New Hybrid Quantum Monte Carlo Algorithm
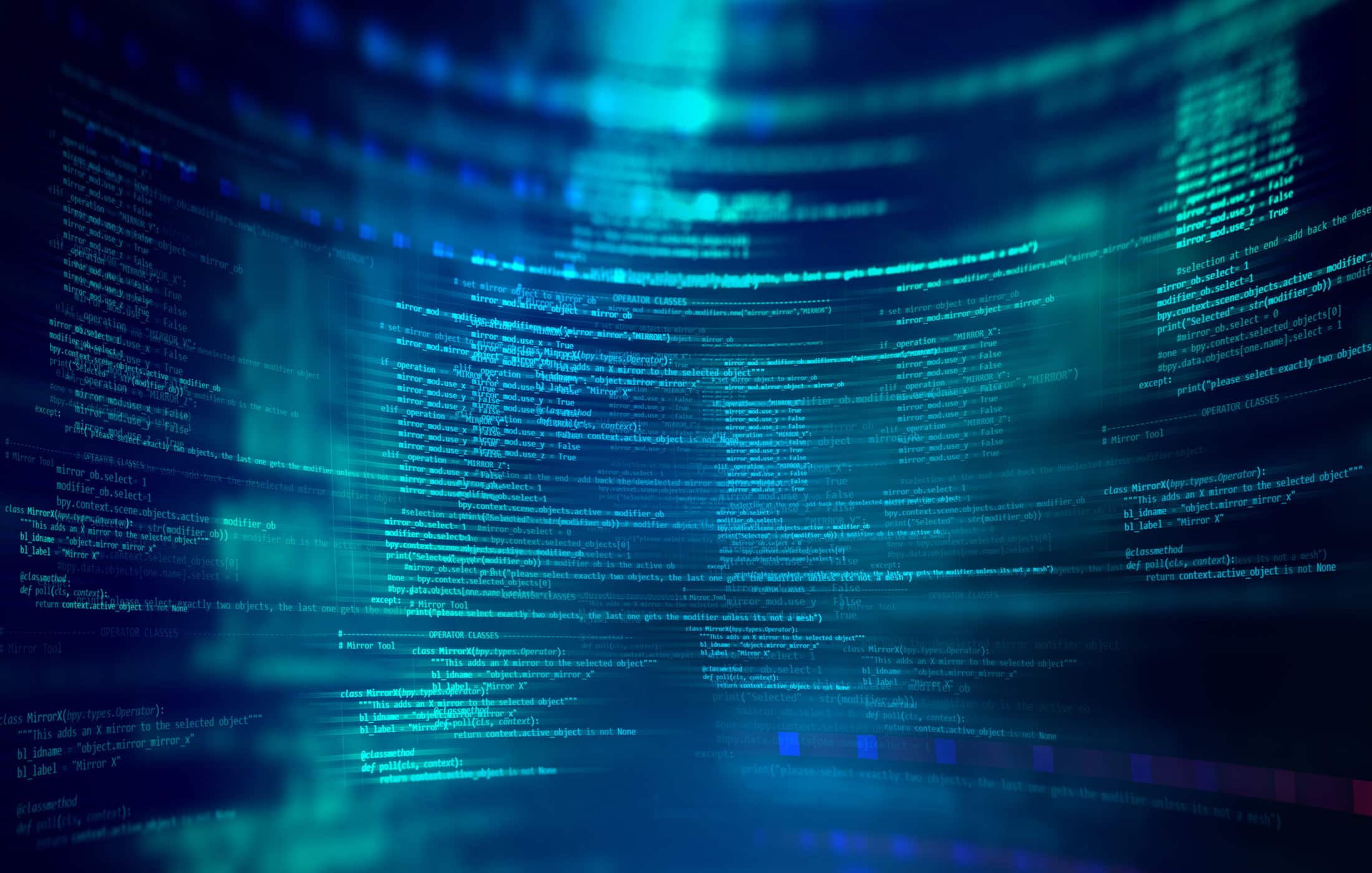
Table of Contents
Beijing, August 2023 – A team of physicists has unveiled a new quantum-classical hybrid algorithm that promises to overcome one of the most vexing hurdles in simulating quantum many-body systems. Researchers Xiaosi Xu and Ying Li have developed a “quantum-assisted” Monte Carlo method that uses a small quantum processor to boost the accuracy of classical simulations. The breakthrough, published in Quantum in 2023, addresses the notorious sign problem in quantum Monte Carlo calculations – a key issue that causes explosive uncertainty in simulations of electrons and other fermions. By incorporating quantum data into the Monte Carlo sampling process, the new algorithm sharply reduces the bias and error that plague fully classical methods, potentially enabling more precise predictions of molecular energies and material properties on today’s imperfect quantum hardware. Experts say this development could accelerate progress toward practical quantum advantage in fields ranging from chemistry to materials science.
