations, which keep quantum computers costly and not widely accessible. In quantum sensing, similar reliability and cost issues exist – some sensors need ultra-low temperatures or are expensive prototypes. These technical constraints mean that in the short run, expectations must be tempered: quantum tools might only outperform classical methods in niche sub-problems or require significant classical post-processing. The risk is overhyping the tech before it truly delivers, which could lead to disillusionment or reduced investment if early returns don’t meet expectations.
Workforce and Knowledge Gap: Successfully applying quantum computing to biology requires an intersection of expertise that is rare – quantum physicists who understand biochemistry, or computational biologists fluent in quantum algorithms. Currently, there is a small workforce of people with this interdisciplinary know-how. Pharma IT teams and chemists largely lack quantum training, and conversely, many quantum computing experts are unfamiliar with the nuances of drug discovery or genomics. This gap could slow adoption as organizations struggle to find or train the right talent. As noted, initiatives are underway to train scientists in quantum drug discovery, but scaling that up is a challenge. If companies do not invest in workforce development, they may have the tech available but not the internal capability to use it effectively. Moreover, the novelty of the field means there aren’t established best practices – early teams are learning via trial and error how to formulate their problems in quantum terms. Academic curricula are only just beginning to integrate quantum computing into life sciences programs. Until a critical mass of practitioners is achieved, this human factor will be a bottleneck.
Data Security and Privacy: The flip side of quantum computing’s power is its ability to break current encryption protocols. This looms as a serious risk for any industry dealing with sensitive data, including healthcare and pharma. Confidential clinical trial data, patient health records, and intellectual property (patents, drug formulas) all rely on encryption for security. If a malicious actor obtains a large-scale quantum computer (or if one becomes available to adversarial nation-states), they could potentially decrypt sensitive biomedical data that was encrypted with RSA, ECC, or other traditional schemes. This threat is not theoretical – experts project that in the next decade or so, quantum attacks on classical encryption will become feasible. The healthcare sector must therefore upgrade to quantum-safe encryption algorithms (like lattice-based, hash-based, or QKD systems) to safeguard data. This transition is non-trivial and requires forward-planning (e.g. ensuring today’s encrypted archives won’t be easily decrypted in a decade by harvesting attacks). There’s also the privacy aspect: quantum computing might enable analyzing combined datasets (say genomic + insurance + IoT health data) in ways that could inadvertently expose personal health information if not properly protected. While quantum computing itself can be used to enhance data security (quantum cryptography offers virtually unbreakable communication channels), the interim period where not everyone is protected is a risk. Regulators are urging “harvest now, decrypt later” awareness – meaning even if data is safe today, it could be stolen now and decrypted in the future when quantum capability is available. Pharmaceutical firms and hospitals need to be proactive in addressing this, or they could face breaches of patient trust and massive liability.
Regulatory and Validation Challenges: The integration of quantum-generated insights into healthcare raises questions for regulators. Agencies like the FDA (Food and Drug Administration) or EMA (European Medicines Agency) have established protocols for approving new drugs or medical devices, which typically involve interpreting results from classical statistical analyses and deterministic simulations. Quantum computing introduces probabilistic algorithms and outputs that may be harder to interpret in traditional ways. How do you validate that a quantum-derived drug candidate is genuinely promising? Regulators might require additional evidence or new types of analysis, which could slow down approval if not anticipated. Moreover, the lack of standards or guidelines for quantum software in healthcare is a gap – for instance, if a company uses a quantum algorithm to prioritize clinical trial endpoints, how should that process be audited? There’s a risk that regulators, moving cautiously, could become a bottleneck for quantum-enabled products or decisions until they update their frameworks. On the flip side, without guidance, companies might hesitate to act on quantum outputs in critical decisions (like skipping certain trial phases based on quantum predictions) for fear of regulatory pushback. Close communication between industry and regulators is needed to develop appropriate standards. Ethical guidelines will also be needed if quantum algorithms are used in patient-facing decisions – ensuring transparency and fairness, so that, for example, a quantum AI system diagnosing patients doesn’t inadvertently introduce bias or violate informed consent norms. These regulatory hurdles are surmountable, but they require time and education of policy-makers, meaning a risk of delays in quantum tech deployment for healthcare impact.