Expert Commentary
Quantum computing experts are hailing the hybrid approach as an important milestone on the road to useful quantum algorithms. William Huggins of Google Quantum AI, who helped pioneer early quantum-classical Monte Carlo techniques, noted that combining small quantum computations with classical Monte Carlo “offers an alternative path towards achieving a practical quantum advantage for the electronic structure problem” – without requiring extremely accurate quantum hardware or error-corrected qubits. In 2022, Huggins and colleagues demonstrated the power of this approach by using a 16-qubit quantum processor to guide a classical simulation of a chemical system with 120 orbitals. Remarkably, their hybrid algorithm achieved chemical accuracy on this problem, rivaling state-of-the-art classical methods “without burdensome error mitigation.” This showed that even today’s noisy quantum chips can help solve meaningful chemistry problems when paired with smart classical algorithms. “By using a quantum computer, we hope to improve the initial guess that guides our calculation and obtain a more accurate answer,” Huggins explained, referring to how quantum data can steer Monte Carlo simulations toward the correct result.
Other experts echo the optimism. Researchers at Amazon Web Services noted that quantum-assisted Monte Carlo techniques can reduce bias and statistical variance in energy estimates, albeit at the cost of introducing some measurement noise from the quantum hardware. This trade-off is considered favorable if it means more reliable simulations of complex molecules. “The fact that these [hybrid] results are so close to ideal values tells us that the error in our calculation comes from using an approximate ground state on the quantum computer that was too simple, not from being overwhelmed by noise” one researcher observed after large-scale tests. In other words, the limiting factor was the simplicity of the quantum trial wavefunction, not the quantum hardware errors – a promising sign for future improvements. Overall, the quantum computing community sees Xu and Li’s 2023 advance as validation that hybrid algorithms can deliver useful accuracy before fully fault-tolerant quantum computers arrive. “We know the road ahead of us is long, but we’re excited to have another tool in our growing toolbox,” Huggins wrote, reflecting a broader sentiment that such innovations are key steps toward quantum-enabled breakthroughs.
Technical Explanation
The Sign Problem in Quantum Monte Carlo
Classical quantum Monte Carlo (QMC) methods use random sampling to estimate the properties of a quantum system’s ground state. They have been hugely successful for many problems, but they hit a wall for large systems of fermions (particles like electrons) due to the infamous sign problem. In essence, whenever identical fermions exchange places, the quantum-mechanical wavefunction acquires a minus sign. In Monte Carlo simulations, this leads to cancellations between positive and negative contributions. As the system size grows, these cancellations cause the simulation’s statistical variance to blow up exponentially, making the results hopelessly noisy. To keep computations feasible, classical QMC algorithms usually impose a workaround – for example, the fixed-node or constrained path approximation – which forces the simulation to avoid regions where destructive cancellation occurs. While this tames the sign problem, it unfortunately introduces bias: the algorithm is no longer exact, and its accuracy now depends on the quality of the guess used to constrain the signs. In practical terms, one must supply a good approximate wavefunction (a trial state) to guide the Monte Carlo sampling, or else the results deteriorate. Even with a guide, there is always some residual error because the classical computer can’t perfectly represent the true quantum state. This trade-off has been a longstanding hurdle in simulating quantum chemistry and condensed matter systems.
Quantum-Assisted Monte Carlo and Bias Reduction
The new hybrid algorithm by Xu and Li attacks this problem by enlisting a quantum computer to provide a better guide for the Monte Carlo simulation. Instead of relying solely on a heuristic trial wavefunction from a classical method, their approach uses a quantum processor unit (QPU) to prepare and sample a trial state that captures more of the true quantum correlations.
In practice, the quantum device is used to efficiently compute key quantities that are very costly to obtain classically – for example, the overlaps between the trial state and the Monte Carlo “walker” states, and the local energy of those walkers. These overlap and energy values are fed into the classical Monte Carlo update steps, so that the random walk is biased toward the true ground state but in a way informed by quantum-mechanical accuracy. Essentially, the quantum computer serves as a shortcut to evaluate how “good” a given configuration is (by comparing it to a quantum-prepared state), something that would otherwise require exponentially hard computations on a classical machine. This extra guidance dramatically reduces the systematic error. “The quantum trial state provides a better approximation to the ground state… thus reducing the bias and variance in QMC calculations,” as an AWS quantum research blog explains. By using the QPU to filter out the Monte Carlo samples that would have caused sign cancellations (i.e. the problematic negative weight contributions), the hybrid algorithm effectively mitigates the sign problem without freezing the simulation into a biased approximation.
Compared to a purely classical QMC, the result is a closer convergence to the true ground-state energy. In Xu and Li’s paper, they describe a framework that allows the quantum assistance to be dialed up or down flexibly. They present two strategies based on the extent of quantum resources used: even a minimal quantum helping hand yields a noticeable improvement over classical results, and increasing the quantum involvement (toward a full quantum amplitude estimation of the energy) leads to further bias reduction. In simulated benchmarks on fermion models, both strategies produced ground-state energy estimates that were significantly more accurate (lower in energy) than the standard constrained QMC result, closing much of the gap to the exact answer.
Bayesian Inference to Reduce Quantum Measurements
One innovation in Xu and Li’s 2023 algorithm is the use of Bayesian inference to dramatically cut down the number of quantum measurements required. In any hybrid scheme, the quantum processor will be invoked repeatedly to gather data (for instance, overlap values or estimator amplitudes) that feed into the Monte Carlo. Naively, to get high-precision results, one might have to perform many runs on the quantum hardware and average the results, since each quantum measurement has statistical noise. This could become a bottleneck if millions of shots are needed. Xu and Li address this by adopting a Bayesian approach: instead of taking a simple empirical average of quantum measurements, they continually update a probability distribution (a Bayesian posterior) for the quantity of interest, refining their estimate with each new piece of data. In effect, the algorithm “learns” the value of the bias-correcting term more efficiently than brute-force sampling.
The authors report that this Bayesian amplitude estimation strategy achieves the desired bias reduction with much fewer quantum samples than traditional methods. Importantly, they find that they can maintain a stable quantum advantage – meaning the quantum-assisted algorithm stays more accurate than the classical one – even as they reduce the number of quantum measurements. This makes the hybrid approach more practical on real hardware, where run-time (and cost) are constrained. By intelligently leveraging prior knowledge and updating credences, the Bayesian inference step minimizes redundant queries to the QPU. It’s a bit like using statistical “sense” to get more bang for the buck from each quantum experiment, ensuring the quantum boost comes at minimal overhead.
Quantum Resource Requirements
A key appeal of this hybrid Monte Carlo method is its frugal use of quantum resources. The quantum computer serves as a co-processor for specific tasks and need not simulate the entire many-body system on its own. In fact, Xu and Li designed the algorithm such that the quantum computer is used at its minimal cost and still can reduce the bias. Concretely, this means only a relatively small number of qubits and gate operations are required to gain the quantum advantage. For example, in the earlier Google experiment, the team was able to unbias a simulation of a 120-orbital system using just a 16-qubit quantum circuit as the trial state generator. This is orders of magnitude fewer qubits than would be needed to represent the full 120 orbitals on a quantum computer directly.
The new Xu–Li algorithm follows a similar philosophy: let the classical computer handle the heavy lifting of sampling a large configuration space, and enlist the quantum device sparingly for the pieces it can do exponentially better (like evaluating overlaps in a large Hilbert space). This separation of duties means the algorithm can run on near-term quantum hardware. The required quantum circuits involve preparing approximate ground-state wavefunctions and measuring overlaps or probabilities – tasks that can often be done with shallow circuits or phase estimation routines on tens of qubits. Notably, the algorithm avoids complicated controlled operations that some quantum algorithms need.