Barriers to Adoption (Costs and Integration): Even once quantum computing solutions become available, pharma and biotech firms may encounter practical barriers to adopting them. Cost is one issue: currently, accessing a quantum computer can be expensive, and scaling up usage might incur significant cloud computing fees or investments in on-premise quantum infrastructure (for those few who might consider it in the future). Smaller biotech startups might find cost a limiting factor, potentially widening the gap between cash-rich pharma giants and resource-strapped innovators. Over time costs are expected to come down, but in the near term this could slow democratization of the tech. Another barrier is integration with existing IT systems and workflows. Companies have massive investments in classical computing pipelines, software tools, and data infrastructure. Introducing quantum algorithms means developing new software that often has to work in tandem with classical systems (hybrid workflows). The complexity of retrofitting or complementing established pipelines with quantum components – and training IT staff to maintain these – shouldn’t be underestimated. There may be resistance to change, or simply the inertia of large organizations, which could lead to slow adoption even when quantum methods are available. Additionally, the benefit of quantum computing will vary by problem – some tasks might see only incremental improvement, not justifying the switch. Identifying the “low-hanging fruit” where quantum clearly wins is itself a challenge. Until strong success stories and ROI cases emerge, some decision-makers will remain skeptical (especially given the history of hype cycles). Finally, the field of quantum algorithms is still evolving; there’s a risk for early adopters that they invest in a particular quantum approach which becomes obsolete or surpassed by a better algorithm or hardware paradigm (for example, betting on quantum annealing vs. gate-model computing). This uncertainty can make companies hesitant to commit fully.
Despite these challenges, the overall trajectory remains positive – each risk is being actively addressed through research and policy. Technical issues are being tackled by vigorous development of better qubits and error-correction techniques. The workforce gap is starting to close as training programs and interdisciplinary collaborations grow. On security, there’s a push for widespread adoption of quantum-resistant encryption (with NIST, for instance, standardizing post-quantum cryptographic algorithms to replace vulnerable ones). Regulators are engaging with the quantum topic more now than a few years ago, and likely to release guidance as the tech comes closer to practical use. Knowing these hurdles ahead of time allows the industry to plan mitigations. Patience and realism will be key: quantum computing for pharma is not a magic bullet arriving overnight, but a powerful tool that will incrementally integrate into the landscape. By acknowledging the risks – technical, human, and organizational – stakeholders can ensure that when quantum solutions do arrive at scale, they are ready to be adopted safely, securely, and effectively in service of better health outcomes.
Conclusion
Quantum computing is poised to become a catalytic force in the global pharmaceuticals and biotechnology industries. Its ability to tackle problems of staggering complexity – whether simulating the quantum behavior of drug molecules, analyzing massive genomic datasets for personalized medicine, or optimizing the myriad decisions in R&D and supply chains – offers a new computational paradigm for an innovation-hungry sector. We have seen that even in its nascent state, quantum technology is already making waves: early experiments have accelerated molecular discovery, quantum sensors are breaking new ground in biomedical imaging, and companies big and small are gearing up through partnerships and pilot projects to be part of this coming revolution. The key applications range from drug discovery (with quantum simulations promising faster and more accurate identification of drug candidates) to patient-centric care (with quantum algorithms potentially enabling treatment plans tailored to one’s DNA). And as fault-tolerant quantum computers emerge in the next decade or so, these capabilities will only multiply – potentially shaving years and billions off the drug development process and unlocking therapies for diseases long deemed “undruggable.”
Yet, a clear-eyed view acknowledges that challenges remain on the road to this quantum-enabled future. Technical limitations, a need for skilled talent, and considerations of security and regulation mean the industry must tread thoughtfully even as it leaps forward. The encouraging news is that the pharma/biotech sector, often criticized in the past for conservatism, is largely leaning in to quantum computing rather than shying away. Companies are investing, learning, and collaborating at unprecedented levels to overcome these hurdles – effectively preparing the field for quantum’s transformative entrance. In labs and boardrooms today, strategies are being crafted not just to incorporate quantum tools, but to evolve organizational mindsets for the quantum era. This proactive stance bodes well for a smoother integration of quantum technologies when they mature.