In one related hybrid Monte Carlo scheme, researchers highlighted that their approach “does not require controlled real-time evolution, thus making its implementation much more experimental-friendly.” Likewise, because the Monte Carlo algorithm is non-variational (it doesn’t rely on tuning a large number of quantum circuit parameters via an optimizer), it sidesteps the dreaded “barren plateau” issue that can afflict variational quantum algorithms.
In summary, the quantum-classical Monte Carlo method strikes a balance between simplicity and power: it demands only moderate quantum resources – well within the reach of current or imminent devices – while still delivering a statistically significant improvement over purely classical computation. Xu and Li emphasize that their framework is scalable in the sense that one can incrementally increase the quantum resources (more qubits, deeper circuits, more samples) to systematically improve accuracy. This opens the door to a family of implementations suitable for different hardware budgets, all built on the same core principle of quantum-assisted sampling.
Comparison with Other Hybrid Approaches
The idea of blending quantum computing with classical Monte Carlo is gaining momentum, and Xu and Li’s work builds on several earlier attempts — while also introducing important innovations. The first notable demonstration of a hybrid quantum Monte Carlo was by Huggins et al. in 2022, which showed how a small quantum computer could unbias fermionic Monte Carlo simulations. In that scheme, a quantum processor was used to prepare a trial wavefunction and perform measurements that guided a projector QMC algorithm, greatly reducing the fixed-node error.
Xu and Li’s algorithm shares the same high-level goal (mitigating the sign problem by quantum means) but improves on scalability and efficiency. A limitation of the 2022 approach was the potentially large number of measurements needed from the quantum machine to average out noise. By employing Bayesian inference, Xu and Li manage to extract the bias-correcting information with far fewer samples, making their method more viable as systems scale up. They also propose a more flexible framework: rather than a one-size-fits-all algorithm, they outline two modes of operation, corresponding to different levels of quantum resource investment. This is a departure from the earlier “all-in” strategy and acknowledges that in some cases one might want to minimize quantum usage (to save time or cope with hardware limits) while still gaining an advantage. The numerical results in their paper showed notably improved accuracy compared to the fully classical algorithm across both modes, indicating that even a little quantum help can go a long way.
Other research groups have explored alternative hybrid QMC approaches. For instance, Yukun Zhang and colleagues proposed integrating classical shadows (a modern technique for compressing quantum state information) into the quantum-classical Monte Carlo loop. Their 2022 “Quantum Computing Quantum Monte Carlo” approach suggested using random quantum circuits and classical post-processing to estimate overlaps, though it came with some computational overhead of its own.
Each of these variants – whether it’s Xu and Li’s bias-correcting toolkit, or Zhang’s classical shadows – highlights different trade-offs in the quest to make quantum-augmented Monte Carlo practical. The consensus is that no single approach has “won” yet, and ongoing research is comparing their advantages. Xu and Li’s contribution of Bayesian optimization may alleviate some of that sampling cost concern by squeezing more information out of each quantum run.
It’s also instructive to contrast these Monte Carlo hybrids with the more well-known variational hybrid algorithms like the Variational Quantum Eigensolver (VQE). VQE attempts to solve the same electronic structure problems by running a parameterized quantum circuit and variationally adjusting it to minimize energy. While powerful, VQE faces challenges with many parameters and deep circuits, often requiring error mitigation and suffering from potential barren plateaus. The quantum-classical Monte Carlo approach, on the other hand, does not variationally optimize a quantum state – it uses quantum calculations in a more targeted way, to augment a stochastic projection of the ground state. This has a few implications: (1) The quantum circuits can be relatively shallow and fixed, since we are not performing iterative circuit optimization; (2) any remaining bias comes from identifiable sources (like the trial state choice or finite sampling) rather than an opaque variational ansatz; (3) the algorithm naturally tolerates certain noise, because random sampling inherently involves averaging (in fact, the 2022 Google experiment observed that their hybrid Monte Carlo was “naturally robust to noise” from the quantum hardware ).
On the flip side, hybrid QMC still requires a steady supply of random numbers and repeated quantum measurements, and its convergence properties can be complex to analyze (similar to other Monte Carlo methods). There is no free lunch: one trades a hard quantum state preparation problem for a hard sampling problem, but one that can now be managed with help from quantum subroutines. Researchers are actively testing these methods on various model systems to determine where the sweet spot lies. It may turn out that for certain strongly-correlated materials or molecules, the quantum-assisted Monte Carlo approach vastly outperforms any variational method or pure classical method – while for others, simpler methods suffice until larger quantum computers are available. As one AWS report pointed out, “it is an open research direction to find systems and trial states for which quantum computers are advantageous compared to a purely classical approach.” The good news is that multiple independent studies now confirm the core promise: a judicious combination of Monte Carlo and quantum computing can surpass the accuracy of classical simulation alone. The remaining task is to refine these techniques and map out exactly when and how to use them for maximum benefit.
Industry and Practical Impact
The development of hybrid quantum Monte Carlo algorithms comes at an opportune time, as industries are eager for methods to simulate complex quantum systems beyond the reach of today’s classical computers. Accurately calculating the properties of molecules and materials has direct applications in a variety of fields. Some key domains poised to benefit from these advances include:
Pharmaceuticals & Biotech
Drug discovery and design rely on understanding molecular interactions and reaction energetics. For example, predicting how a drug molecule binds to a protein target (and with what energy) is crucial for developing new medications. Ab initio methods like full Configuration Interaction are prohibitively expensive for such large, strongly-correlated biochemical systems. Quantum-assisted Monte Carlo could enable more accurate screening of drug candidates by capturing electronic correlations that classical algorithms miss. Even incremental improvements in computing binding energies or reaction pathways could shorten the R&D cycle for new pharmaceuticals. Quantum startups in the biotech space and research initiatives (like those at the Cleveland Clinic’s quantum program) are already exploring how hybrid quantum-classical tools might accelerate tasks such as protein folding and drug docking simulations.
Materials Science & Chemistry
The ability to simulate novel materials – from catalysts for sustainable chemistry to high-temperature superconductors – is a game changer for innovation. Many advanced materials involve strongly correlated electrons (think transition metal complexes, magnetic materials, or battery compounds) where classical methods struggle. Hybrid QMC algorithms can potentially tackle the electronic structure of these materials with higher fidelity, leading to better predictions of properties like conductivity, reactivity, and stability. For instance, in catalysis and chemical engineering, knowing reaction energy barriers and kinetic rates is essential; these quantities depend on accurately solving quantum many-body problems. The new algorithm by Xu and Li, or ones like it, might allow companies to computationally evaluate catalysts or materials before investing in lab prototypes, thus saving time and resources. In the long run, one can envision integrating such algorithms into materials design workflows – e.g. coupling a quantum-assisted Monte Carlo simulation engine with classical molecular dynamics or machine learning to discover optimal material compositions. Early adopters in this arena could be industries like petrochemicals, renewable energy (photovoltaic material design), and semiconductor design, where quantum effects are significant.
Quantum Chemistry and HPC Simulation
Even outside of specific industry verticals, the impact on the quantum chemistry community itself is profound. Chemists and physicists have a long tradition of leveraging high-performance computing (HPC) for simulations. Tools like classical QMC, density functional theory, and coupled-cluster methods are standard in national lab and pharmaceutical company computing arsenals. The arrival of a quantum-enhanced algorithm means these practitioners could, in the near future, access quantum-augmented HPC services. For example, cloud platforms might offer “quantum Monte Carlo” simulators where a quantum backend automatically boosts certain high-precision calculations. Amazon, for one, has already demonstrated running a hybrid quantum AFQMC workflow on their Braket cloud service as a proof-of-concept. In practical terms, a researcher could submit a job to the cloud that performs a difficult molecular energy calculation – behind the scenes, part of that job would dispatch circuits to a quantum processor to evaluate overlaps, then return the result to continue the classical Monte Carlo sampling. This convergence of quantum and classical HPC could usher in a new era of simulation-as-a-service, where end users may not even need deep quantum expertise to take advantage of the quantum speedup. Moreover, academic researchers in quantum chemistry will gain a powerful new “knob” to tune in their algorithms: by allocating some quantum computing time, they might solve problems previously deemed intractable with classical computing alone. The broader availability of such hybrid methods could spark further theoretical developments and cross-pollination between the quantum computing and computational chemistry communities.
Business Perspective
Major quantum computing players are already positioning themselves to leverage this kind of hybrid algorithm in their roadmaps. Google’s Quantum AI team has been at the forefront, as evidenced by their Nature 2022 experiment that used the Sycamore quantum processor to perform the largest quantum-assisted chemistry simulation to date. Google is likely to continue refining these algorithms, possibly integrating them into their open-source tools (like TensorFlow Quantum or OpenFermion) so that researchers can easily experiment with quantum-assisted Monte Carlo on available quantum processors.
IBM, meanwhile, has a strong focus on quantum advantage for practical problems and is developing an ecosystem (Qiskit Runtime, etc.) to support hybrid workflows. IBM has noted that combining quantum and classical resources is crucial for tackling scientific problems in materials, chemistry, and life sciences. It would not be surprising to see IBM include quantum Monte Carlo sample routines in future releases of their Qiskit software, allowing users to plug in an IBM quantum backend to bolster classical chemistry simulations. In fact, IBM’s partners like Algorithmiq and QunaSys (quantum software startups specializing in chemistry) are actively working on bringing cutting-edge algorithms to enterprise clients.
Startups and smaller companies are also poised to run with these ideas. Firms such as QunaSys (Japan) and Algorithmiq (Finland) are collaborating with industry and hardware providers to implement quantum chemistry solutions; they could adopt Xu and Li’s algorithm to enhance the accuracy of their simulation products for clients in chemicals and pharma.
Similarly, general quantum software startups like Zapata Computing and QC Ware may integrate hybrid Monte Carlo techniques into their platforms for customers who demand high-precision modeling (for instance, in finance for risk modeling or in aerospace for materials design, where Monte Carlo methods are common).
Even companies building quantum hardware might use this algorithm as a benchmark to demonstrate the utility of their machines on real-world problems – for example, showcasing that a 50-qubit superconducting system or an ion-trap device can improve a materials science simulation that classical computers alone cannot solve as accurately.
The practical impact of this development is also drawing interest from high-performance computing (HPC) centers and supercomputer labs. They see hybrid quantum algorithms as a way to extend the life of Moore’s Law by augmenting classical supercomputers with quantum co-processors. Rather than replacing supercomputers, quantum chips would work alongside CPUs/GPUs to tackle the hardest parts of simulations. Monte Carlo simulations are a staple workload in many HPC contexts (from nuclear physics to climate modeling – anywhere sampling of a complex space is needed). IBM’s recent work on a quantum-enhanced Markov Chain Monte Carlo for sampling complex probability distributions is a proof point that hybrid Monte Carlo ideas aren’t limited to quantum physics problems. In that 2023 IBM experiment, a quantum circuit was used to speed up a classical MCMC sampling of an Ising model, yielding convergence in fewer iterations than classical algorithms and demonstrating robustness to noise. This suggests that hybrid Monte Carlo techniques have broad applicability, potentially benefiting machine learning (e.g. sampling from Bayesian models), optimization, and other areas beyond chemistry. For the companies and institutions investing in quantum computing, these algorithms provide a concrete path to near-term value: they don’t require full-scale quantum computers to start delivering insights, and they can be tested and used incrementally. In an era where achieving a clear quantum advantage is the holy grail, having a hybrid algorithm that a) addresses important industry-relevant problems and b) can run on NISQ (noisy intermediate-scale quantum) devices is extremely attractive. It means quantum hardware in the next few years could be practically utilized in R&D pipelines at, say, a drug company or a materials design firm, instead of being confined to laboratories or abstract demonstrations.
Future Outlook
The emergence of quantum-assisted Monte Carlo algorithms like the Xu–Li method is part of a larger trend toward hybrid quantum-classical computing, which many believe is the most viable route to quantum advantage in the near term. Rather than waiting for fully error-corrected, million-qubit quantum computers (which are likely years away), researchers are finding ways to harness the unique strengths of today’s small quantum processors in tandem with classical computation. This symbiotic approach plays to the strengths of each side: the classical computer manages large-scale bookkeeping and sampling, while the quantum computer tackles the classically intractable pieces of the problem (like handling the exponential complexity of quantum states, albeit in small subsystems). The success of the hybrid Monte Carlo strategy could be a blueprint for other hybrid algorithms. We may soon see similar quantum boosts being applied to molecular dynamics simulations, optimization problems (quantum-assisted simulated annealing or genetic algorithms), and even AI/ML workflows (imagine a quantum-assisted Bayesian sampler for machine learning models).
Importantly, the techniques developed here – such as using Bayesian inference to minimize quantum measurements, or leveraging inherent symmetries for error resilience – can be transferred to other algorithms. They demonstrate clever ways to make the most out of imperfect quantum hardware. Xu and Li showed, for example, that if the physical system being simulated has certain symmetries, their quantum-assisted Monte Carlo becomes naturally error-resistant, since symmetric observables can cancel out some noise. Ideas like this will likely be adopted in future hybrid proposals to ensure that noise doesn’t wash out the quantum advantage. And as hardware improves (more qubits, less noise), the quantum portion of these algorithms can take on larger roles, further extending the reach of simulations. There is a clear roadmap: start with a small quantum aid that gives a measurable improvement (as has now been done), then gradually increase the quantum contribution as devices scale, with the ultimate aim of surpassing what classical computing alone can ever do. Each step of that journey provides value – we don’t have to wait for the endgame to reap benefits.
The path to practical quantum advantage will likely be paved with hybrid algorithms accomplishing specific tasks that classical methods struggle with. Quantum-assisted Monte Carlo is a strong candidate to be one of the first such tasks where a stable quantum advantage is realized in practice. The authors of the 2023 study explicitly suggest that their approach is a promising way to demonstrate quantum advantage on NISQ devices in the near future. Achieving this would be a watershed moment: it would mean that for a particular class of important problems (like computing a complex molecule’s ground state energy), a combined quantum-classical computation is unequivocally better than any known classical computation. That’s a pragmatic definition of quantum advantage and could energize investment and development in the field once confirmed. It’s worth noting that this does not mean quantum computers are outright replacing classical ones; rather, it’s about integration – much like GPUs accelerated AI by working alongside CPUs. In the coming years, we can expect to see more experiments and benchmarks of hybrid algorithms on increasingly powerful quantum testbeds. Each iteration will teach us more about the dos and don’ts of dividing work between quantum and classical resources.
If hybrid Monte Carlo techniques continue to advance, the implications extend to how we design quantum computers as well. We might optimize future hardware specifically for tasks like these – for example, ensuring fast qubit reset and readout to facilitate rapid repeated measurements (since these algorithms call the quantum routine many times), or tailoring qubit connectivity to efficiently prepare the kinds of trial states needed for chemistry problems. On the software side, quantum programming frameworks will likely offer higher-level modules for hybrid algorithms. We may soon have a Qiskit or Cirq module where a user can input a molecular Hamiltonian and automatically perform a quantum-assisted QMC simulation, without having to script the interplay of classical and quantum steps from scratch.
The broader impact on computational science cannot be overstated. Problems that were once thought to require an eventual fault-tolerant quantum computer might be solvable sooner via these hybrid tricks. It’s a shift from an all-or-nothing mindset to a continuum of quantum assistance. As one Nature commentary put it, the question has become “Meanwhile, how can we best exploit our powerful classical computers to advance our understanding of complex quantum systems?” – and the answer is increasingly: by augmenting them with quantum modules wherever they provide an edge. This philosophy will guide research in the near term.
In conclusion, Xu and Li’s 2023 quantum-classical Monte Carlo algorithm is a shining example of the tangible progress being made toward useful quantum computing. It tackles a real and challenging problem (the fermion sign problem) with ingenuity, blending new and existing techniques to achieve a result unattainable by classical means alone. The work stands on the shoulders of prior studies and pushes the envelope further by improving scalability and reducing quantum resource demands. Its significance is not just in the numbers and graphs of bias reduction, but in heralding a new computational paradigm where quantum and classical processors work in tandem to solve what neither could do as effectively on its own. As hybrid algorithms like this mature, we move closer to the era of practical quantum advantage – one focused application at a time. The coming years will reveal whether this approach can indeed demonstrate a definitive advantage in simulations of complex molecules and materials. If it does, it will mark the beginning of a new chapter in computational science, one where chemists, physicists, and engineers routinely enlist quantum computers as partners in discovery. The journey is just starting, but with each hybrid algorithm developed, the destination – revolutionary insights into the quantum world – comes into clearer view.